Some takeways after MEMCON 2024
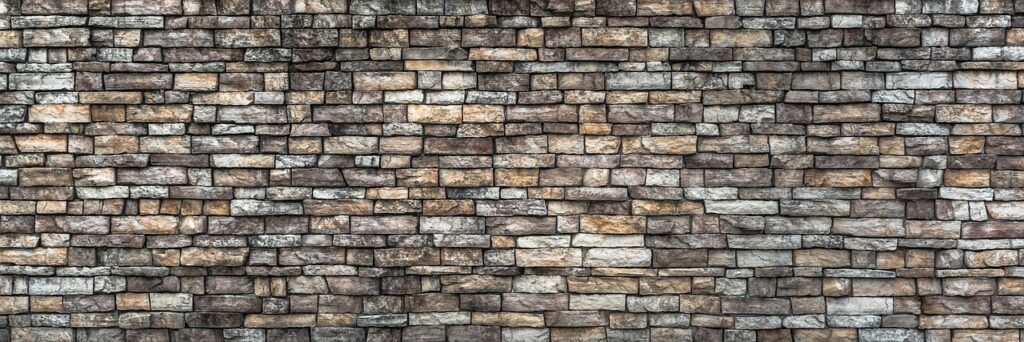
Image by jeonsango on Pixabay
During an interview by Brian Calvert for a March 2024 piece in Vox, climate lead and AI researcher at Hugging Face Sasha Luccioni drew a stark comparison: “From my own research, what I’ve found is that switching from a non-generative, good old-fashioned quote-unquote AI approach to a generative one can use 30 to 40 times more energy for the exact same task.”
Calvert points out that gen AI involving large language model ( LLM) training demands thousands of iterations. Additionally, much of the data in today’s training sets is more or less duplicated. If that data were fully contextualized and thereby deduplicated, such as via a semantically consistent knowledge graph, for example, far smaller training sets and training time would be sufficient.
(For more information on such a hybrid, neurosymbolic AI approach can help, see “How hybrid AI can help LLMs become more trustworthy,” https://www.datasciencecentral.com/how-hybrid-ai-can-help-llms-become-more-trustworthy/.)
So-called “foundational” model use demands truly foundational improvements that by definition will be slower to emerge. For now, LLM users and infrastructure suppliers are having to use costly methods just to keep pace. Why? LLM demand and model size is growing so quickly that capacity and bandwidth are both at a premium, and both of those are hard to come by.
Regardless of the costs, inefficiencies and inadequacies of LLMs, strong market demand continues. Hyperscale data centers continue to upgrade their facilities as rapidly as possible, and the roadmap anticipates more of the same for the next few years.
Even though developments in smaller language models are compelling, the bigger is better model size trend continues. During his keynote at Kisaco Research’s MEMCON 2024 event in Mountain View, CA in March 2024, Ziad Kahn, GM, Cloud AI and advanced Systems at Microsoft, noted that LLM size grew 750x over a two-year period ending in 2023, compared with memory bandwidth growth of just 1.6x and interconnect bandwidth of 1.4x over the same period.
The era of trillion-feature LLMs and GPU superchip big iron
What the model size growth factor implies is LLMs introduced in 2023 that are over a trillion features each.
Interestingly, the MEMCON event, now in its second year, had many high-performance computing (HPC) speakers who’ve often been focused for years on massive scientific workloads at big labs like the US Federally Funded Research and Development Centers (FFRDCs) such as Argonne National Laboratory, Lawrence Berkeley National Laboratory, and Los Alamos National Laboratory. I’m not used to seeing HPC speakers at events with mainstream attendees. Apparently that’s the available cadre that will point the way forward for now?
FFRDC funding reached $26.5 billion in 2022, according to the National Science Foundation’s National Center for Science and Engineering Statistics. Some of the scientific data from these FFRDC’s is actually now being used to train the new trillion-feature LLMs.
What’s being built to handle the training of these giant language models? Racks like Nvidia’s liquid-cooled GB200 NVL 72, which includes 72 Blackwell GPUs, 36 Grace Hopper CPUs, and an overall total of 208 billion transistors, which are interconnected with the help of fifth-generation, bi-directional NVLink. Nvidia launched the new rack system in February 2024. CEO Jensen Huang called the NVL 72 “one big GPU”.
This version of LLM big iron, as massive and intimidating as it looks, actually draws quite a bit less power than the preceding generation. While a 1.8T LLM in 2023 might have required 8,000 GPUs drawing 15 megawatts, today a comparable LLM can do the job with 2,000 Blackwell GPUs drawing four megawatts. Each rack includes nearly two miles of cabling, according to Sean Hollister, writing for The Verge in March.
My impression is that much of the innovation in this rack stuffed full of processor + memory superchips involves considerable packaging and interconnect design innovation, including space, special materials and extra cabling where they’re essential to address thermal and signal leakage concerns. More fundamental semiconductor memory technology improvements are going to take more than a few years to kick in. Why? A number of thorny issues have to be addressed at the same time, requiring design considerations that haven’t really been worked out yet.
Current realities and future dreams
Simone Bertolazzi, Principal Analyst, Memory at chip industry market research firm Yole Group, moderated an illuminating panel session near the end of MEMCON 2024. To introduce the session and provide some context, Bertolazzi highlighted the near-term promise of high-bandwidth memory (HBM), an established technology that provides higher bandwidth and lower power consumption than other technologies available to hyperscalers.
Bertolzazzi expected HBM DRAM in unit terms to grow at 151 percent year over year, with revenue growing at 162 percent through 2025. DRAM in general as of 2023 made up 54 percent of the memory market in revenue terms, or $52.1 billion, according to Yole Group. HBM has accounted for about half of total memory revenue. Total memory revenue could reach nearly $150 billion in 2024.
One of the main points panelist Ramin Farjadrad, Co-Founder & CEO at chiplet architecture innovator Eliyan made was that processing speed has increased 30,000x over the last 20 years, but that DRAM bandwidth and interconnect bandwidth have only increased 30x each during that same time period. This is the manifestation of what many at the conference called a memory or I/O wall, a lack of memory performance scaling just when these 1T learning models demand it.
This is not to mention that there are a number of long-hyped memory improvements that are sitting on the sidelines because the improvements are only proven in narrowly defined workload scenarios.
The ideal situation is that different kinds of memory could be incorporated into a single heterogeneous, multi-purpose memory fabric, making it possible to match different capabilities to different needs on demand. That’s the dream.
Not surprisingly, the reality seems to be that memory used in hyperscale data center applications will still be an established tech hodgepodge for awhile. Mike Ignatowski, Senior Fellow at AMD, did seem hopeful about getting past the 2.5D bottleneck and into 3D packaging, as well as photonic interconnects and co-packaged optics. He pointed out that HBM got started in 2013 as a collaboration between AMD and SK Hynix.
The mentioned alternative to HBM, Compute Express Link (CXL), does offer the abstraction layer essential to a truly heterogeneous memory fabric, but it’s early days yet, and it seems the performance CXL offers doesn’t yet compare.
DRAM leader Samsung, with nearly 46 percent of the market in the last quarter of 2023, according to The Korea Economic Daily, is apparently planning to increase HBM wafer starts by 6x by next year. Doesn’t seem likely that they’ll be closing the demand gap any time soon.