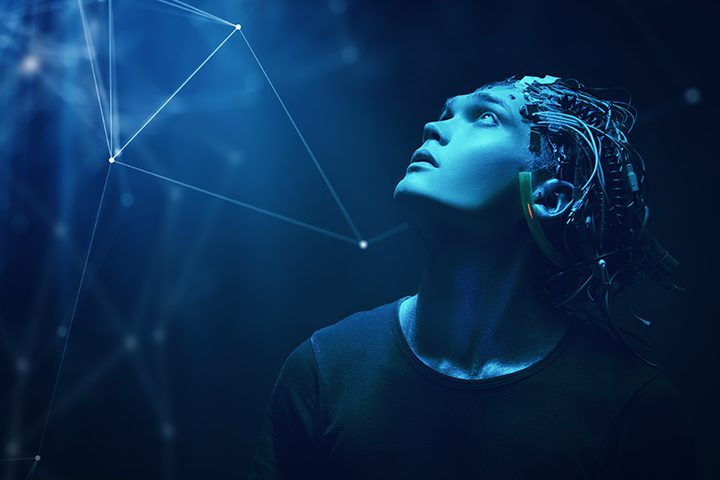
Data Points
- Hybrid AI is already overcoming the limitations of single technique approaches
- Symbolic AI understands actual knowledge, not just data
- Hybrid solutions open the “black box” of AI, helping to democratize the technology across the workforce
Symbolic AI and machine learning/deep learning all have their own set of strengths that, when used together in a hybrid fashion, make for a powerful approach that uniquely supports a number of key enterprise applications going forward. But before we get to that, let’s take a look at what each brings to the table.
Symbolic AI
Symbolic AI is designed to use actual knowledge to give it a greater understanding of real-world ideas and concepts. It’s similar to how humans (aka, biological intelligence) use specific, domain-oriented, symbolic, and semantic knowledge to interpret our surroundings. Symbolic AI links its algorithmic results to explicit representations of the knowledge it is exposed to. The most significant contribution of symbolic AI is in the area of natural language processing (NLP) and natural language understanding (NLU).
Machine Learning/ Deep Learning
Machine learning (ML) is the most common form of AI in the enterprise development pipeline at the moment. Since it has the ability to process disparate data sets autonomously and change its own behavior in response, it essentially “learns” the patterns of its environment and responds accordingly. This makes it a flexible and valuable tool for automated and autonomous compute environments.
Deep learning (DL) is machine learning based on neural networks. On average, DL can return better results but with a computational/energy cost that can be many orders of magnitude higher than simpler ML techniques.
Tapping the Value of Unstructured Language
AI has the potential to dramatically lessen two of the chief burdens that stand in the way of greater productivity and profitability. There’s the need to tap the hidden value of unstructured data efficiently and effectively. And there’s the need to implement greater levels of automation, not only over the rote, repetitive functions that make up the vast majority of structured data-driven workflows but also over information-intensive (or unstructured data-driven) processes.
At the same time, organizations will have to introduce new technology approaches in ways that are non-disruptive and fully transparent, so that any changes it brings can be easily understood and the resulting benefits are clear and immediate.
Pure machine learning approaches have a difficult time meeting all these requirements. By nature, they are a black box scenario: their inner working – basically their decision-making processes – remain hidden and unexplainable. For instance, when ML alone is used for natural language processing, any changes to input data can result in model drift, forcing you to train and test the data once again.
Symbolic and machine learning approaches aren’t mutually exclusive methodologies: by adopting a hybrid approach featuring all of these, the enterprise can achieve the best of all worlds. This includes efficient, effective support for core processes and applications, full transparency into why a given AI model behaves as it does and what can be done to correct it if its performance starts to waver.
At most enterprises, in fact, the largest trove of information is unstructured data in the form of language. From emails to voice recordings, legal contracts to regulations, the spoken and written word are how business gets done. Traditional compute platforms are great at processing structured data, such as client lists, financial records, and performance metrics, but only recently has AI made it possible to tackle unstructured content in a cost-effective way.
The Power of Symbols
Symbolic reasoning and semantic understanding can produce more accurate results across the entire AI ecosystem, while also reducing the time and expense of training new models, first by improving the quality of input data and then streamlining the cumbersome and resource-intensive infrastructure needed to support these massive volumes.
Operationally speaking, a hybrid environment provides the means to push AI past simple automating of rote, repetitive tasks to take on the higher-level jobs that require both knowledge and expertise but are still not at the strategic level where human operators are needed.
A prime example is annotation, the process of labeling information so it can be used by machines. This is a difficult and tedious job that nevertheless requires lengthy and costly training. But by leveraging the proper knowledge repositories and graphs within a symbolic learning environment, this training can be simplified to the point that it can be spread across the entire knowledge workforce.
Trust Matters
As mentioned above, another key problem with current AI deployments is trust. ML/DL suffers from the black box problem in that its outputs are not transparent or explainable.
This problem typically manifests itself in the realm of bias creeping into the AI training model. Stories abound of AI spewing out racist, misogynistic, and otherwise hurtful results because its input data has been skewed in this direction. Without the ability to peer directly into these feeds and see how the algorithms are responding to them, the entire system must be taken off-line and broken down, then retrained and re-introduced to production. Bias can also hamper AI in other ways, such as falsely predicting purchasing trends in key market segments or failing to account for cultural factors in college admissions.
Explainable AI
By introducing symbolic AI, the black box is opened so that users can understand why the machine is acting in a specific way and what can be done to change it if the results are undesirable. In addition, this visibility enables operators to continually monitor their own processes so they can be simplified and optimized.
AI is a powerful tool that can do wonders for enterprise data operations, but it is still in its infancy. The limitations of standard, single-mode AI iterations are already becoming clear to forward-leaning organizations. What is needed is a way for the technology to be more adaptive and more capable of delving into the depths of stored data, while at the same time becoming easier to use and less costly.
Hybrid AI – based on symbolic AI that understands actual knowledge rather than simply learning patterns – is the best way for enterprises to tap the value of all the data they’ve been collecting over the years.
Marco Varone
Marco Varone is the founder and CTO of expert.ai (formerly Expert System) and is one of the leading experts on semantic technology and natural language processing. He created the core technology of the company (formerly Cogito platform), and has worked in the field of artificial intelligence, semantic technology, natural language understanding and natural language processing in every advanced context: search engines, text analytics, NL interfaces, Q & A systems, automatic categorization, and many others. He has led the company throughout its consistent growth and has helped expert.ai to achieve major successes in various business sectors. He coordinates all activities of Research and Development and in his spare time, he writes about these issues on the company blog and his own blog, Semanticamente.