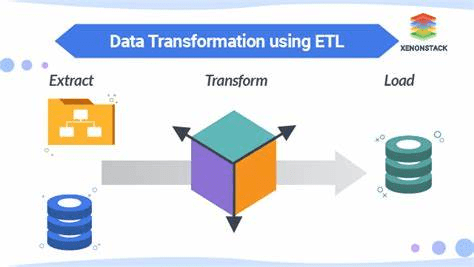
In today’s digital era, big data has emerged as a pivotal asset for organizations across various industries. The vast volumes of data generated every moment offer unparalleled insights into customer behaviors, market trends, and operational efficiencies. However, the true value of this data lies not just in its quantity but in the ability to process and analyze it effectively. This is where Extract, Transform, Load (ETL) processes become indispensable.
ETL is the backbone of data engineering, serving as a critical pipeline for transforming raw data into meaningful information. The extraction phase involves retrieving data from multiple sources, which could range from traditional databases to real-time streaming platforms.
In the transformation step, we cleanse, normalize, and reformat this diverse data, ensuring it adheres to a consistent structure and format, crucial for accurate analysis. Finally, the loading phase involves transferring this processed data into a data warehouse or analytical tool for further exploration and decision-making. This systematic approach not only streamlines data management but also enhances the reliability and usability of the information, turning big data into an invaluable resource for business intelligence and strategic planning.
Understanding Big Data and its business implications
Big data, characterized by its immense volume, varied formats, rapid generation, and the need for veracity, has become a cornerstone in contemporary data engineering. Volume refers to the massive quantities of data generated every second, from terabytes to petabytes, posing significant storage and processing challenges. Variety encompasses the diverse types of data, ranging from structured data in databases to unstructured data like emails, videos, and social media interactions. This diversity demands sophisticated parsing and integration techniques to ensure cohesive data analysis.
Velocity highlights the speed at which data is created and needs to be processed, often in real-time, to maintain its relevance. This necessitates robust and agile processing systems capable of handling streaming data and quick turnarounds. Lastly, veracity underscores the importance of accuracy and reliability in data. In the realm of big data, ensuring the quality and authenticity of information is paramount, as even minor inaccuracies can lead to significant misinterpretations in large-scale analyses.
In the business context, big data plays a critical role in driving decisions and spurring innovation. By analyzing vast datasets, organizations can uncover patterns and insights that were previously inaccessible, leading to more informed strategic decisions, personalized customer experiences, and efficient operations. However, managing big data comes with its own set of challenges, including ensuring data privacy, securing data against breaches, and maintaining data integrity. Overcoming these challenges is essential for businesses to truly leverage the potential of big data in driving growth and competitive advantage.
The role of ETL in Big Data management
The Extract, Transform, Load (ETL) process is fundamental in big data management, serving as the critical pathway for turning vast amounts of raw data into valuable insights. At its core, ETL encompasses three key stages:
Extract: This initial phase involves gathering data from various sources. In big data environments, these sources are diverse and voluminous, ranging from on-premises databases to cloud-based storage and real-time data streams. The challenge lies in efficiently extracting data without compromising its integrity, necessitating robust and versatile extraction mechanisms.
Transform: Once extracted, the data often exists in a raw, unstructured format that is not immediately suitable for analysis. The transformation stage is where this data is converted into a more usable format. This involves cleaning (removing inaccuracies or duplicates), normalizing (ensuring data is consistent and in standard formats) and enriching the data. For big data, transformation is a complex task due to the variety and volume, requiring advanced algorithms and processing power to handle the data effectively.
Load: The final stage involves transferring the processed data into a data warehouse or analytical tool for further analysis and reporting. In big data scenarios, this often means dealing with large volumes of data being loaded into systems that support high-level analytics and real-time querying.
The significance of ETL in big data management cannot be overstated. It enables efficient handling of large datasets, ensuring data quality and consistency, which are crucial for accurate analysis. Moreover, ETL acts as a bridge between the raw, often unstructured data, and the actionable insights businesses need. By effectively extracting, transforming, and loading data, ETL processes unlock the value hidden within big data, facilitating informed decision-making and strategic business planning. This transformation from data to insights is not just a technical process but a business imperative in the data-driven world.
Deep dive into ETL processes for Big Data
In the realm of big data, the ETL process is tailored to handle the complexity and volume inherent in diverse data sources. During extraction, data engineers employ techniques like parallel processing and incremental loading to efficiently pull data from various sources, be it traditional databases, cloud storage, or real-time streams. These techniques are crucial in managing the high volume and velocity of big data, ensuring a seamless extraction process without system overload.
Transformation is arguably the most intricate phase in big data ETL. It involves cleaning the data by removing inconsistencies and errors, which is vital to maintain data quality. Normalization is another key aspect, where data from different sources is brought to a common format, facilitating unified analysis. Advanced strategies like data deduplication and complex transformations are often employed, using tools capable of handling big data’s scale and complexity.
When it comes to loading, efficiency is paramount, especially with large datasets. Common techniques like bulk loading involve loading large volumes of data in batches, significantly reducing the time and resources required. Partitioning data before loading it into a data warehouse or lake is another strategy that enhances query performance and data management.
The retail industry provides a real-world example. A large retailer might use ETL to integrate customer data from various sources – online transactions, in-store purchases, and customer feedback. In this scenario, ETL processes not only handle the sheer volume of data but also transform it into a structured format suitable for analysis. ETL in big data scenarios showcases its transformative power by using this transformed data for personalized marketing, inventory optimization, and enhancing customer experiences.
Optimizing ETL for business intelligence
Integrating ETL Processes with business intelligence
Integrating ETL processes with business intelligence (BI) tools is crucial for deriving actionable insights from big data. This integration allows for the seamless flow of cleansed and transformed data into BI platforms, enabling real-time analytics and reporting. ETL plays a pivotal role in ensuring that the data feeding into these tools is accurate, consistent, and formatted appropriately for complex analyses.
In the context of data warehousing and data lakes, ETL is essential for structuring and organizing data. Data warehouses tailor ETL processes to transform and load data into a format optimized for query performance and data integrity. For data lakes, especially those handling unstructured or semi-structured data, ETL is critical in tagging and cataloging data, making it searchable and usable for analytics purposes.
ETL’s contribution extends to predictive analytics and informed decision-making. By efficiently processing and preparing large datasets, ETL enables businesses to leverage advanced predictive models and machine learning algorithms. These models can analyze historical and real-time data, providing businesses with foresight into market trends, customer behavior, and operational efficiencies. Thus, optimized ETL processes are not just about data management; they are a cornerstone in a company’s strategic decision-making toolkit.
Best practices in ETL for maximizing business value
Data quality management in ETL processes
Effective data quality management in ETL involves implementing validation rules and consistency checks to ensure accuracy and reliability. Additionally, regularly auditing and cleansing data helps maintain its integrity throughout the ETL process. In terms of data security and compliance, it’s crucial to employ encryption and access controls during data extraction and loading. Furthermore, adhering to regulatory standards like GDPR and HIPAA is essential for compliance and maintaining consumer trust.
Continuous monitoring and optimization of ETL processes are vital. Additionally, this includes performance tuning of ETL jobs, regularly updating the data transformation logic to align with evolving business needs, and proactively identifying bottlenecks. Such practices not only improve efficiency but also ensure that the ETL process continually adds value to the business by providing high-quality, secure, and relevant data for decision-making.
Conclusion
ETL processes are indispensable in the realm of big data, serving as a critical conduit for transforming vast, unstructured datasets into actionable business insights. The efficiency and effectiveness of ETL directly influences a business’s ability to harness the full potential of big data, driving informed decision-making and strategic planning. In an era where data is a key competitive differentiator, investing in robust ETL tools and processes is not just advisable but essential. Businesses that prioritize and refine their ETL practices unlock the true value of their data, fostering growth and innovation in today’s data-driven marketplace.