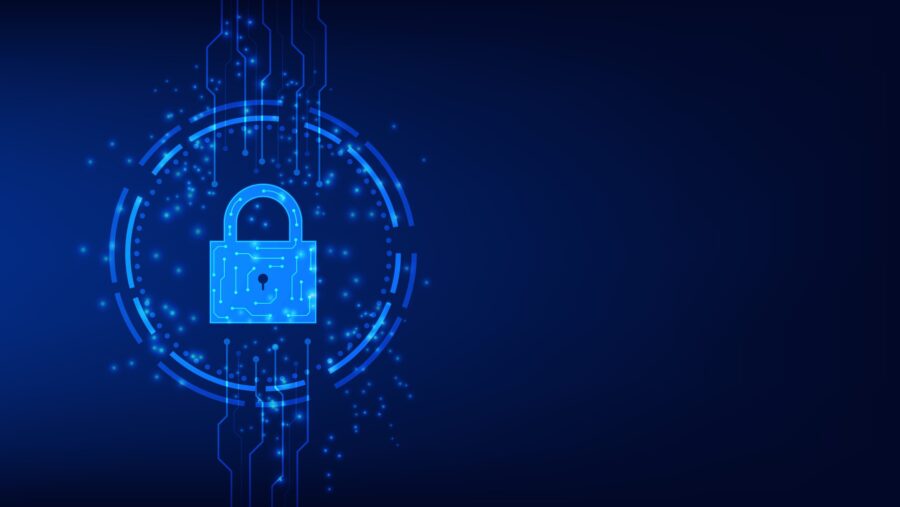
E-commerce has improved technology and convenience for consumers globally. Fraud is a problem in e-commerce. Merchants and platforms fight fraud to protect their businesses and customers. Anomaly detection is a powerful tool for identifying irregular patterns and potential fraud. This article explores how anomaly detection is used in fraud detection for e-commerce and discusses different approaches to implementing this technology.
E-commerce companies have a responsibility to protect their customers from fraudulent activity by securing their platforms. Cybercriminals exploit online vulnerabilities, posing a threat to businesses and customers.
What is e-commerce fraud?
E-commerce fraud is online deception involving the theft of information, unauthorized purchases, or false claims. The perpetrators focus their efforts on online retailers, payment systems, and customers, leading to financial losses, reputational harm, and an erosion of trust in the affected businesses.
Types of e-commerce fraud
Fraud in online commerce can happen in a variety of forms, depending on the strategy that the perpetrators of the fraud use to target businesses and customers.
The following are the most common varieties:
- Identity theft: Theft of identities occurs when criminals obtain personal information in order to make unauthorized online purchases and cause victims to suffer monetary loss.
- Credit card fraud: Credit card fraud occurs when con artists obtain cardholder information and then use it to conduct unauthorized transactions, resulting in monetary losses for both the cardholders and the businesses that they target.
- Chargeback fraud: Fraudulent chargebacks occur when customers question the legitimacy of their transactions, resulting in monetary harm to businesses.
- Phishing and social engineering: Cybercriminals use to trick customers to reveal sensitive information or commit fraud.
- Account takeover fraud: Fraud committed by taking over a victim’s account is known as account takeover fraud. Unauthorized users access a victim’s account and commit fraud or steal personal information.
- Refund fraud: People commit refund fraud by making false claims regarding the non-receipt of goods or the damage of goods in order to obtain refunds or replacements that are not deserved.
- Affiliate fraud: Fraud committed by affiliates occurs when dishonest affiliates attempt to manipulate commission structures by fabricating leads, sales, or clicks in order to receive unauthorized payouts.
- Counterfeit products: Sellers deceive customers with low-quality or fake items, harming brands.
- Dropshipping fraud: Deceptive dropshippers take payments but don’t send items or use stolen credit cards to buy and ship items to victims.
When it comes to digital transactions and online shopping, one of the most pressing concerns is e-commerce fraud. Businesses have a responsibility to their customers and themselves to protect themselves and their customers from the risk of fraud by making investments in security precautions, fraud detection systems, and employee training. Business tactics vary as much as e-commerce fraud types.
Understanding anomaly detection
Anomaly detection is about finding patterns or data points that deviate from the majority. E-commerce anomalies can include unusual transaction amounts, irregular purchasing behaviors, or suspicious account activities. Detecting anomalies is important as they could indicate fraud, like identity theft or credit card fraud.
Integration of anomaly detection in e-commerce fraud detection
Anomaly detection is important for fraud detection in e-commerce. Integration improves detection and response to suspicious activities. Anomaly detection is used in fraud detection for e-commerce.
Real-time monitoring and alerts
Anomaly detection monitors e-commerce transactions and user behavior in real time. Unusual patterns trigger alerts for investigation. This approach prevents fraud before it causes harm. Abnormality detection systems can immediately detect suspicious transactions, allowing businesses to prevent fraud and optimize digital marketing strategies.
Identification of unknown threats
Anomaly detection finds new patterns or fraud missed by rule-based systems. Anomaly detection models analyze data to learn and adapt to new fraudulent tactics, improving fraud detection.
Reduced false positives
Anomaly detection reduces false positives in fraud detection. By identifying anomalies, the system can differentiate between customer behavior and suspicious activities, minimizing disruptions to transactions.
Behavioral analysis
Anomaly detection analyzes user behavior by creating profiles and understanding normal patterns. Any deviation can be investigated. This approach is good for detecting account takeovers when the user’s behavior changes a lot.
E-commerce fraud prevention and detection
Businesses use methods for e-commerce fraud prevention, detection, and response to protect themselves and customers from threats. Some methods include:
1. Multi-factor authentication (MFA)
Multi-factor authentication, also known as 2FA or two-step verification, is a security process that requires users to provide two forms of identification to verify their identity when logging in or completing a sensitive transaction. Even if an intruder manages to steal one form of identification, multi-factor authentication (MFA) makes it much more difficult for them to access accounts or systems and thereby increases security.
The following are the three primary classifications that authentication factors can be placed in:
- Something you know: Includes passwords, PINs, or security questions for identity verification.
- Something you have: Smart cards, hardware tokens, and smartphones with authentication apps are examples.
- Quality you have: This includes unique human identifiers like fingerprints, facial recognition, and voice patterns.
Two factors are needed for MFA. Users enter a password and a one-time code from a mobile authenticator app. Attackers must compromise more authentication factors to gain unauthorized access, making it harder.
2. Machine learning and artificial intelligence
ML and AI are used to prevent and detect e-commerce fraud by analyzing data, identifying patterns, and adapting to trends. These technologies reduce manual review and rule-based systems by improving fraud detection accuracy and efficiency.
Here are some ways that ML and AI can be applied to e-commerce fraud prevention and detection:
- Anomaly detection: ML algorithms detect anomalies in transactional data. Flag anomalies for investigation.
- Scoring risks: Artificial intelligence (AI) systems assign risk scores to transactions based on factors such as transaction history, user behavior, geolocation, and device information. Transactions that involve a high level of risk may be subject to a manual review or additional authentication.
- Predictive analytics: Predictive analytics uses historical data to predict fraud and help businesses reduce risks.
- Behavior analysis: AI systems are able to analyze user behavior in order to identify fraudulent activity or attempts to take over an existing account.
- Monitoring in real time: Machine learning and artificial intelligence make real-time monitoring possible, allowing for immediate threat detection and response.
- Adaptive learning: Learning that is adaptable means that machine learning and artificial intelligence are able to adapt to new trends and strategies used by fraudsters. The effectiveness of fraud detection systems can be maintained through continuous learning.
- Reducing false positives: Reducing the number of false positives Traditional methods of fraud detection produce a high number of false positives, which leads to dissatisfied customers and missed sales opportunities. The accuracy of fraud detection is improved by ML and AI because they take into account more factors and can adjust to new information.
Online stores and transaction fraud
Analyzing large transaction data is challenging. Machine learning is used by fraud managers to investigate the reasons why certain transactions were not flagged as potentially fraudulent. Juniper Research predicts online retailers will lose $50.5B to fraud by 2024.
After running your ML system, you can learn which items are targeted by fraudsters, risky shipping information, and which card payments to block to avoid high chargeback rates.
Key approaches to anomaly detection
Effective anomaly detection requires robust approaches. Common fraud detection approaches for e-commerce:
Statistical methods
Statistical methods use math and stats to model behavior and find deviations. Methods include Z-score, Gaussian distribution, and clustering algorithms.
Machine learning
Machine learning techniques are powerful tools in anomaly detection. Algorithms learn from data to detect patterns and anomalies.
Unsupervised learning
Unsupervised learning algorithms detect anomalies without labeled data. They learn from normal data to identify outliers.
Hybrid approaches
Hybrid approaches combine multiple methods, often using statistics and machine learning. This method combines different approaches to improve anomaly detection accuracy.
Conclusion
In the ever-evolving landscape of e-commerce worth trillions, and the dynamic world of digital marketing, staying one step ahead of fraudsters is a constant challenge. Anomaly detection is crucial for e-commerce fraud detection. Its adaptability and real-time insights make it essential for fraud detection. E-commerce platforms can improve fraud prevention and safety by using anomaly detection and key approaches.
AI fraud detection is useful for e-commerce businesses to prevent fraud. AI algorithms analyze data to detect fraud, benefiting businesses by reducing losses, improving efficiency, and enhancing customer trust. Implementing these systems can be challenging due to data quality issues, false positives, model bias, technical expertise, and adversarial attacks. Businesses can implement AI-based fraud detection and prevention systems by addressing challenges and partnering with experienced professionals.