The benefits of AI for healthcare have been extensively discussed in the recent years up to the point of the possibility to replace human physicians with AI in the future.
Both such discussions and the current AI-driven projects reveal that Artificial Intelligence can be used in healthcare in several ways:
- AI can learn features from a large volume of healthcare data, and then use the obtained insights to assist clinical practice in treatment design or risk assessment;
- AI system can extract useful information from a large patient population to assist making real-time inferences for health risk alert and health outcome prediction;
- AI can do repetitive jobs, such as analyzing tests, X-Rays, CT scans or data entry;
- AI systems can help to reduce diagnostic and therapeutic errors that are inevitable in the human clinical practice;
- AI can assist physicians by providing up-to-date medical information from journals, textbooks and clinical practices to inform proper patient care;
- AI can manage medical records and analyze both performance of an individual institution and the whole healthcare system;
- AI can help develop precision medicine and new drugs based on the faster processing of mutations and links to disease;
- AI can provide digital consultations and health monitoring services — to the extent of being “digital nurses” or “health bots”.
Despite the variety of applications of AI in the clinical studies and healthcare services, they fall into two major categories: analysis of structured data, including images, genes and biomarkers, and analysis of unstructured data, such as notes, medical journals or patients’ surveys to complement the structured data. The former approach is fueled by Machine Learning and Deep Learning Algorithms, while the latter rest on the specialized Natural Language Processing practices.
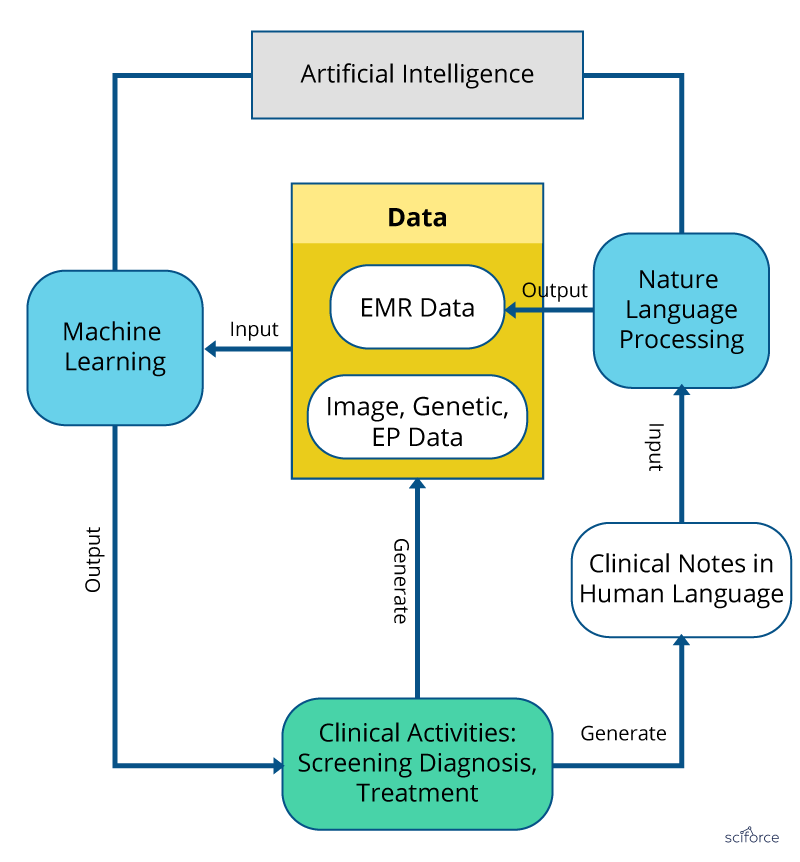
Figure 1. Machine Learning and Natural Language Processing in healthcare.
Machine Learning Algorithms
ML algorithms chiefly extract features from data, such as patients’ “traits” and medical outcomes of interest.
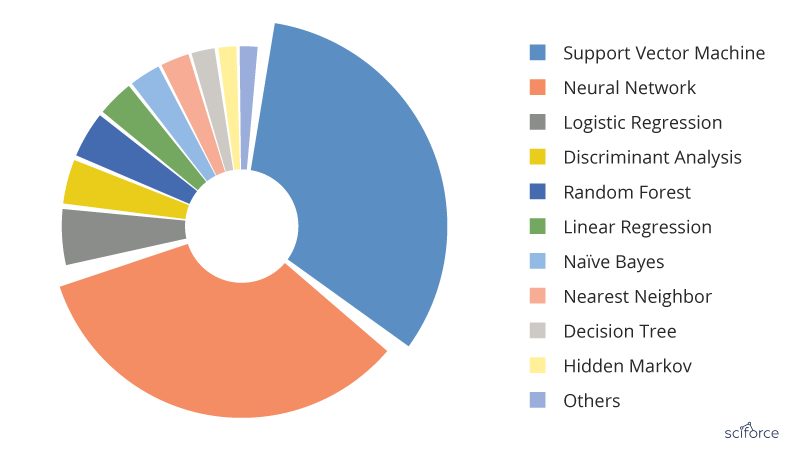
Figure 2. The most popular Machine Learning algorithms used in the medical literature. The data are generated through searching the Machine Learning algorithms within healthcare on PubMed
For a long time, AI in healthcare was dominated by the logistic regression, the most simple and common algorithm when it is necessary to classify things. It was easy to use, quick to finish and easy to interpret. However, in the past years the situation has changed and SVM and neural networks have taken the lead.
Support Vector Machine
Support Vector Machines (SVM) can be employed for classification and regression, but this algorithm is chiefly used in classification problems that require division of a dataset into two classes by a hyperplane. The goal is to choose a hyperplane with the greatest possible margin , or distance between the hyperplane and any point within the training set, so that new data can be classified correctly. Support vectors are data points that are closest to the hyperplane and that, if removed, would alter its position. In SVM, the determination of the model parameters is a convex optimization problem so the solution is always global optimum.
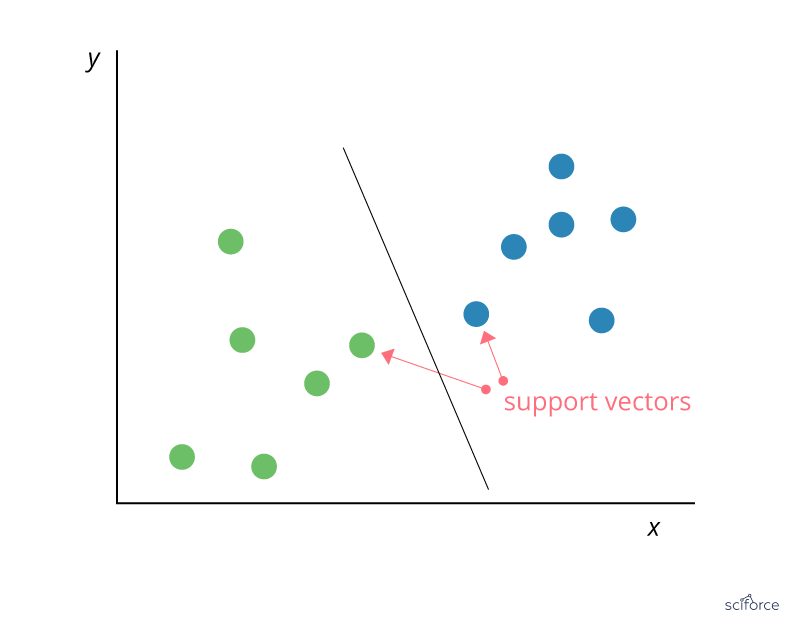
Figure 3. Support Vector Machine
SVMs are used extensively in clinical research, for example, to identify imaging biomarkers, to diagnose cancer or neurological diseases and in general for classification of data from imbalanced datasets or datasets with missing values.
Neural networks
In neural networks, the associations between the outcome and the input variables are depicted through hidden layer combinations of prespecified functionals. The goal is to estimate the weights through input and outcome data in such a way that the average error between the outcome and their predictions is minimized.
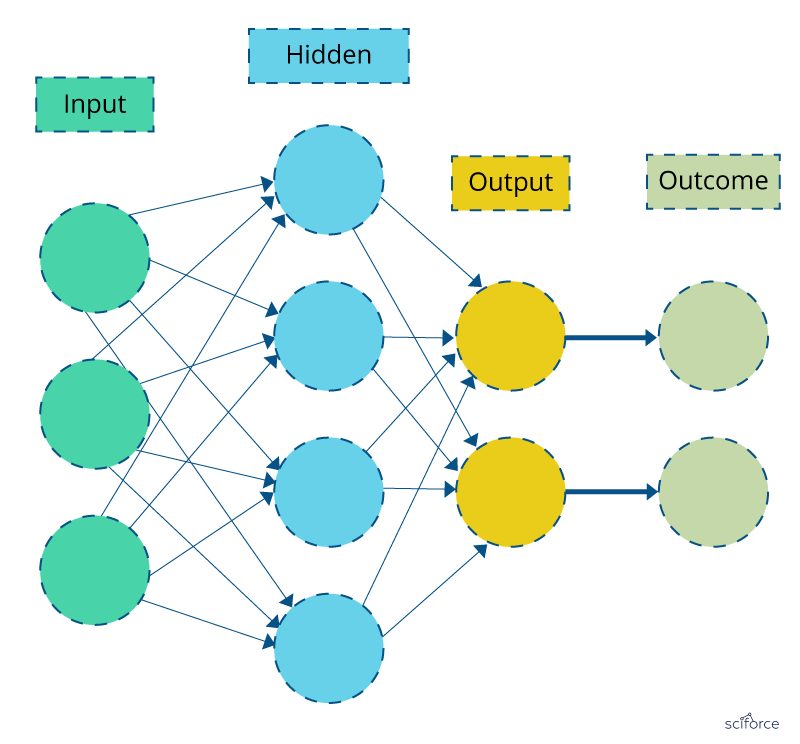
Figure 4. Neural Network
Neural networks are successfully applied to various areas of medicine, such as diagnostic systems, biochemical analysis, image analysis, and drug development, with the textbook example of breast cancer prediction from mammographic images.
Logistic Regression
Logistic Regression is one of the basic and still popular multivariable algorithms for modeling dichotomous outcomes. Logistic regression is used to obtain odds ratio when more than one explanatory variable is present. The procedure is similar to multiple linear regression, with the exception that the response variable is binomial. It shows the impact of each variable on the odds ratio of the observed event of interest. In contrast to linear regression, it avoids confounding effects by analyzing the association of all variables together.
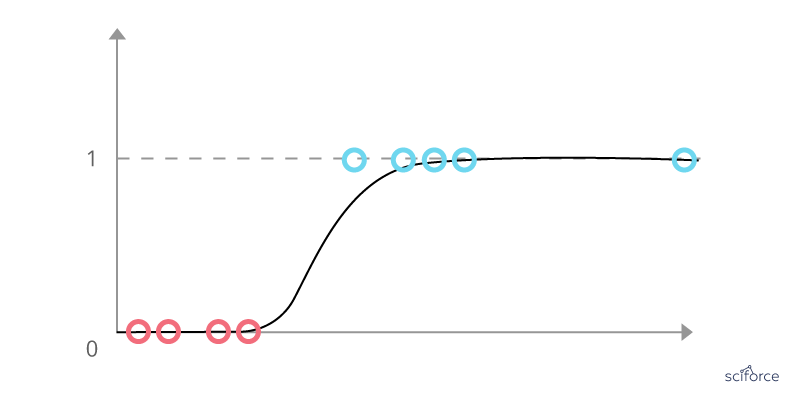
Figure 5. Logistic Regression
In healthcare, logistic regression is widely used to solve classification problems and to predict the probability of a certain event, which makes it a valuable tool for a disease risk assessment and improving medical decisions.
Natural Language Processing
In healthcare, a large proportion of clinical information is in the form of narrative text, such as physical examination, clinical laboratory reports, operative notes and discharge summaries, which are unstructured and incomprehensible for the computer program without special methods of text processing. Natural Language Processing addresses these issues as it identifies a series of disease-relevant keywords in the clinical notes based on the historical databases that after validation enter and enrich the structured data to support clinical decision making.
TF-IDF
Basic algorithm for extracting keywords, TF-IDF stands for term frequency-inverse document frequency. The TF-IDF weight is a statistical measure of a word importance to a document in a collection or corpus. The importance increases proportionally to the number of times a word appears in the document but is offset by the frequency of the word in the corpus.
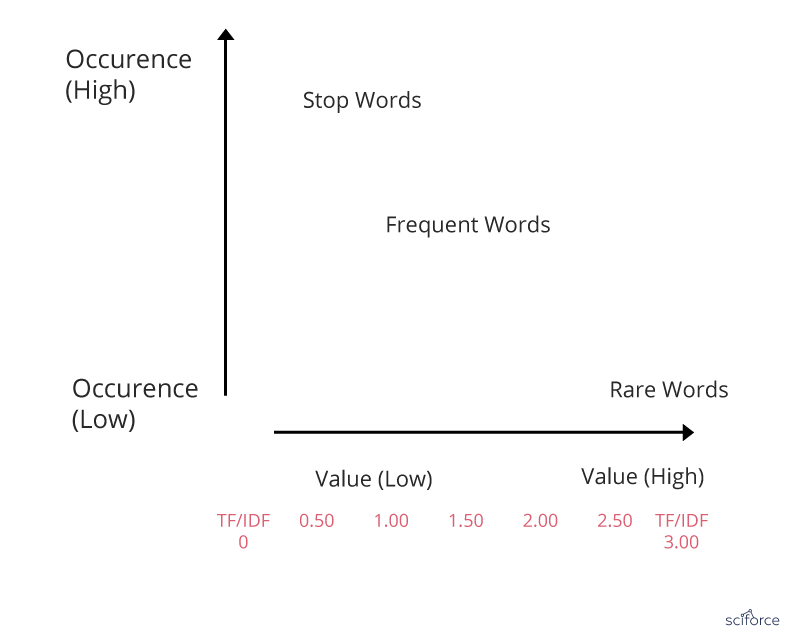
Figure 6. TF-IDF
In healthcare, TF-IDF is used in finding patients’ similarity in observational studies, as well as in discovering disease correlations from medical reports and finding sequential patterns in databases.
Naïve Bayes
Naïve Bayes classifier is a baseline method for text categorization, the problem of judging documents as belonging to one category or the other. Naive Bayes classifier assumes that the presence of a particular feature in a class is unrelated to the presence of any other feature. Even if these features are interdependent, all of these properties independently contribute to the probability of belonging to a certain category.
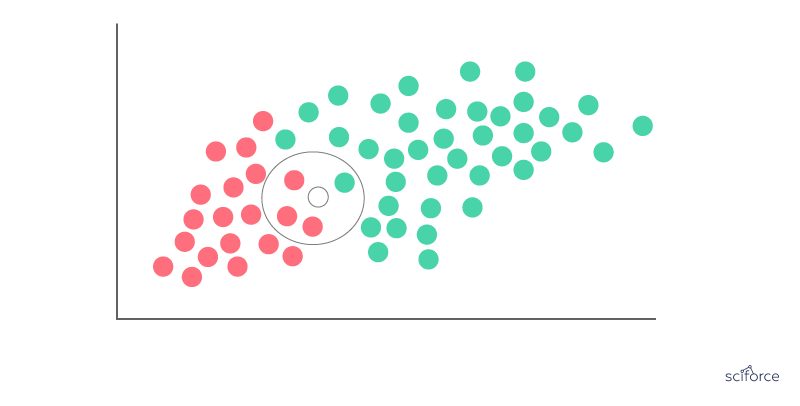
Figure 7. Naïve Bayes classifier
It remains one of the most effective and efficient classification algorithms and has been successfully applied to many medical problems, such as classification of medical reports and journal articles.
Word Vectors
Considered to be a breakthrough in NLP, word vectors, or word2vec, is a group of related models that are used to produce word embeddings. In their essence, word2vec models are shallow, two-layer neural networks that reconstruct linguistic contexts of words. Word2vec produces a multidimensional vector space out of a text, with each unique word having a corresponding vector. Word vectors are positioned in the vector space in a way that words that share contexts are located in close proximity to one another.
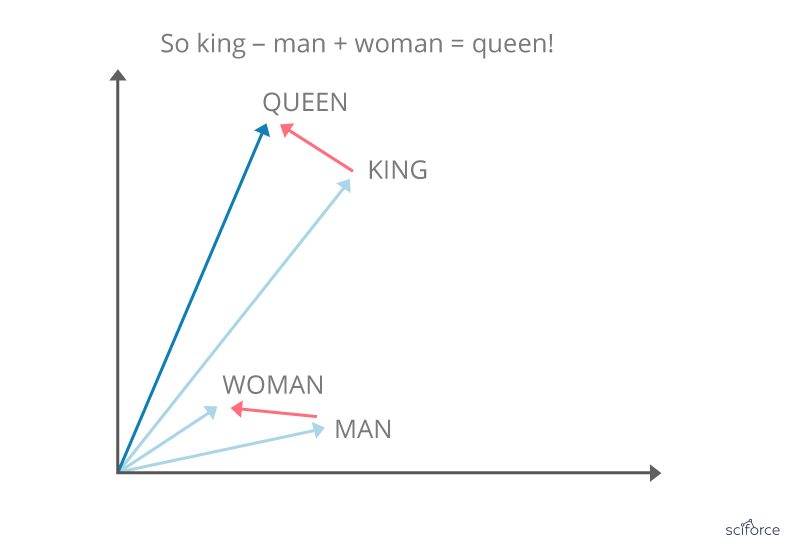
Figure 8. Word vectors
Word vectors are used for biomedical language processing, including similarity finding, medical terms standardization and discovering new aspects of diseases.
Deep Learning
Deep Learning is an extension of the classical neural network technique, being, to put it simply, as a neural network with many layers. Having more capacities compared to classical ML algorithms, Deep Learning can explore more complex non-linear patterns in the data. Being a pipeline of modules each of them are trainable, Deep Learning represents a scalable approach that, among others, can perform automatic feature extraction from raw data.
In the medical applications, Deep Learning algorithms successfully address both Machine Learning and Natural Language Processing tasks. The commonly used Deep Learning algorithms include convolution neural network (CNN), recurrent neural network, deep belief network and multilayer perception, with CNNs leading the race from 2016 on.
Convolutional Neural Network
The CNN was developed to handle high-dimensional data, or data with a large number of traits, such as images. Initially, as proposed by LeCun, the inputs for CNN were normalized pixel values on the images. Convolutional networks were inspired by biological processes in that the connectivity pattern between neurons resembles the organization of the animal visual cortex, with individual cortical neurons responding to stimuli only in a restricted region of the receptive field. However, the receptive fields of different neurons partially overlap such that they cover the entire visual field. The CNN then transfers the pixel values in the image by weighting in the convolution layers and sampling in the subsampling layers alternatively. The final output is a recursive function of the weighted input values.
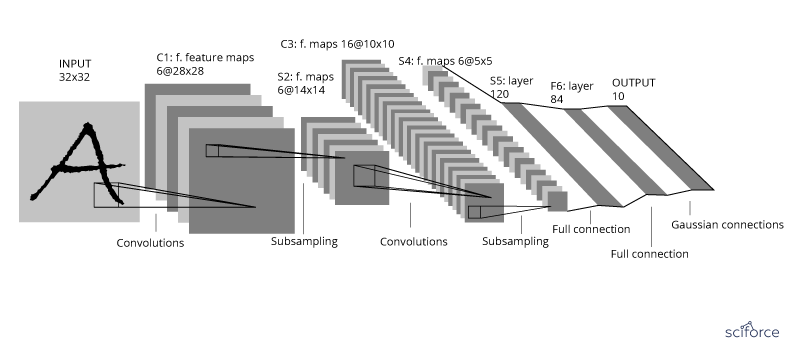
Figure 9. A convolutional neural network
Recently, the CNN has been successfully implemented in the medical area to assist disease diagnosis, such as skin cancer or cataracts.
Recurrent Neural Network
The second in popularity in healthcare, RNNs represent neural networks that make use of sequential information. RNNs are called recurrent because they perform the same task for every element of a sequence, and the output depends on the previous computations. RNNs have a “memory” which captures information about what has been calculated several steps back (more on this later).
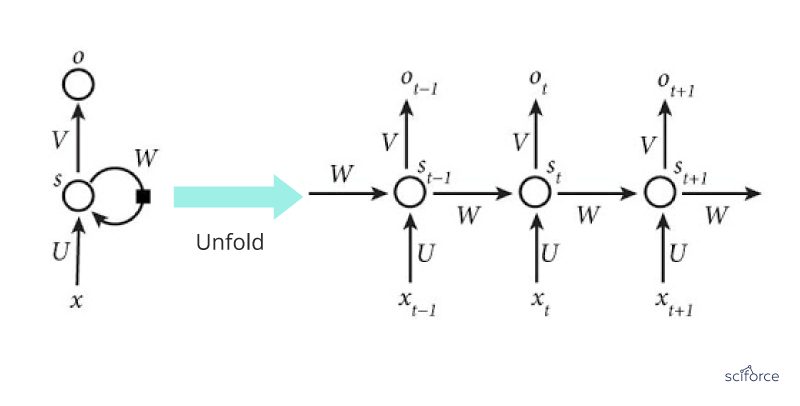
Figure 10. A recurrent neural network
Extremely popular in NLP, RNNs are also a powerful method of predicting clinical events.
Until recently, the AI applications in healthcare chiefly addressed a few disease types: cancer, nervous system disease and cardiovascular disease being the biggest ones. At present, advances in AI and NLP, and especially the development of Deep Learning algorithms have turned the healthcare industry to using AI methods in multiple spheres, from dataflow management to drug discovery.