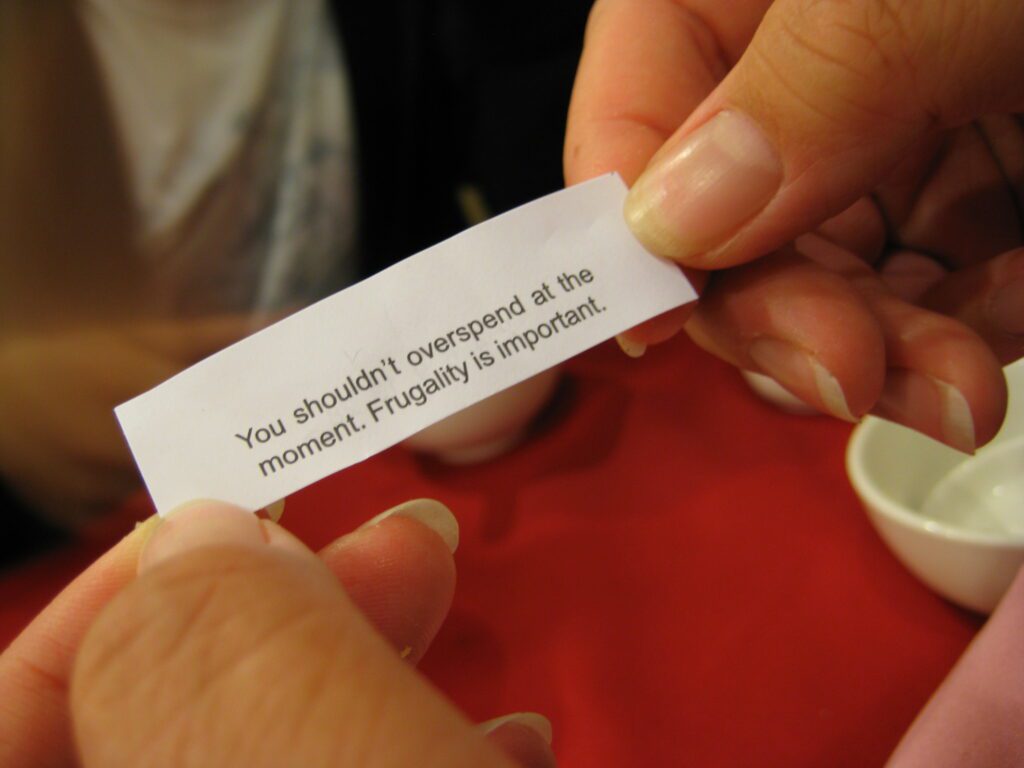
Photo by Ernesto Andrade on Flickr
Gartner’s April 2024 IT forecast estimated 8 percent growth from 2023 to 2024, forecasting $5.06 trillion in spending worldwide in 2024, rising to $8 trillion “well before the end of the decade.”
The amount the world spends on IT now surpasses what the entire population of people in low-income countries (4.5 billion people, 56 percent of the 8 billion people populating the globe) spend annually, according to The World Bank. Nearly half of low-income country spending is solely for food and beverages.
Implicit in all the trillions in IT spending is a built-in annual increase in a data integration tax due to unnecessary complexity. Dataware provider Cinchy estimates that half of enterprise IT budgets are now integration-related.
Why so much spending on integration? It all boils down to a lack of vision, planning, management acumen and sound technology choice. Specifically, it’s helpful to think about these ten different categories of IT management and related organizational management deficiencies:
- Passive or ineffectual leadership. Leadership often fails to grasp the power dynamics of business technology, how power shifts and how it’s used as technology becomes more pervasive and data mastery increases in importance. The result empowers new tech fiefdoms, each of which tries to assert its own dominance over the others. Each fiefdom has its own technology preference, and each department can have its own separate shadow IT presence. The result is misalignment and sprawl. Data utility suffers, and data complexity and fragmentation become more and more problematic.
- Incentive structure inadequacies. Any effort to create system interoperability requires method and motivation alignment at all organizational levels. Fiefdoms aren’t often incentivized to align and collaborate with one another. And even when they are, the incentivization isn’t actively managed. Even the best incentive structures need periodic realignment.
- Low investment in and commitment to scalable graph-based data architecture. Traditional integration and management technologies don’t scale, and yet companies continue to reinvest in these, overlooking the scalability and long-term cost effectiveness of knowledge graphs and web semantic standards to manage all kinds of enterprise data.
- Misconceived, undermined and duplicated data/content/knowledge management. Graph-based data management implies one set of methods regardless of the type of data being managed. Which means data, content and knowledge management can all be unified within a single department. Data quality and availability are key to success in both AI and traditional IT, but these factors get lost in the overall AI noise.
- Data ownership and access confusion. Every data source can have its own separate ownership and access rules. Often the default rule is no access, which leads to a need to negotiate access each time for each individual need in what constitutes a data adhocracy.
- Data model inconsistency. Every mobile or desktop app you use will most likely assert its own data model. The more data models to support, the higher the integration tax and the lower the likelihood data described by that data model will be fully integrated.
- Application sprawl. Duplicated application functionality fragments the data applications use to serve the same business functions.
- Naming inconsistencies. If you create or use a database with row and column headers, it’s likely those headers will differ slightly from database to database. Same with spreadsheets. Thus more potential duplication, integration hassle and lack of ultimate integration success.
- Data source inconsistency. Demand for new and improved input sources rises continually. Those third-party data sources differ from one another in slight, but troublesome ways, including implicit data model, format and quality variation. Data quality is most often inadequate to the task at hand.
- Dependency entanglements. Dependencies in general become more numerous and problematic in less predictable ways as systems, sources and methods proliferate.
How to pocket next year’s data integration tax increase
A good way to counter your organization’s data integration tax rate increases would be through its AI budget. Boards understand the need to take advantage of AI. Take the opportunity to educate them on the necessity of the right data foundation for AI. Start by crafting effective arguments for building a data foundation for AI, one use case at a time.
Find a nagging pain point that’s feasible to alleviate over the near term. Then propose the data foundation in miniature be designed to be reusable and scalable as the best knowledge graphs have been.
Your AI system can in this way run in parallel with your legacy IT environment. With the help of savvy technology choices, you’ll be able to ensure that 100 percent of the foundation you’re building using this parallel method will be legacy free.
Once the AI system becomes more broadly capable, you can use the same foundation for a transformed overall architecture based on findable, accessible, interoperable and reusable (FAIR) data principles.
A good way to learn best FAIR data practices and transformation strategy would be to study what companies such as AstraZeneca and Nokia have been doing in the area of biomedical research. (See https://www.datasciencecentral.com/digital-twins-interoperability-and-fair-model-driven-development/.)