Generative AI is probably the best product from humankind since fire and baked bread.
This analogy stands valid with respect to its comparison with fire because when fire was discovered, people feared it. They saw fire as apocalyptic, capable of causing destruction. It was only when we as humans worked on domesticating fire that evolution fell in place.
Artificial Intelligence (AI), specifically Generative AI stands at a similar juncture. At one side, we have tech enthusiasts, who are supercharged about the possibility of its implications across domains and industries. On the other hand, there are skeptics, who believe AI to be an agent of doom, causing ripples of fear around talent obsolescence by AI tools.
Now, this conversation gains more heat with the rise of a very niche application of Gen AI in the space of audio. After enabling artists and writers with the tools to think, visualize, and create better, Gen AI has carved a unique place in the field of acoustics.
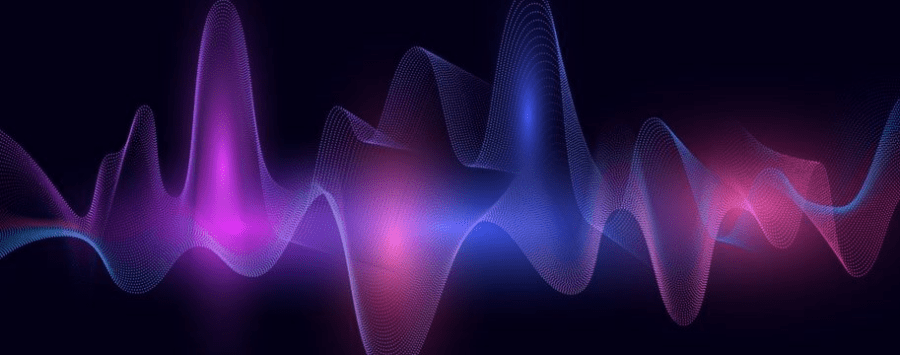
What is generative AI audio?
In the simplest of terms, this technology involves the generation of audio content with text as input or prompts. This audio content could range between anything from the generation of sound effects to an entire music album (more on its applications later).
The anatomy of generative AI audio
Converting texts as simple and vague as cinematic music for a horror short film comprising a string section is a daunting task. It’s a complex magic trick that involves layers of intricate technologies, techniques, and processes.
For the successful generation of audio that is as close to being passable involves techniques such as:
Tokenization – where data is broken down into discrete tokens that are individually analyzed and processed by Machine Learning (ML) algorithms at work. Each token is attributed to a distinct aspect of an audio signal such as pitch, scale, rhythm, and more.
Quantization – where continuous audio signals are represented as discrete values so the same generation technique deployed in LLMs (Large Language Models) can be utilized for audio generation.
Vectorization – that involves the transformation of audio signals into high-dimensional vector spaces to establish the relationship between diverse audio signals. ML models then identify and interpret patterns in signals to generate fresh audio or sound.
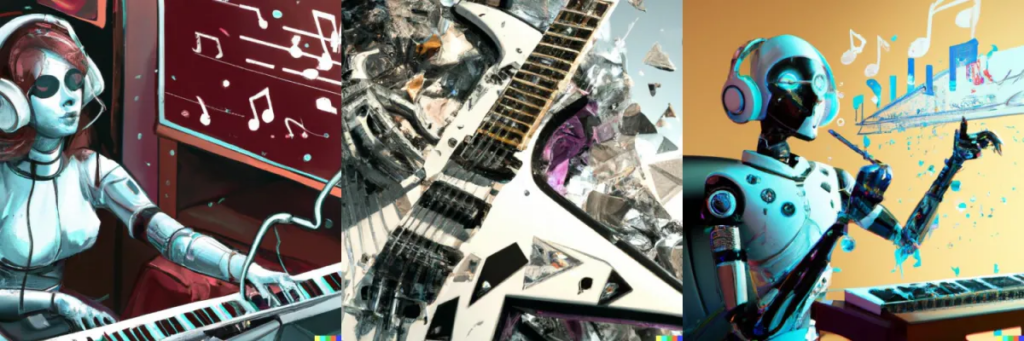
Applications of generative AI audio
While it’s easier to classify the applications of Gen AI audio into just music creation or deep fake audios, there are several unique and game-changing use cases of this technology that are highly relevant and required today.
Let’s explore some compelling ones.
Voiceovers and text to speech in EdTech
One of the most novel applications of Generative AI audio lies in the space of EdTech and infotainment, where artificial voice synthesizer technologies to generate voices of tutors and sound effects can be used to uplift storytelling in audiobooks, YouTube videos, course reading materials, eLearning modules, and more.
Sound designing
In the realm of movies and video games, where creativity knows no bounds, technicians are often compelled to invent fresh sounds. Imagine the sounds of Dune that made the auditory experience as immersive as possible; studios are required to push limits of familiarity and blend two or more everyday sounds into something unheard. Gen AI for audio can pull this off seamlessly through prompts that can be later tweaked by experts.
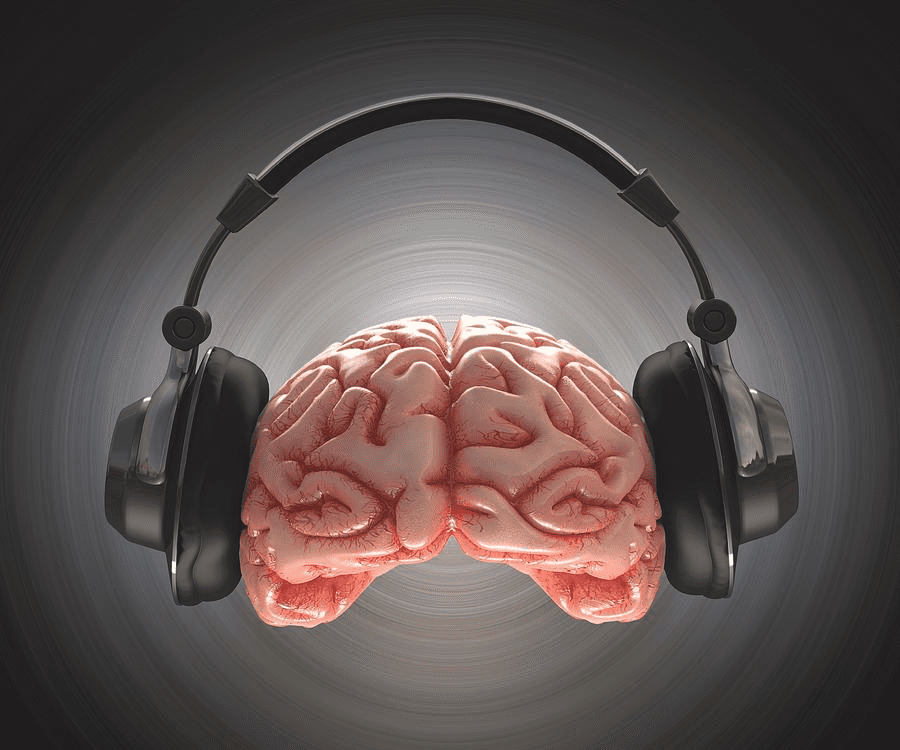
AI music creation
While this is a delicate topic that involves opinions and debates, it’s inevitable to acknowledge the power of Gen AI in creating music from scratch. From gaming to filmmaking, AI tools enable independent creators and artists with limited budget to elevate their content into something epic and cinematic.
Hyper-personalized chatbots
As brands and businesses race relentlessly to deliver the best of personalized experiences to customers, they can amplify this by a notch through Generative AI audio. Based on target audiences and demographics, chatbots can be trained to speak in specific ethnicities involving accents, dictions, and slangs people are familiar with for instant brand connection.
Real-time audio description for accessibility
Smartphones, devices, and even video streaming platforms have real-time audio description of content to simplify complexities for visually impaired people. Such autonomous generation of real-time audio content enables differently abled people to seamlessly achieve their everyday task, which otherwise might be constraining.
Challenges involved in generative AI audio development
Despite its potential being vast and niche, there are several bottlenecks stalling tech enthusiasts and businesses from leveraging the complete potential of this technology. Instead of taking a generic approach, let’s classify them into three unique aspects:
Technical and output-specific challenges
As fascinating as music generation from scratch sounds, the concept is still in its nascent stages. This means it is not devoid of technical concerns such as
- poor audio quality
- missing beats
- robotic delivery of accents and voices
- inconsistencies in real-time generation of audio
- audio results completely in a different tangent from entered prompt
- high latency and more.
Ethical constraints
This is a two-fold challenge that involves:
Deepfakes and misinformation, where synthesized audio morphed over an existing video or plain audio clips can be generated in the voices of targets to extract money or money’s worth or push specific agendas
Ownership and copyright that debates the perpetual question of who owns the music or sound generated by AI? Moreover, is it ethical to train on online data for audio synthesize without fair compensation to original creators?
Sourcing training datasets
The previous challenge is an excellent segue into this topic as black hat techniques prevail in training Generative AI audio models. With that said, there is also a pressing demand for quality audio training datasets that have clean audio customized to distinct requirements.
Bias is another critical concern in training Generative AI audio models, where subconscious input of audio data that is stereotypical, inaccurate or offensive could ruin and rupture outputs generated.