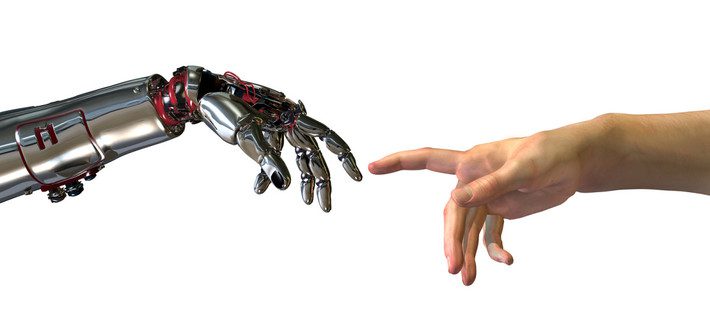
In a meeting with engineering leadership, I was told, “We’ll tack on the AI later.”
While doing ethnographic testing with a β customer, I was asked if the AI would just learn and then do everything perfectly.
During a discussion with engineering, it was unclear to me how we were going to train 400-dimensional sentence embeddings.
Those are a few examples of challenges a product manager might face when working with artificial intelligence.
As someone who has practiced the craft for decades (I founded a company that built an AI-related, algorithmic product and now run product management for applied AI for Xerox at PARC), I want to share some thoughts on what is distinctive about product management for AI. Product managing AI-based applications is still product management, but it requires some additional know-how, and maybe even some magic dust.
Product Management Baseline
While the role of product manager has been around since the ’30s, the specifics of the job function (the “mini-CEO”) have generally been vague. Nevertheless, the popularity of careers in product management have soared in recent years. Artificial intelligence has also been around since the ’50s, yet interest in AI has recently gone stratospheric. As such, now is a great time to examine product management in the area of AI.
In order to frame the discussion before jumping into the AI stuff, this definition from Marty Cagan’s book Inspired, which is both clear and encompassing, is the best I have heard:
The product manager has two key responsibilities: assessing product opportunities and defining the product to be built.
Assessing opportunities involves researching the market, talking with potential customers to identify their pain points, researching competitors and alternatives and, critically, understanding the capabilities of the organization that is going to develop the product. Defining products is then the process of describing what should be built, what the product should do and what features need to be delivered to meet, and then exceed, the needs of the users.
No matter the product, these two responsibilities will not change; the techniques and skills required to effectively deliver, however, will vary widely. With respect to AI-driven products, these skill requirements can be particularly acute. Punching your ticket to the Super Happy Magic Forest (my favorite children’s book by 100 miles, written by Matty Long) involves more than just slapping some AI into your PowerPoint. So let’s get to it.
Special Skills, Techniques, Attitudes and Responsibilities for AI Product Management
The product manager’s toolkit is comprised of methods to generate ideas, validate problems, define solutions, validate solutions, position products and otherwise put together an overall product vision and roadmap. While at a very high level all of this remains consistent no matter the product, when the application has a substantial AI component many of the methods need to be updated or revised and the product manager needs a certain set of fundamental building blocks to be able to effectively execute. A little product management magic dust for AI is required.
Based on personal experience, research and conversations with others, here are a few important things to consider:
- Understand the technology – Not understanding the capabilities and limitations of AI models will make it particularly challenging to assess opportunities and define AI-driven products. What is the model and from where is the data coming? Is there a sufficient quantity of data and is it ‘structured’ enough and labeled for training? How much cleaning and preprocessing is required? What are the inputs and outputs, and what algorithm is going to determine the relationship? What data will be used to further enhance the product as it ‘learns,’ and how will that work when data changes over time and by environment? If you don’t understand what these questions are about, my next post will be for you.
- Communicate the value proposition – As with every product the product manager will need to describe the problem being solved, but with AI you will also need to explain whether you are eliminating humans or enhancing them. How is your product or solution going to fit into existing workflows and are you able to talk credibly about the technology (see point above) to ‘sell’ the solution to potential β customers? For both customers and internal management stakeholders, you will have to manage the ‘hype’ around AI in order to control expectations without being pessimistic. How will you identify metrics to indicate the extent to which the product is delivering value? Will users need to understand and embrace ‘probabilistic’ results or functionality, making “Is this thing working?” a difficult question to answer? Will users blindly trust results and what dangers might that entail?
- Define the MVP – Defining the product, perhaps the trickiest element of any product manager’s job, gets more complicated when dealing with AI. Are there ways to build quick-‘n-dirty systems first, end-to-end, before expending considerable time and effort optimizing with more complex architectures? If so, what are those simple systems and how are you eventually going to switch to something more robust, assuming you get traction? Additionally, while the product might ‘learn’ over time, eventually generating superior results, do the initial outputs meet a minimum threshold of quality so that people will use the product, so that it can learn? Is the research-level AI sufficient for commercial release or is the real-world commercial implementation going to be a disappointment?
- Do not forget the non-AI stuff – All the cutting-edge AI stuff is thrilling, but even if you nail the AI-related elements, you cannot neglect the non-AI stuff that goes around it. A self-driving vehicle still needs beverage holders. This might not be as sexy, but poor functionality around your AI core could ruin a product if it doesn’t get the attention it deserves.
More to Come
This list is not comprehensive, and it’s obviously filled with lots of questions and, as of yet, few answers. Nonetheless it is hopefully a starting-point for thinking about challenges around product managing AI-related products. I intend on exploring each of these four main areas, and perhaps others, in future posts.
Thank you and suggestions are welcome
I hope you enjoyed my inaugural post on LinkedIn. There is much I want to learn, so please share your thoughts and insights in the comments. Suggestions for follow-up posts are also encouraged. I am looking forward to sharing ideas with the community and continuing to develop my understanding of this fast-moving profession.