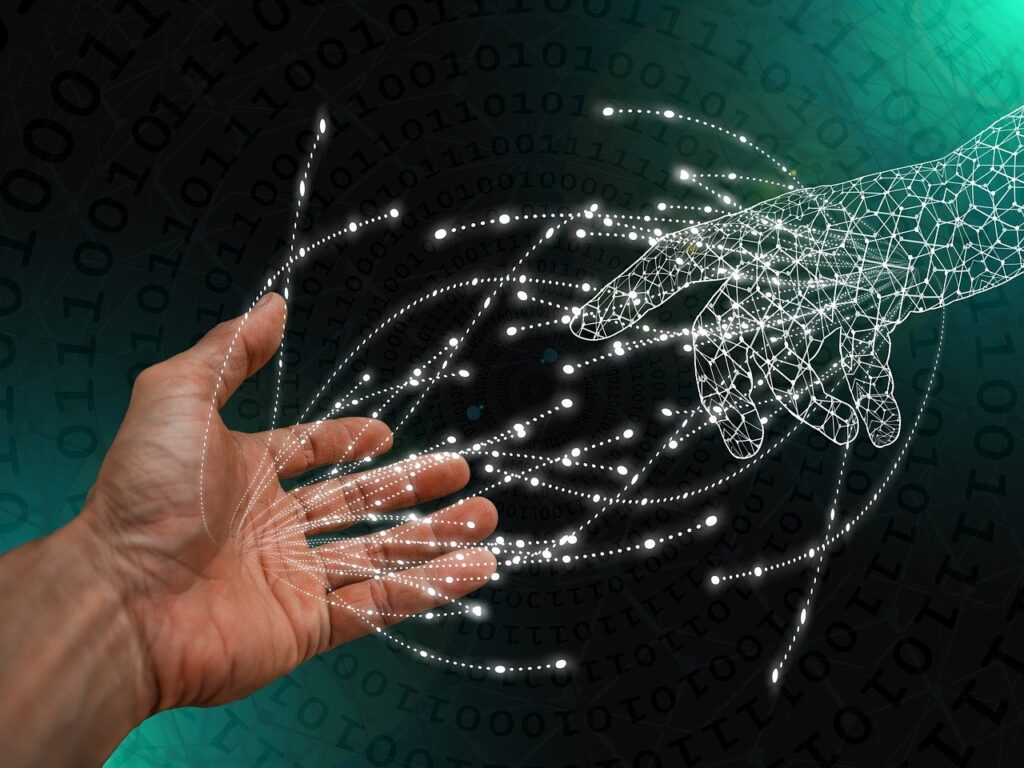
Image by Gerd Altmann from Pixabay
Every week, I watch emerging tech markets evolve and try to ponder what the changes mean in terms of real business impact on large enterprises and other kinds of businesses as well. Being a market watcher has been a habit of mine for almost 25 years now as a longtime forecaster, researcher and trends analyst.
It’s best as a market analyst to track how systems as a whole evolve, rather than just individual technologies. Doesn’t make sense to adopt the part without attention to its impact on the whole.
The capabilities of a given AI system in that sense, I’ve learned, hinge on sufficient amounts of comprehensive, relevant data that’s findable, accessible, interoperable and reusable (FAIR). If you don’t have the right kind of FAIR data to make the AI system work the way it needs to, you don’t have an advanced AI system, period.
Furthermore, companies who’ve contributed most to FAIR data initiatives have committed to standards and a knowledge graph approach.
Interestingly, Gartner in its 2023 Hype Cycle for Artificial Intelligence plotted knowledge graphs near the end of the Trough of Disillusionment, implying a level of maturity most newer AI-related technologies don’t have.
In its Impact Radar for 2024, Gartner also put knowledge graphs in the bullseye with generative AI, the two technologies Gartner decided promised the biggest impact potential this year. The implication I’m taking from these Impact Radar + Hype Cycle findings is that knowledge graphs should be a higher priority than generative AI. After all, generative AI has just entered the Trough of Disillusionment, and gen AI needs quality, contextualized data just as much as any other form of AI does.
Seven tenets of transformation in the AI era
If AI depends on data, what is digital transformation without data layer transformation?
For the past 15 years, enterprise data layer transformation has been a key focus of mine, particularly transformations that involve the use of semantic graph databases or knowledge graphs more generally. I’ve had that focus because data maturity writ large is a precondition to business intelligence, analytics and AI success.
As I’ll describe shortly, using a hands on knowledge graph approach can change an enterprise’s entire data management mentality. Committing to such an approach makes systems designed to harness the power of AI as a whole more scalable and manageable
These seven tenets of enterprise transformation in the AI era underscore both the situation we’re really in and what it will take to make substantive AI progress:
- Despite the hype since 2019, we still have only narrow artificial intelligence, and still only natural language processing, not understanding.
- To be able to make best use of the AI that exists demands that each enterprise build a modern knowledge foundation, i.e., data that’s logically connected, contextualized and extensible with the help of shared, interlinked, symbiotic graph data models.
- Organizations can’t be data mature if they’re not in data sharing mode whenever they can be, as well as pushing the data sharing envelope. Holding onto data doesn’t make sense if you’re not sharing with the people who need what that data can provide.
- Transformation doesn’t matter if you’re not providing enterprise-wide and even boundary-crossing transparency in the process.
- It doesn’t make sense to buy “AI” without having the ability first to feed the AI the best, high quality, relevant, disambiguated data you can.
- Most of the AI software providers out there, unfortunately, aren’t focused on helping you solve your data problems.
- Most enterprises have huge, unresolved data problems. Therefore, most enterprises won’t benefit all that much from AI.
Example case studies from enterprises committed to multi-year, AI-era transformation
Enterprises who’ve demonstrated years of commitment to knowledge graph-based data contextualization, management and sharing are among those organizations most worth emulating. Below are two compelling recent examples.
Aerial drone interdiction knowledge sharing at the US Department of Homeland Security (DHS). DHS at the Semantic Arts’ 2023 Data-Centric Architecture Forum shared details of its efforts to integrate heterogeneous, real-time intelligence sources at scale using a standards-based knowledge graph approach. DHS was focused on detecting smuggling drones crossing the US-Mexican and US-Canadian borders and quickly alerting agents to the landing sites so the smugglers could be apprehended.
Port-wide emissions monitoring using knowledge graph-based digital twins and agents. Portsmouth Ports now monitors and shares timely metrics on emissions updates to a broad range of industry users. Armed with this information, shipping companies, for example, can determine which vessels, when and where, are out of compliance with emissions control requirements. This level of temporal + spatial detail is essential to bring various transportation types and the ports themselves into compliance with UK regulations. (See the IOTICS Portsmouth Ports use case at https://www.datasciencecentral.com/preconditions-for-decoupled-and-decentralized-data-centric-systems for more information.)
More case studies like these can be found on my Data Science Central page at https://www.datasciencecentral.com/author/alanmorrison/ Let me know your thoughts. If these case studies help, or if there are other case studies along these same lines you’d like to point out, please let me know.
Wow, great one Alan. The seven tenets are spot on a very quotable (which we will be doing) Nicely done