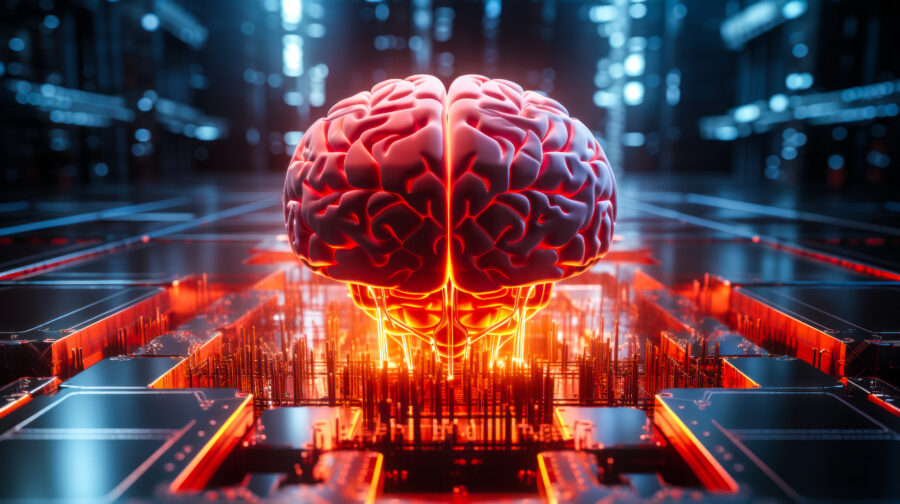
It is unlikely that AI safety for superintelligence and artificial general intelligence could be achieved directly without a track from current risks. Already, there are minute fractions of predicted existential threats of AGI that provide a map towards preparing for the unknowns, ahead.
AI is a dynamic non-living thing. Its dynamism applies to tasks, positive or otherwise, but it does not have a core aspect of what comes with the dynamism of organisms—the ability for some control, resulting in misuses like deepfakes and misinformation.
If it were possible to collect certain input and output vectors of large language models, it could become a near-term AI safety mechanism against present problems of deepfakes and misinformation.
Currently, what are the available technical options to fight deepfakes? Mostly guardrails, digital hashing, watermarks, authentication, encryption, identity verification, and others. While they hold potency in many aspects, they may not be thorough enough for the safety needs against several voice cloning or impersonation techniques, as well as deepfake images, videos, and misinformation reaches.
Though, without guardrails, these problems would have been much worse, guardrails appear to bear individual capability more than general capability, where safety may matter more.
How can deepfake audio be tracked at the source? How is it possible to collect this across AI tools that are indexed on search engines? How can this apply to deepfake videos and images, as well as misinformation?
A research area for AI safety, for now, could be web crawling and scraping of AI tools, for their embeddings. This could be possible by a different kind of robot.txt and data API. The research would explore how to do this, in intervals, for certain keywords, to monitor what is processed, to provide information, to sources where they might be used, to foil their impact on arrival.
In a recent report by Reuters, Exclusive: Multiple AI companies bypassing web standard to scrape publisher sites, licensing firm says, it is stated that “Multiple artificial intelligence companies are circumventing a common web standard used by publishers to block the scraping of their content for use in generative AI systems, content licensing startup TollBit has told publishers. TollBit said its analytics indicate “numerous” AI agents are bypassing the protocol, a standard tool used by publishers to indicate which parts of its site can be crawled. The robots.txt protocol was created in the mid-1990s as a way to avoid overloading websites with web crawlers. More recently, robots.txt has become a key tool publishers have used to block tech companies from ingesting their content free-of-charge for use in generative AI systems that can mimic human creativity and instantly summarize articles.”
Since AI models gather data on web scraping, it should be possible to allow some of their inputs and outputs to be scrapped as well, in a technical adventure that can be done within the province of the US AI Safety Institute and the UK’s.
These embeddings from several sources, around certain keywords or events timing, could become fresh sets of data to explore intent, using extensive dot products, predicting for major risks of AGI.
The compute requirement may be tapered by having the scraped embeddings in blocks, for reporting and tracking, aside from experimenting for future risks. This approach may also provide an extra option among ongoing efforts against hallucinations.
There is a new paper in Nature, Detecting hallucinations in large language models using semantic entropy, where the authors wrote, “Here we develop new methods grounded in statistics, proposing entropy-based uncertainty estimators for LLMs to detect a subset of hallucinations—confabulations—which are arbitrary and incorrect generations. Our method addresses the fact that one idea can be expressed in many ways by computing uncertainty at the level of meaning rather than specific sequences of words. Our method works across datasets and tasks without a priori knowledge of the task, requires no task-specific data and robustly generalizes to new tasks not seen before.”
What would it mean for AGI to be safe? A question is intent—good intent, as well as little to no goal direction, while it can be free enough to do good stuff. Efforts on these could begin now, with current misuses, which may prospect a general standard as well, where, long before AGI, AI outputs and inputs can be generally tracked, then monitored, in a way to gather use ranges, and place safety, against allowing open risks maturation.