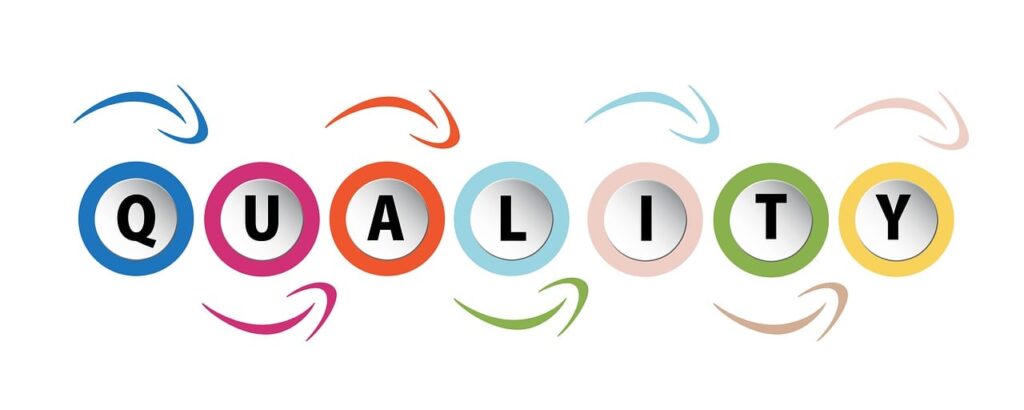
Image by Gerd Altmann from Pixabay
In March 2024, dbt Labs, a freemium platform and tools provider focused on helping organizations with their data transformation efforts, completed its State of Analytics Engineering survey of 456 data practitioners and leaders. Among the key data prep findings were these:
- 57 percent of respondents said poor data quality was their top concern, up from 44 percent in 2022.
- 57 percent said that they were or would soon be managing data for AI training.
- Almost 50 percent ranked low stakeholder data literacy as a major concern.
- 44 percent of respondents identified ambiguous data ownership as a top concern.
Unsurprisingly, the data practitioners surveyed said they spend 55 percent of their time maintaining or organizing data sets. And nearly 40 percent said integrating data from various sources was their biggest challenge.
The good news was that close to 40 percent of companies intend to maintain their investment in data quality, platforms and catalogs, with 10 to 37 percent planning to increase these investments over the coming year, depending on the investment category.
Of course, the big question is how to make the most impact with those investment dollars. If a given company is reporting a major issue with ambiguous data ownership, ownership squabbles will be consuming a big portion of the time that could be spent on activities that directly impact data quality.
Three root causes of data quality shortfalls in enterprises
1) Lack of an innovative data collection, management and reuse culture. Until generative AI and machine learning in general took the spotlight, leadership wasn’t placing a priority on data. The focus was on applications. Organizations have had to get to their data through applications that fragmented or trapped the data the applications generated. Many of these applications continue to be underused.
2) Perpetuation of costly legacy data architecture that doesn’t scale. Most companies don’t realize they spend more and more year over year on what dataware company Cinchy calls an “integration tax,” with 50 percent of their IT budgets or more spent on integration because of architecture complexity. Standards-based semantic graph architectures allow more ease of integration.
3) Failure to see the problem through an Integration tax lens. Overly complicated archtiectures continue to cause integration costs to spiral out of control. Until organizations learn to connect and contextualize the data layer with the help of a flexible, tiered, unitary data model for reusability at scale, they will keep having to face yearly integration tax increases. (For more information, see “The role of trusted data in building reliable, effective AI” https://www.techtarget.com/searchenterpriseai/tip/The-role-of-trusted-data-in-building-reliable-effective-AI.)
Push proactive, organic data management efforts upstream to boost data quality
The closer to the data source, the higher the impact potential on quality. Being proactive about first-hand collection and focusing your data quality efforts upstream (closer to the source) allow more control.
The further organizations are from the original context of the data collection and the mentality of the person doing the data collection, the harder it is to harness and then repurpose that data.
An example of effective upstream management processes: An oil field example
Oil industry processes aren’t just about pumping crude out of the ground and converting it to fuel or petrochemicals at centralized refineries.
Besides what happens at refineries, there are lots of upstream processes, both existing and emerging. Let’s take direct lithium extraction (DLE), which when done upstream at oil field sites is on the cusp of commercialization, as an example of an emerging, innovative process that has parallels with what could be done in the data quality/management space.
Active oil fields produce brine, which occurs naturally in geologic formations or as a result of water injection to force oil to wells where it can be pumped out.
DLE takes brine (which is dirty, salty water), decontaminates it and then extracts lithium from it.
Currently, DLE in operation at salt lakes is responsible for ten percent of lithium production for applications such as electric vehicle and mobile phone batteries.
Hard rock lithium mining, by contrast, is responsible for around 60 percent of production, according to the Sustainable Minerals Institute of the University of Queensland (UQ).
Volt Lithium in Calgary, Canada, to name one of a number of DLE startups, claims a 99 percent decontamination and 90 percent lithium extraction capability, according to UQ. Volt plans to start DLE operations at oil fields in Alberta, Canada in the third quarter of 2024. DLE’s emerging role in oil field brine processing is timely, given that research firm BMI has predicted a lithium shortage by 2025.
Be a data producer. Own your own data and data lifecycle processes
New, fit-for-purpose data sources means being able to see things from a producer’s point of view, as well as having marketable resources on hand to share, trade or monetize. Data product advocates anticipate that every data consumer will become a producer as well.
Final point: Data quality’s been a nagging issue in enterprises for decades, and it’s only gotten worse. It’s clear companies are stuck in a data management rut, still doing things in ways that don’t result in significant improvement.
I’ve written a lot over the past four years about how to do data management better with the help of standard, well-described graph data as an integration and sharing medium. Browse through some of my other posts if you’re looking for helpful ways to kickstart a data transformation effort.
Hi Alan
A proper case can be made if the mining companies allow for DS to be implemented at their sites.
I doubt if a firm like VL will be open to it.
Although any mining company like VL would be great opportunity for DS.