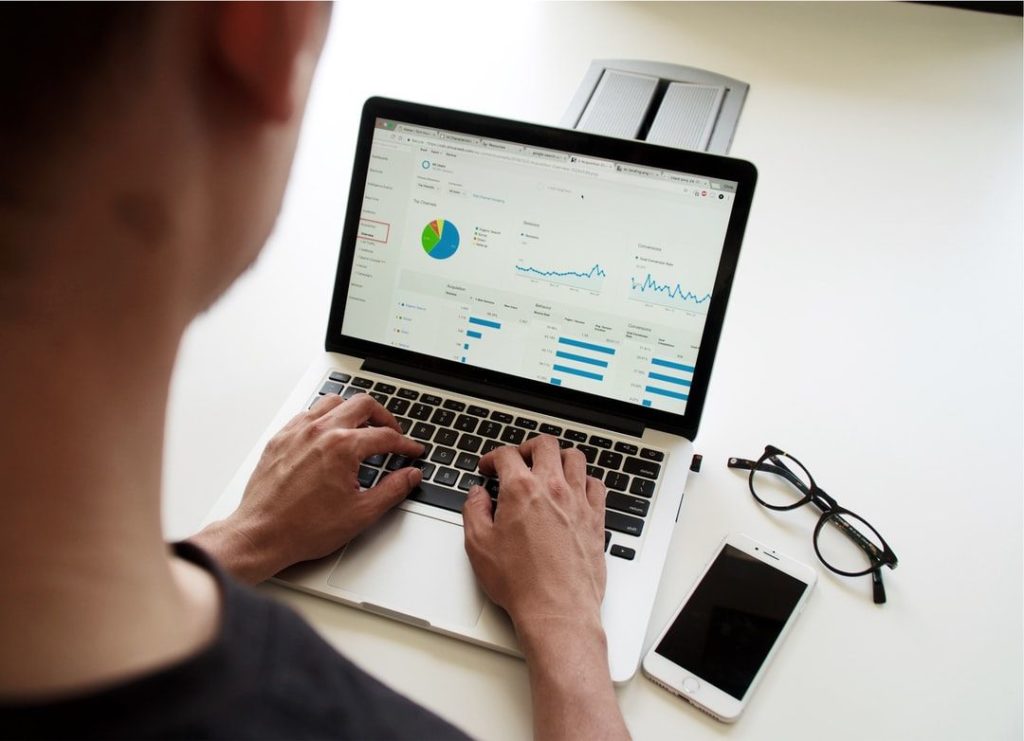
An average consumer uses various marketing channels while interacting with a brand. The numbers were calculated in Upland BlueVenn’s latest Digital Divide Report. They examined 500 marketers and 4000 consumers across the UK and US in 2021, and found that at least 20 marketing channels were used. When data comes from so many ends, the biggest challenge marketers face today is cleaning data and identifying which records belong to the same consumer.
Data has become a crucial asset for every process happening at an organization. It is extensively used to extract insights about who the consumer-base is, what their demographics are, their likes and dislikes, and so on. But due to poor data quality issues, not only marketers, but sales reps, product managers, and other important roles end up missing out on big opportunities since they cannot false data to build customer relationships.
In this blog, I want to talk about 360-customer view, customer data matching, and the best practices to follow to achieve a single source of truth. Let’s dive in.
What is 360 customer view?
The terms ‘Customer 360’ or ‘360 Customer View’ refer to a complete, 360-degree view of a customer’s data. Interactions that a customer has with your brand are recorded across datasets in your organization. From website visiting activity, to product purchases, reviews, and support tickets – all this information is usually scattered across data files, sources, or third-party applications. And when various departments want access to this customer data, they end up using different versions and formats.
What is customer data matching?
Customer data matching is a process of comparing potential duplicate records of the same customer, and identifying whether they belong to the same individual.
At times, you have uniquely identifying attributes in your customer dataset (such as Social Security Number, Unique Customer ID, etc.) that can help in matching records. But in the absence of such attributes, you can select a combination of non-unique attributes (such as Customer Name, Address, Phone, etc.), and calculate the likelihood of the records being similar.
Best practices to achieve 360 customer view
Here are some of the best practices that your teams can adopt to ensure better customer data quality.
- Define data quality for your customer dataset
It is very difficult to achieve something that is not well-defined. One of the most significant practices that people need to adopt is to define what the customer data should include. Usually, organizations rely on and accept whatever third-party applications are storing for them, instead of proactively creating their own customer data model that suits their needs.
You need to consider what type of information you want to store about your customers, as well as what they are willing to share. Once you have that, you can define the customer data model that notes down all attributes, what they mean, whether they are required or optional, and so on.
- Educate teams on handling customer data
Nowadays in the multidimensional business world, we have various departments operating at a company. And almost every functional group handle customer data at some point of their operations. This is why it is necessary to train and educate teams on how to handle customer data.
Introduce the concept of data literacy at your organization by designing and assigning courses to teams. Here you can explain what the customer dataset contains, what each data field means, what is the criteria for quality, what is the right and wrong way of manipulating customer data, and so on.
Not only will this keep the customer data clean, but also help them to assess how to use customer data and get maximum value out of it.
- Continuous customer data profiling
Data profiling assesses the current state of your data according to the defined data quality. This helps in identifying missing, incomplete, invalid, or inaccurate information. Scheduling regular data profile reports can help in catching data quality issues early on and fixing them before they migrate to all systems. You can also set custom workflows that alert important roles (such as data stewards) when data quality plummets below an acceptable threshold.
- Implement end-to-end data quality processes
Achieving a single customer view is not only about matching duplicates or filling in missing information. The results of data matching are most accurate when the data is subjected to a systematic process of data quality management.
A data quality management lifecycle can contain multiple processes, such as data profiling, data cleansing, data standardization, data transformation, data matching, data merging and purging, amongst others. Try to implement a systematic data pipeline that converts raw customer data into the useful, complete view of customers.
- Utilize tools and technology
Once you figure out the right processes that work for you in attaining a single source of truth for your customer data, the next step is to automate and optimize by using appropriate tools and technology. You want to ensure that the processes are repeatable and the quality of your customer data is being constantly monitored and fixed.
Some companies opt for complete data management systems with in-built data quality features. The choice of a solution depends on your business needs.
Conclusion
More than half of customer data is either lost or left unused due to poor management and data quality practices. A customer dataset that is clean, standardized, and deduplicated, is one of the biggest assets for any organization. This does not only facilitate day-to-day operations, but also becomes the foundation for improved customer experiences and reliable consumer insights.