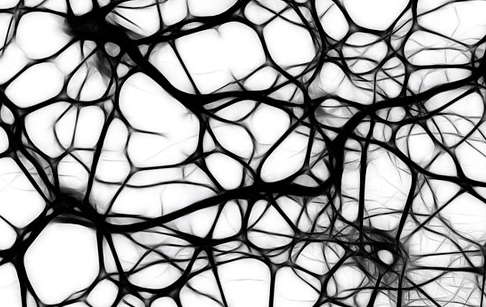
Artificial intelligence (AI) has reached peak hype. News outlets report that companies have replaced workers with IBM Watson and that algorithms are beating doctors at diagnoses. New AI startups pop up everyday, claiming to solve all your personal and business problems with machine learning.
Ordinary objects like juicers and Wi-Fi routers suddenly advertise themselves as “powered by AI.” Not only can smart standing desks remember your height settings, they can also order you lunch.
Much of the AI hubbub is generated by reporters who’ve never trained a neural network and by startups or those hoping to be acqui-hired for engineering talent despite not having solved any real business problems. No wonder there are so many misconceptions about what AI can and cannot do.
Neural networks were invented in the ’60s, but recent boosts in big data and computational power made them actually useful. A new discipline called “deep learning” has arisen that can apply complex neural network architectures to model patterns in data more accurately than ever before.
The results are undeniably impressive. Computers can now recognize objects in images and video and transcribe speech to text better than humans can. Google replaced Google Translate’s architecture with neural networks, and now machine translation is also closing in on human performance.
The practical applications are mind-blowing as well. Computers can predict crop yield better than the USDA and indeed diagnose cancer more accurately than elite physicians.
John Launchbury, a director at DARPA, describes three waves of artificial intelligence:
- Handcrafted knowledge, or expert systems like IBM’s Deep Blue or Watson
- Statistical learning, which includes machine learning and deep learning
- Contextual adaption, which involves constructing reliable, explanatory models for real-world phenomena using sparse data, like humans do
To read the full original article click here. For more deep learning related articles on DSC click here.
DSC Resources
- Services: Hire a Data Scientist | Search DSC | Classifieds | Find a Job
- Contributors: Post a Blog | Ask a Question
- Follow us: @DataScienceCtrl | @AnalyticBridge
Popular Articles