Thousands of companies today declare they plan to invest in big data, sometimes with legitimate reasons. They want to hire scientists, invest in infrastructure, revolutionize the field, and so on. This is why many people are looking into changing their careers and working on new skills meant to secure them a position as a data scientist. Since there aren’t many big data science faculties, it is safe to assume that most graduate students are in the same position.
How does one become a data scientist?
They need to either learn more than what the curriculum teaches them or get the necessary skills after graduating. So the natural question is how exactly to prepare. And to answer that, one needs to understand the basic things about the necessities of the companies hiring data scientists. You would think that any question is easy to answer since this domain is all the rage today. Surprisingly, that is not so.
Open problems
Exactly how big is Big Data? What is the average set size that an average hiring company has on the table? What is the set size distribution? What is the usual signal to noise ratio encountered in the data sets used by these companies? These are all simple questions that should have numerical answers. They are all important points because the data size determines the minimal skill set for a future hire.
There is not enough quantitative knowledge on how big is Big Data.
Having quantitative estimations regarding big data feels natural since everyone is looking so much into it. A targeted systematic search looking at dozens of scholarly papers, books, statistical reports, research news and media articles revealed that no one knows the answers to these questions. Answering these questions would require a study obtaining the data from the hiring companies and the data scientists currently working for them.
The state of the field
The most on-topic report available looking at the data set size, “The State of Big Data Infrastructure”, quotes an unknown number of respondents (p.7, Fig. 6). The same report quotes 90% of companies as reaping the benefits of big data and most respondents having data sets over 1PB.
The State of Big Data Infrastructure, p.7, April 2015, based on a number of “X” respondents. Set size distribution as % of the total
This comes into conflict with 2 other reports stating that most companies fail at using big data and that only 29% of companies are actually using big data to make predictions. It also comes into conflict with the data from the KDnuggets surveys quoting the largest data set as being less than 1TB for 70% of the respondents. As we all know, the differences in findings can be put to the exact nature of the questions, which highlights another problematic aspect of statistic research.
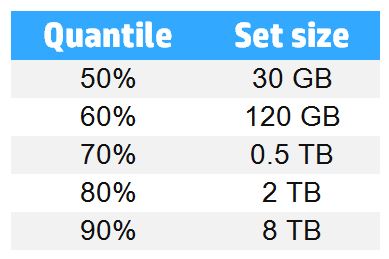
KDnuggets, numbers based on smoothing, 2015
While the discrepancy in terms of declared beneficial outcomes can be put to the nature of the questions, the 3 order of magnitude difference between the stated data set sizes remains questionable.
Current studies only seem to care about how much companies are planning to invest in data research, define big data, categorize it, or talk about the 3V – which are a nice but strictly qualitative/descriptive approach. Other studies are looking at data sets possibly just because they are already available, even if the data is specific to a business model that is not easy to replicate or even useful to most companies. Researchers are looking at the industry giants but there is no word about the set size in the average company. The KDnuggets surveys are investigating the largest data sets handled by a specific company, without asking about the typical set size. It is indeed wise to be able to handle data surges however the daily load is much more useful to know for those trying to prepare for such a job.
Some papers even go as far as to forecast the future of the field, but the analysis is obviously qualitative, having no strong quantitative background to justify the predictions. These predictions may well be interesting, but if they are only useful for a narrow category of people or companies, it can be said that they miss their target. It is completely understandable that the field spreads over many domains which makes it difficult to run a quantitative analysis, however the most notable of these domains, such as DNA sequencing or the Sloan Digital Sky Survey, are not representative for big data in any other way than by being wondrous exceptions.
The Internet of Things size estimations are based on a single prediction from 2006.
Another point which should be mentioned regards the size of the Internet of Things. As an important slice of the big data domain, the Internet of Things is worthy of thorough investigations and updated predictions because of the huge expected variation and exponential growth of the data size, right? Not so. All papers quoting the IoT as encoding 50 to 100 trillion objects by 2030 can be tracked back to a single book written by J. B. Waldner, “Nanocomputers and Swarm Intelligence”. Notably, the original French version of this book was published in 2007, which, knowing publishers, pinpoints the writing stage as being 2006 at best. 2006 was, as predictions go, 100 years ago. It is very difficult to predict the future because it is impossible to guarantee the progress of a certain field or foretell innovation. We cannot know if an invention will happen by a certain date even when that date is near because research projects often run into unexpected bottlenecks. The idea for the Gravity Probe B originates from the 60’s, when it was proposed at a cost of $35M because most of the technology was readily available. The mission launched 43 years later in 2004, at a cost of $870M. This is a fair example of why it is wrong to use in 2016 data prophesied in 2006.
This book may very well continue to be quoted until the late 23rd century.
It is not surprising that the fuzzy state of the field triggers uninformed and counterintuitive reactions from the business executives at large. Apparently the majority of decision makers across 8 industrial sectors believe that they need to use big data or they will lose their market positions to the new entrants who are already using it to gain competitive advantage. These new entrants are possibly the companies from four paragraphs ago, who cannot use data science properly to make predictions. From a business perspective this is a serious loss of strategic thinking, because the main focus of manufacturers should be quality, price and customer service, exactly the same tools of the trade that have been successfully employed for centuries to promote business growth. A state of false urgency and inappropriate focus can only damage businesses.
Some useful information
However all is not lost. The size of a data set can be calculated in advance as a function of the number of variables, observations and the size of a variable in bytes. Sometimes the software adds pointers with each observation, very slightly increasing the size of the set.
where N is the number of observations, V is the number of variables and W is the average width of a variable in bytes.
At the same time, the study analyzing the size of the largest sets can function as a cutoff for the expected, business-as-usual set sizes. Another interesting point is that the information that can be extracted from data sets of various sizes plateaus after a certain number of data points, which means that data sets can be adjusted in terms of size. It is not yet clear how this adjustment should be done.
Research directions
The conclusion of this attempt to find quantitative descriptions of big data is that, with a few extremely competent exceptions, a thick fog of confusion and misunderstanding is plaguing the field. Companies are complaining about a lack of talents, but it is unclear how many of these companies follow through with their plan to hire data scientists and how many remain at a declarative level.
If anyone out there is about to start a research project, these are a few open questions.
It would be interesting to see a study investigating how many of the companies publishing job ads actually hire someone within 6 months, and out of these how many actually use the data properly and in a predictive manner. At this point, it seems that 65% of companies are using the data to monitor and control the equipment, an operational usage as opposed to prediction-based decision making.
These are all open questions and if someone preparing to start an investigation is, by any chance, reading this article, hopefully they will design their research to answers some of these points.