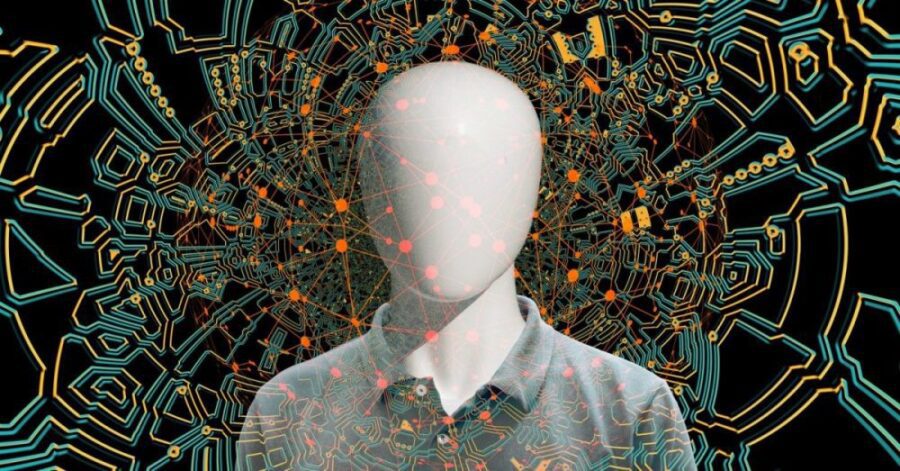
The emergence of deepfakes has presented both fascinating opportunities and formidable challenges in the digitally evolving landscape. Deepfakes, a portmanteau of “deep learning” and “fake,” are hyper-realistic digital forgeries created using sophisticated artificial intelligence (AI) algorithms. As these AI-generated images and videos become increasingly indistinguishable from reality, the role of data science in understanding, creating, and combating deepfakes has never been more critical.
The convergence of machine learning and AI in deepfake creation
Deepfakes are primarily powered by a subset of machine learning known as deep learning, particularly through algorithms like Generative Adversarial Networks (GANs). GANs operate on a principle of two competing neural networks: a generator that creates images and a discriminator that evaluates their authenticity. By training on vast datasets of real images or videos, these networks learn to produce highly convincing forgeries. This process exemplifies the core of data science – utilizing statistical methods to interpret complex datasets and extract meaningful patterns.
Data processing and analysis in deepfake technology
At the core of deepfake technology lies the rigorous data analysis and processing process. Data scientists employ advanced techniques to handle and interpret the large volumes of image and video data required to train deepfake algorithms. This involves preprocessing data, augmenting datasets to improve model robustness, and optimizing neural networks to enhance the realism of generated content. Efficiently processing and analyzing this data is pivotal in advancing deepfake technology.
Ethical implications and the responsibility of data science
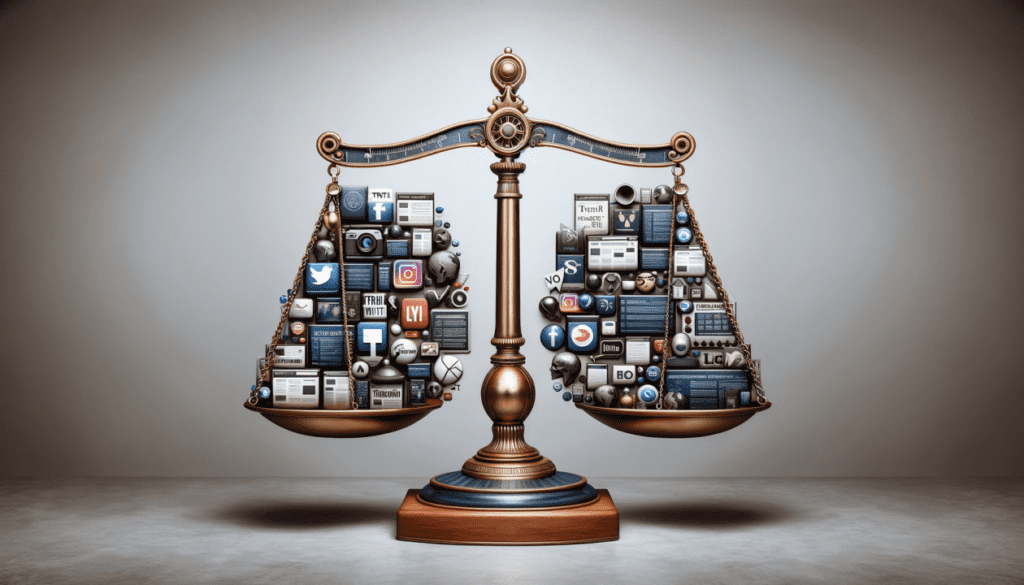
The creation of deepfakes raises significant ethical concerns, particularly regarding consent, privacy, and the potential for misinformation. As this technology’s creators, data scientists must upskill through Data Science course online and be responsible for addressing these ethical challenges. This involves developing guidelines for the responsible use of deepfake technology, ensuring transparency in AI-generated content, and advocating for policies that prevent misuse. The ethical dimension of data science is crucial in maintaining public trust and ensuring that deepfake technology is used for beneficial purposes.
The critical role of data science in deepfake detection
As deepfakes become more sophisticated, distinguishing between actual and manipulated content becomes increasingly challenging. Here, data science plays a vital role in developing detection methods. These methods often involve training machine learning models to recognize subtle inconsistencies typical in deepfakes, such as unnatural eye blinking patterns or irregular facial expressions. The field of data science contributes significantly to enhancing these detection algorithms, employing techniques like feature extraction, anomaly detection, and temporal analysis.
Challenges and innovations in deepfake detection
Pursuing effective detection methods presents significant challenges and opportunities for innovation. As deepfakes become increasingly sophisticated, traditional detection techniques often struggle to keep pace, leading to an ongoing ‘arms race’ between creators and detectors of these AI-generated forgeries.
- Current state of deepfake detection
The current methodologies in deepfake detection predominantly revolve around machine learning algorithms. These include facial recognition analysis, which scrutinizes subtle irregularities in facial features, and pattern inconsistency identification, focusing on anomalies in digital footprints that are not typically perceptible to the human eye. Behavioral signal analysis is another emerging technique, examining nuances in human behavior that often need to be more accurately replicated in deepfakes.
These methods encounter limitations, particularly as deepfake technology evolves to correct its inaccuracies. The adaptability of deepfake algorithms means that detection tools must continuously evolve to identify new patterns of falsification.
- Innovative approaches in detection
In response to these challenges, data science is spearheading innovative approaches to enhance deepfake detection. One such approach involves advanced deep learning models that analyze audio-visual inconsistencies. These models are trained on substantially large datasets to discern discrepancies in speech patterns, facial movements, and background noise that are characteristic of deepfakes.
Another promising avenue is the utilization of blockchain technology for digital content verification. By creating immutable digital content records, blockchain can provide a verifiable history of media files, making it easier to distinguish original content from manipulated versions.
- The role of data science in advancing detection
The advancement of deepfake detection is heavily reliant on data science. Data scientists are at the forefront of developing more advanced and structured algorithms that can learn and adapt to the evolving nature of deepfakes. This involves technical expertise and a deep knowledge of the ethical outcomes of AI and machine learning.
Collaboration across various sectors, including academia, industry, and government, is crucial. Data scientists are working alongside policymakers to establish standards and protocols for deepfake detection, ensuring a unified approach to tackling this issue.
Pushing the boundaries through research and development
The ongoing research and development in the field of deepfakes are heavily reliant on data science. This research is not only focused on improving the realism of deepfakes but also on understanding their limitations and potential applications. For instance, data science research is exploring the use of deepfakes in entertainment, education, and even in creating digital avatars for virtual meetings. Simultaneously, there is a concerted effort within the data science community to develop more robust detection mechanisms to counter malicious uses of deepfakes.
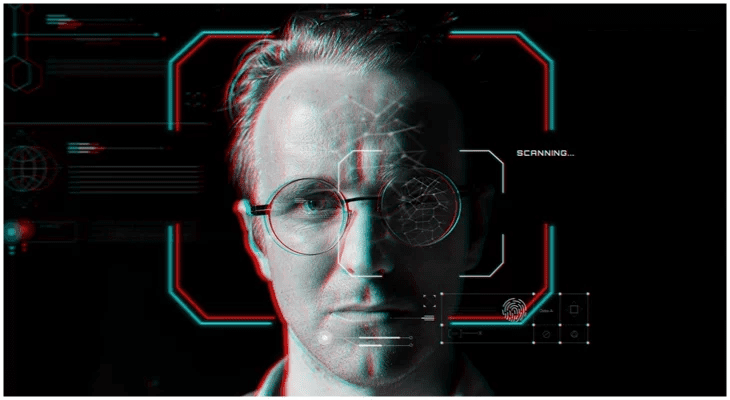
Future implications and the evolving landscape
As technology advances, deepfakes and data science interplay is poised for significant evolution. With the increasing accessibility of AI technology, deepfake creation is becoming more widespread, presenting both challenges in terms of potential misuse and opportunities for innovative applications in fields like entertainment, education, and communication. In this evolving landscape, the role of data science is crucial, balancing the drive for technological innovation through the best Data Science course with ethical responsibility. Data scientists must navigate these developments with a focus on advancing detection methods, ensuring responsible usage, and collaborating across disciplines to shape the future of deepfake technology.
Deepfakes is inextricably linked to the field of data science. From the algorithms that create these digital illusions to the methods employed to detect them, data science is fundamental to understanding and shaping the future of deepfake technology. As we venture further into this era of AI-generated content, the role of data science will be pivotal in harnessing the potential of deepfakes while safeguarding against their risks. The journey ahead is complex and fraught with challenges, but the possibilities are boundless with responsible stewardship and continued innovation in data science.