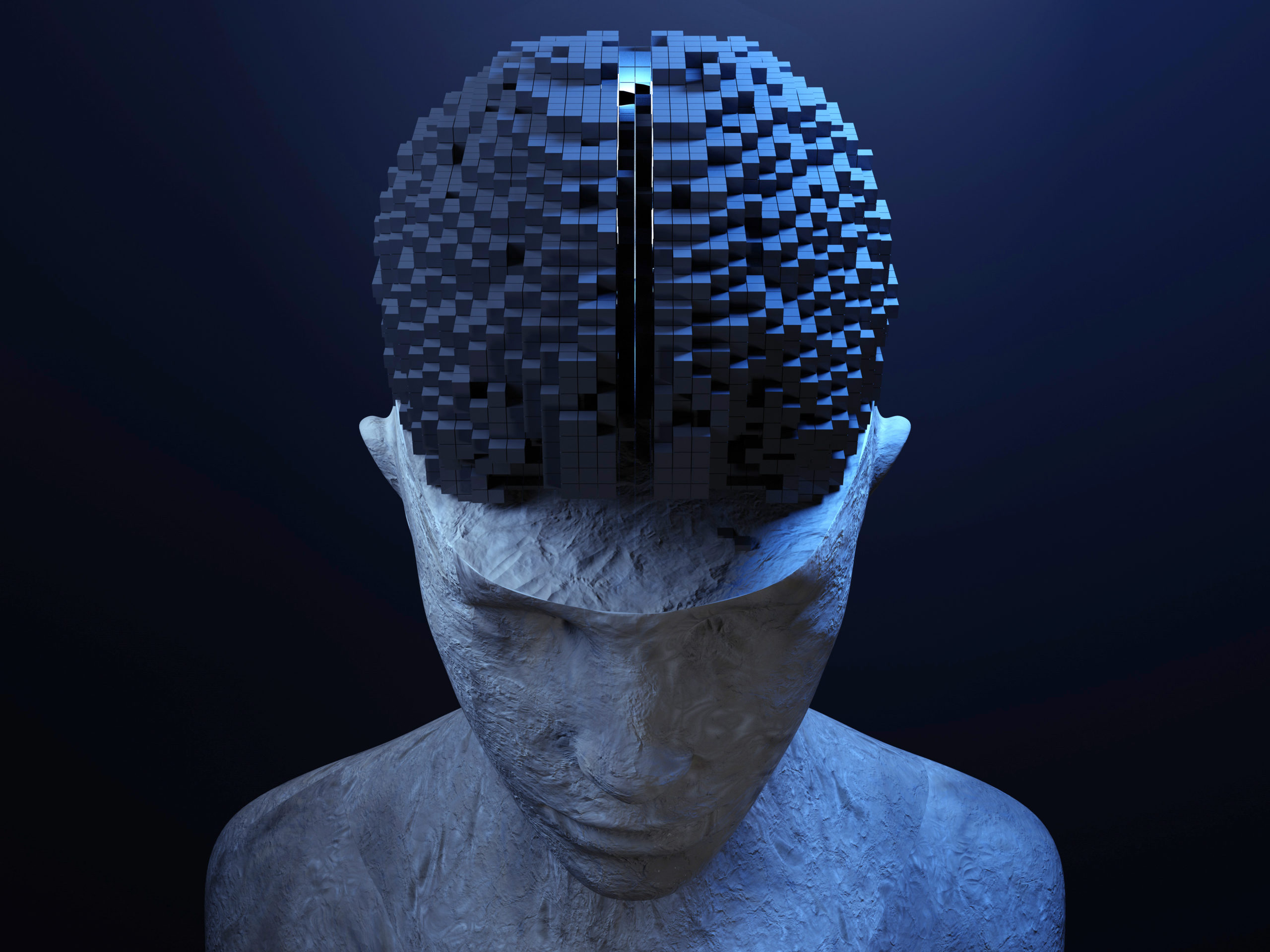
Understand how AI can be counterproductive, the need for adopting an Ethical & Responsible AI Framework, and the necessary principles you need to build one.
Since the invention of Artificial Intelligence, many enterprises have adopted it in their operations for various reasons. From helping people identify the shortest distance to their destinations to solving high-impact problems like climate change, AI has been used in every field.
However, there have been situations where AI has become counterproductive to organizations costing them their reputation and money.
In March 2016, Microsoft launched its chatbot named Tay on Twitter. The main objective of Tay was to use a conversational style of language in addressing and solving customer issues. Sadly, some people learned the chatbot algorithm and influenced it with hate tweets and inflammatory remarks. Tay later published some objectionable tweets and Microsoft had to take down the tool.
Microsft launched chatbot Tay tweeting about genocide.
In another example, Amazon developed a recruitment tool to expedite the hiring process and select the best candidates. Unfortunately, the AI was fed with biased data which made the tool prefer white men over other candidates. Amazon later dumped the tool on the grounds of misogyny and racism.
These are just a few examples that came into the limelight because of the organizations involved with them. In reality, most enterprises faced and are still facing such issues.
To prevent these issues, some companies are adopting a more accountable, fair, and understandable version of AI called Responsible AI. In simple words, Responsible AI is a framework that is followed by an organization to ensure their solutions and products adhere to the defined ethics and laws.
Responsible AI systems are generally built over a defined set of principles. Some organizations have well-defined principles in place, but most don’t.In this article, we list out and explain the bare minimum principles you would need to adopt Responsible AI in your organization.
Human-Centeredness
The very reason for using AI is to improve operations and processes thereby helping humans have efficient and (close to) accurate solutions. However, most AI development procedures do not involve the end user while designing the models.
Consider the example of using AI to identify routes to construct highways in a densely populated country like India. You might use data from satellite images, travel preferences of the people, the importance of the cities, travel frequency, and more data sources. But you will also have to consider all the stakeholders involved in the construction.
These are the people who own farms across the highway, the small business owners who run shops, and other similar people. Failing to involve all stakeholders might lead to delays in the construction due to non-cooperation and disagreement by some stakeholders.
The best way to address situations like these would be to involve a human in the loop to make sure all decisions are acceptable. But then again, the question of monitoring arises here. Practically, analyzing every decision made by the AI becomes a tedious task.
Having a model monitoring system like the one offered by Censius AI helps you track every decision. Once the right set of data and all the necessary parameters are ingested into the models, the Censius Monitoring platform analyzes all outcomes to check for drifts, anomalies, and any data quality issues.
Fairness
The reasons behind a successful AI model are the quality of data used and the people who build it. Ensuring fairness in both these sources allows you to avoid problems such as the Amazon recruitment tool.
One of the factors that make your AI smart is the people who build it. It becomes more important if the solution is going to be used by people from multiple cultures, races, genders, and backgrounds. To provide an example, consider a model used to solve a global problem like climate change. Since climate change has different effects on different regions of the world, you will have to involve a diverse set of people to address it effectively.
The second factor is the data used to build the model. Every region of the world has its societal history. Some places have a history of gender discrimination, some have a history of racism and some have a history of classism. Although we as a society have progressed, there are still unconscious biases that are transferred into the systems we use.
The data generated by these systems are later used by the AI model to build solutions. Ensuring this data is checked for any of such biases before moving the model into production plays a huge role in building fair and just solutions.
Building an all-inclusive but diverse ML team is a good approach here. People from different backgrounds bring rich and varied perspectives to problem-solving. Having these experts ensures the data fed into the models is free from bias to some extent.
Privacy
When you are building an AI model, you are dealing with a lot of data. Model efficiency increases as you feed it with varied data. But, dealing with such huge data is quite a responsibility.
Most governments today have strict data guidelines to keep data privacy issues in check. The data might involve sensitive information of your users, coming from various sources. Ensuring this data is obtained with user permissions and securing it should be your most important concern. Violating the data privacy of your users will not only lead to lawsuits but will also hurt the reputation of your enterprise.
It is your responsibility to ensure you use the data collected from your users responsibly. The data you collect has the power to build models that influence people. Using it to improve or harm the world is an ethical question that needs to be highlighted and remembered while building models.
Allowing users to see what data is collected from them and how you use it helps you build credibility. Maintaining data privacy documents to help users understand these aspects makes them easily accessible too.
Transparency
Creating an AI model that delivers 100% efficient results is practically impossible. There are so many changes that a model goes through in terms of data and the scenarios surrounding it. Due to this, the decisions made by the model seem incoherent at times.
Understanding the decisions becomes very important in such situations. To enable this level of transparency, models will have to be designed in such a way that any user can access the decision-making process of the model – be it the data fed or the algorithm used.
Also, with the range and prominence of the decisions that are made by AI, most governments hold organizations accountable for them. All these factors lead you to design explainable or transparent AI models.
For example, consider a bank using your model to determine loan approvals. Two people with the same income, credit score, age, educational background, and race apply for a loan. The model approves the loan application of one person and rejects the other. The other person then has the right to claim the reason for this.
In such situations, if you can extract the entire decision-making cycle of your model, you can easily understand and explain it to the user. Having this level of transparency pays you well in ensuring your models abide by the ethics and laws.
Our AI observability platform helps you bring required transparency in ML outcomes with continuous monitoring of them and root-cause analysis capabilities.
Security
Model security is the most important aspect of the entire ModelOps process. Your models are built to augment human decision-making so that there is increased efficiency. But if your models are not secured, they might end up taking you down along with them.
The example of Microsoft Tay is one such scenario. Of course, the chatbot was learning from user tweets. But those tweets were not monitored in the first place. A controlled data set would have generated a very different result.
Security not only involves data but also the people who access it. People having access to the algorithm and the data that is fed to your model reduces your control over it. The model needs to be managed by people who understand the data and the algorithm. The responsibility of this falls onto the higher management or the C-level executives.
Determine Your Principles For A Responsible AI Framework
A responsible AI framework has become a need for more than a choice. With the implications your models have, as well as the data that they work with, ensuring a responsible approach is of utmost importance.
The principles described in this article are widely used by most organizations to build a successful Responsible AI framework. You can either implement these principles directly into your framework, add some over these, or come up with principles of your own. The best method to decide on these principles would be to go back to your organization’s mission, vision, and values. Combined with what problem you are solving using AI, you can set well-defined principles to build a Responsible AI framework.
References:
- Ten Principles of Responsible AI for Corporates by Anand S Rao – https://towardsdatascience.com/ten-principles-of-responsible-ai-for-corporates-dd85ca509da9
- Artificial Intelligence at Google: Our Principles – https://ai.google/principles/
- The 4 Foundations of Responsible AI by Mattew Nolan – https://www.cmswire.com/information-management/the-4-foundations-of-responsible-ai/
- Principles to Practices for Responsible AI: Closing the Gap by Daniel Schiff, Bogdana Rakova, Aladdin Ayesh, Anat Fanti and Michael Lennon – https://arxiv.org/abs/2006.04707
- Explainable Artificial Intelligence (XAI): Concepts, taxonomies, opportunities, and challenges toward responsible AI – https://www.sciencedirect.com/science/article/pii/S1566253519308103
- Federal Government Regulation of AI by Joel Nantais – https://towardsdatascience.com/federal-government-regulation-of-ai-4fa08b7bd99a
- In 2016, Microsoft’s Racist Chatbot Revealed the Dangers of Online Conversation by Oscar Schwartz – https://spectrum.ieee.org/tech-talk/artificial-intelligence/machine-learning/in-2016-microsofts-racist-chatbot-revealed-the-dangers-of-online-conversation
- Amazon scraps secret AI recruiting tool that showed bias against women by Jeffrey Dastin – https://www.reuters.com/article/us-amazon-com-jobs-automation-insight/amazon-scraps-secret-ai-recruiting-tool-that-showed-bias-against-women-idUSKCN1MK08G