A Look at TEA’s AI Grading System with Caleb Stevens and Dan Wilson
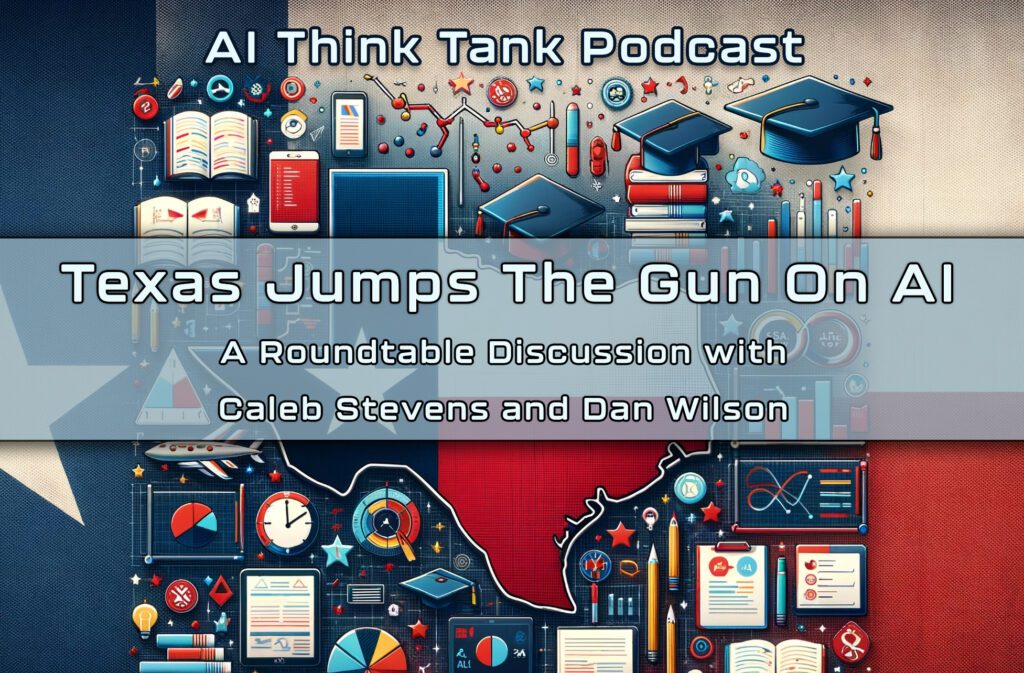
The recent implementation of artificial intelligence by the Texas Education Agency (TEA) to grade the State of Texas Assessments of Academic Readiness (STAAR) tests has sparked significant debate. For show #7 of the AI Think Tank podcast, I had the pleasure of discussing this topic with Caleb Stevens, an infrastructure engineer, founder of Personality Labs, and fellow MIT cohort of mine.
Caleb also used to be the manager of Enterprise Systems at National Math and Science, a non profit organization that specializes in helping teachers teach STEM education in high schools. Together we both share backgrounds in education as well as a passion for helping others truly understand what they are learning.
Context and Background
Caleb explained that previously, the state hired around 6,000 human graders annually to handle the STAAR tests. This year, however, they have reduced that number to fewer than 2,000, relying heavily on AI. This drastic reduction has raised eyebrows and questions about the reliability and fairness of AI in such a critical role.
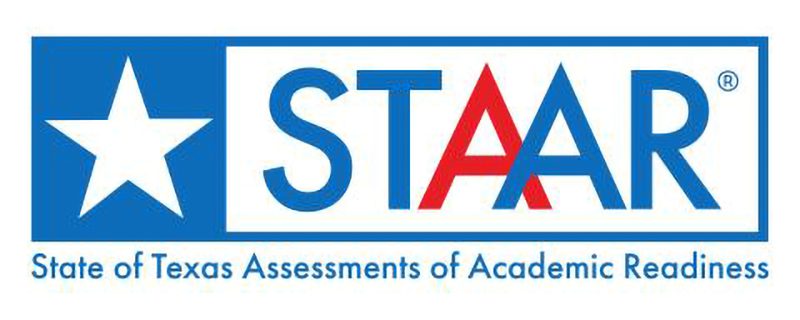
He also highlighted that the AI model used by TEA is said to be trained on just 3,000 human-graded responses. From the outset, this seemed woefully inadequate to both of us. We agreed that such a small dataset couldn’t possibly capture the diversity and complexity needed for accurate grading. That’s the size of a spreadsheet and not big data at all.
We also criticized TEA for not providing sufficient information about the AI’s metrics, training data, or the model used. Without transparency, it’s impossible to assess the AI’s effectiveness and fairness. Caleb noted that “they haven’t released any verifiable metrics, any kind of facts or information on the type of AI they’re using, the models or statistics that they claim to be training on. This lack of transparency is a major red flag.”
Concerns About Bias and Fairness
One of the significant issues Caleb raised was the potential for bias in AI models. Given the limited and potentially non-representative training data, the risk of bias is high. Without knowing the specifics of the AI’s training, it’s hard to gauge the extent of bias present in the system. Caleb mentioned that “we don’t know the training data that was used on this. We don’t know the base model that they’re using this data for, so we don’t know what built-in biases or other things that could be present with this model.”
We both agreed on the need for human oversight to mitigate these risks. Initially, AI should work alongside human graders to ensure accuracy and fairness. Caleb stated that “using artificial intelligence to assist humans in grading would have been a better approach.”
Implications for Teachers and Students
The shift to AI grading has resulted in significant job losses among human graders, which was a major point of concern for us. This reduction not only affects those graders economically but also removes experienced educators from the grading process.
Rebecca Bultsma, an AI Literacy & Ethics Advocate, joined our discussion and highlighted the importance of teachers in the grading process. She noted that grading helps teachers improve their teaching methods and understand student needs better. Rebecca emphasized that “one of the most valuable experiences teachers can have is going and doing marking of the standardized tests, because they’re exposed to a wide range of test answers and things that they might not be exposed to in their own school.”
Rebecca also stressed the need to involve parents in the discussion about AI in education. Parents need to understand how AI impacts their children’s education and have a say in how it is implemented. She said, “if the parents don’t understand how to support at home and the implications, they’re not going to jump on board, they’re going to fight against things like this.”
Historical Context and AI Capabilities
Caleb provided a historical overview of AI, tracing its roots back to the 1950s and explaining how public perception of AI has been shaped by popular culture, which often instills fear and misunderstanding. He remarked, “artificial intelligence isn’t necessarily the Terminator. It’s not out to find Sarah Connor or to take over the world. It’s really, right now, just an assistant to help humanity.”
Despite significant advancements, AI still has limitations, including issues like hallucinations and context loss. These problems are exacerbated when the AI is trained on small datasets. Caleb pointed out that “in short context windows, this level of AI performs fantastically. But 3,000 responses is about the size of one context window for models like GPT-4.”
Financial Implications and Transparency
The TEA’s decision to implement AI grading is partly driven by cost savings. They claim to save up to $20 million, but there is little information on how these savings will be used. Caleb highlighted, “they haven’t said what they’re going to be doing with the money they’re saving. Are they just going to save it? Put it in the bank? Are they going to reinvest this back into schools?”
We both questioned whether the cost savings justify the potential drawbacks, such as job losses and possible inaccuracies in grading. Caleb noted that “they saw that they could save dollar signs and that’s what they went for.”
The lack of transparency about the AI’s operation, data practices, and decision-making processes is a major concern. Without clear information, it is hard to trust the system. Caleb emphasized, “if they would have released model information or something like that, more data and statistics about what they were using, how they were using it, I think would have alleviated a lot of these concerns.”
Pilot Programs and Gradual Implementation
We discussed that a more prudent approach would have been to start with pilot programs in select school districts. This would allow for thorough testing and gradual implementation based on verified results. Caleb agreed, noting that “the way they should have done it was probably run them side by side for the year, right? Aren’t they really doing that?”
By gradually increasing the proportion of AI-graded tests, the TEA could build confidence in the system while continuously refining the model based on real-world performance. Caleb added, “I think I would probably prefer to go the other way or to run two systems side by side for a year.”
Addressing Privacy and Ethical Concerns
Fellow cohort and Cybersecurity Specialist, Yemi Adeniran, raised concerns about the privacy of student data and how it is handled and protected. Ensuring the security and confidentiality of student information is crucial. Yemi asked, “what about the privacy concerns of student data and the handling of protection of students’ data in that regard?”
Caleb acknowledged these concerns and emphasized the need for robust data protection practices, although details on TEA’s current practices are lacking. He mentioned, “we would just have to make positive assumptions here and say that there are laws in the U.S. FERPA is one of them. That’s the Family Educational Rights and Privacy Act.”
Mo Morales, a parent and UX/AI Developer questioned whether students’ creative output is adequately protected, given that their work is being used to train AI models. He asked, “are these students, don’t they enjoy the protections of their own personal, private, creative output like anybody else?”
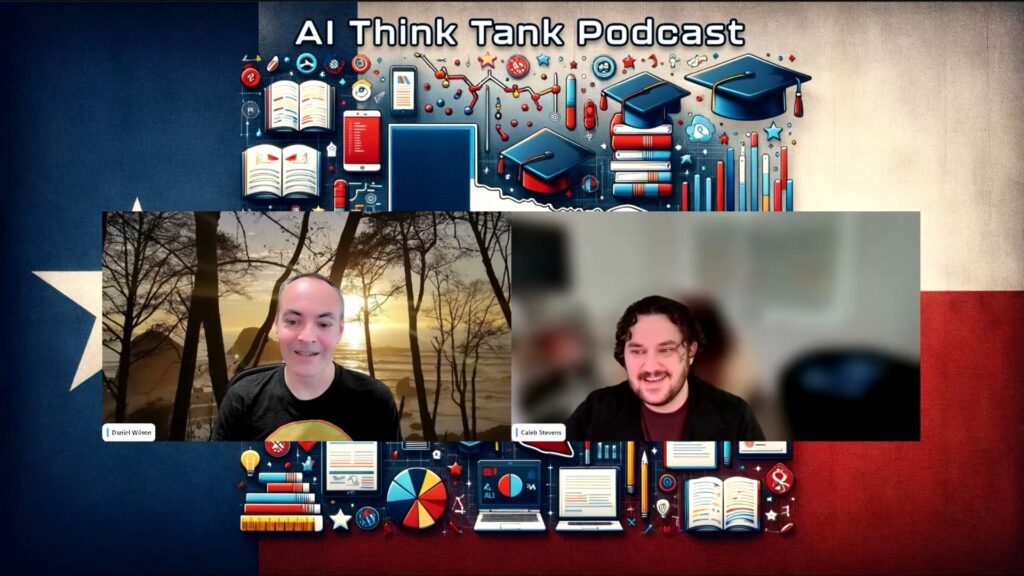
Comparing AI and Human Graders
Patrick Stingely, a Data Scientist and Security Specialist argued that AI might actually reduce bias compared to human graders, who can be influenced by personal biases and subjective judgments. He mentioned, “the computer actually has a better chance of providing unbiased results. One child’s content is going to be the same as another.”
Caleb countered that AI might not understand regional slang or cultural references, leading to unfair grading. He pointed out, “it could show bias because it just doesn’t understand the culture from which the writing is coming from.”
Both sides agreed that a balanced approach, where AI assists human graders rather than replacing them entirely, would be more effective. Caleb stated, “AI can and will assist us in that area. I believe that that probably would have been the better approach to begin with.”
Larry Sawyer, a Principal User Experience Designer with AI specialties made some solid points as well. “Something that Yemi mentioned made me think of this. I mean, it’s been a long time since I was in school, but especially with, like, math and science problems, you have to show your work. Like, you don’t get credit if you can’t show how you got to that solution, right?”
“Why is an educational institution not showing their work? Isn’t that part of their mandate for students?”
Larry followed that with more wisdom stating, “They’ve gone through and graded this stuff. I can see if this was like a private company where they would want to keep things secure and private, you know, from an intellectual property standpoint and other factors. But this is a public school system. Why the secrecy? Why isn’t there more transparency?”
For his final point and something we all agreed on, Larry added, “They have data. Like when some of the biggest challenges that I’ve seen with AI is finding a complete data set for you to be able to utilize and do your testing and your evaluation. Now, I would imagine they have years’ worth of tests and years’ worth of, you know, grades and things that they could go through and and correspond, you know, grade those things at 75-25 and compare it to the grades from the previous years. That’s how you do your testing. You don’t do testing on live data in a live environment. I mean, I don’t understand why they would do that.”
Parental Concerns and Choice
Rebecca emphasized the importance of involving parents in discussions about AI in education. Parents need to understand the implications and have a voice in decisions that affect their children. She said, “if the parents don’t understand how to support at home and the implications, they’re not going to jump on board, they’re going to fight against things like this.”
Parents should have the option to opt-out of AI grading if they are uncomfortable with it. TEA’s current policy requires parents to pay $50 for human grading, which Caleb criticized as unfair. He mentioned, “if you want the luxury of a human grading your paper, you’re going to have to pay $50 a child to do it.”
Learning from Global Perspectives
Rebecca shared insights from Canada, where the approach to standardized testing and funding differs significantly from Texas. Underperforming schools in Canada receive additional support rather than being penalized. She noted, “they’re not punished for underperforming, there is standardized testing. But I don’t think it at all impacts how much money schools get.”
Involving teachers in the grading process is seen as valuable professional development. Teachers benefit from exposure to a wide range of student responses. Rebecca emphasized, “one of the most valuable experiences teachers can have is going and doing marking of the standardized tests, because they’re exposed to a wide range of test answers.”
Conclusion and Recommendations
Several key concerns with TEA’s AI grading implementation stood out, including inadequate training data, lack of transparency, potential bias, job losses, and the impact on education quality. I would love to see working guidelines established for accomplishing safe and accurate testing going forward.
To address these concerns, a more refined and robust approach is recommended:
- Expand Training Dataset: Use a minimum of 100,000 human-graded tests to train the AI, ensuring diverse and comprehensive data.
- Diverse Sampling: Include data from various demographics and regions to minimize bias.
- Human-AI Collaboration: Initially use AI alongside human graders with continuous feedback loops.
- Robust Testing and Validation: Employ cross-validation and regular bias checks.
- Transparency and Peer Review: Make data and methodologies publicly available for review and engage independent third parties for audits.
- Incremental Rollout: Start with pilot programs and gradually increase AI grading.
- Regular Updates and Retraining: Continuously update the model with new data and retrain to adapt to changes.
- Involve Parents and Teachers: Ensure parents have a voice and provide options to opt-out of AI grading if desired.
- Clear Communication: Be transparent about the use of savings and how they will benefit the educational system.
Our discussion underscored the importance of addressing the heartfelt concerns of all stakeholders, including teachers, parents, and students. It is crucial to ensure that the implementation of AI in education enhances the learning experience without compromising fairness, transparency, or quality.
By taking a cautious, well-structured approach, the TEA can build a reliable and effective AI grading system that benefits everyone while maintaining trust and accountability. We hope that with this information, you have more insight and make the right decisions should this impact you, your family, and your community.
Join us as we continue to explore the cutting-edge of AI and data science with leading experts in the field.
Subscribe to the AI Think Tank Podcast on YouTube.
Would you like to join the show as a live attendee and interact with guests? Contact Us