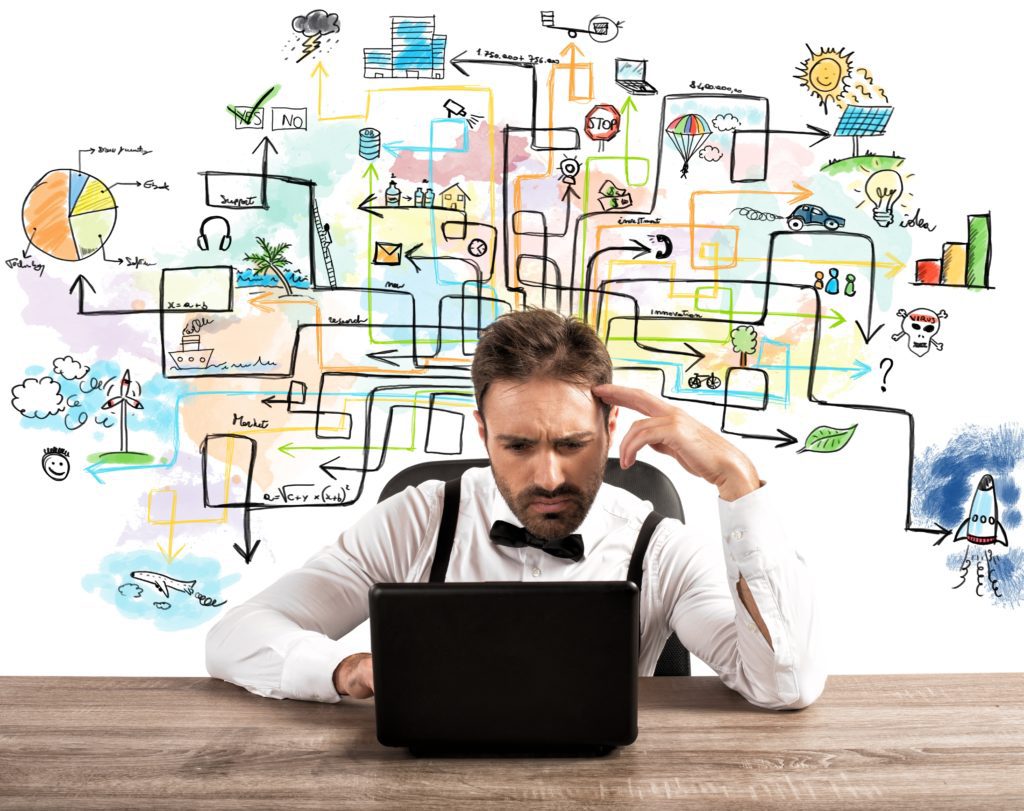
I love this infographic recently floating around LinkedIn. Sorry, don’t know to whom to give credit, but it does provide an interesting depiction of how senior management thinks AI works and the realities of what’s required to make AI work (Figure 1).
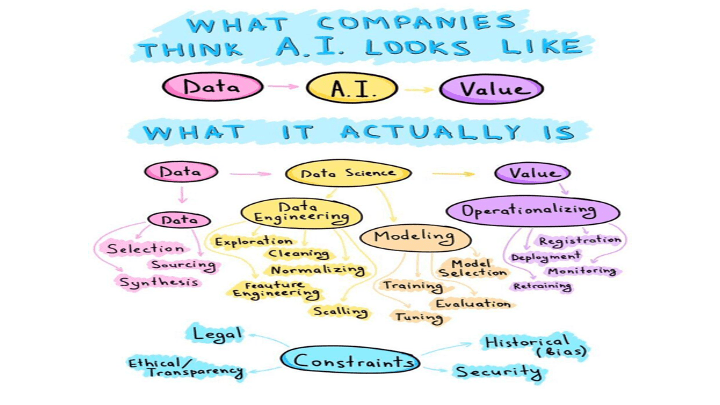
Figure 1: What Companies Think AI Looks Like
It’s a fun visual but missing the most critical high-level circle – Intent.
Intent is an understanding and clarification of the intended need or objective defined at the beginning of the process.
Intent is the why we are on this journey. Understanding Intent requires a detailed articulation of what are you trying to accomplish (e.g., objectives, need, purpose), what are the KPIs and metrics against which you will measure progress and success, who are the different stakeholders and constituents who will be involved in the scoping and execution of business objectives, what are the key decisions that these stakeholders need to make in support of the objectives and what are the KPIs and metrics against which they will measure progress and success, what are the Desired Outcomes, what are the potential costs associated with making the wrong decisions (critical for understanding the ramifications of False Positives and False Negatives), what are the ramifications of objective or nee failure, what are the potential unintended consequences…should I keep going (Figure 2)?
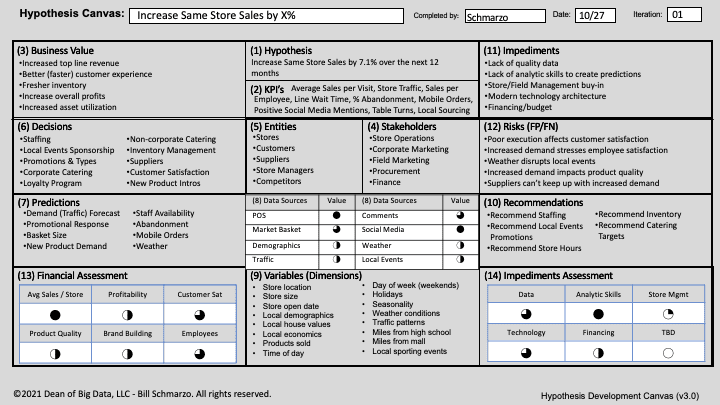
Figure 2: Hypothesis Development Canvas
Yes, Intent is everything, and the Hypothesis Development Canvas in Figure 2 – and the supporting “Thinking Like a Data Scientist” methodology – can help us understand and clarify “Intent.”
But even if we have an understanding and clarification on intent, leadership still seems very quick to come up with excuses as to why they suck at AI. But remember, excuses are not reasons. So, let’s triage 3 “excuses” that recently popped up on LinkedIn around the topic of senior management and AI deployment.
Excuse #1: We Don’t Have the Right Talent
“We can’t hire the right talent and don’t have bottomless budgets to spend on complex technologies and massive cloud costs to train the AI / ML models.”
Counterpoint to #1 – If organizations do the proper amount of pre-work to clearly define the problem they are trying to solve, identify the KPIs and metrics against which success will be measured, and empower the front-lines and subject matter experts to identify the variables, metrics, and “features” that might be better predictors of performance, then organizations don’t need unicorn data scientists and overwhelming compute power to “brute force” the AI model development process (Figure 3).
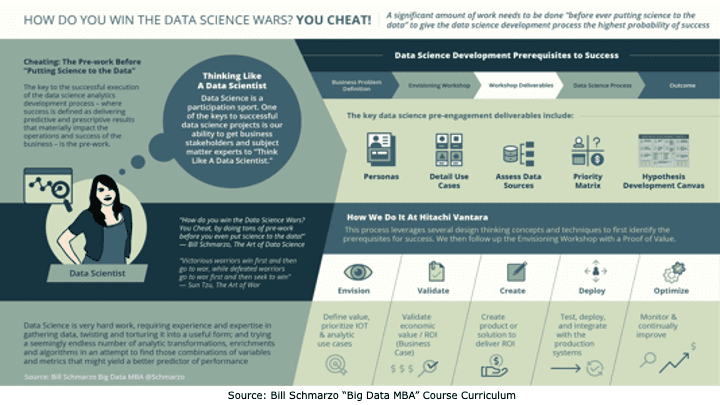
Figure 3: “How Do You Win the Data Science Wars? You Cheat!”
As I discussed in the blog “How Do You Win the Data Science Wars? You Cheat!”:
The key to the successful execution of the data science development process – where success is defined as delivering predictive and prescriptive results that materially impact the operations and success of the business – is the pre-work. This means not an hour or so of “showing up and throwing up’, but an orchestrated business stakeholder and subject matter expert engagement process that ensures that the data science team thoroughly and intimately understands the business opportunity under consideration, understands the metrics and KPI’s against which progress and success will be measured, has identified, validated, valued and prioritized the decisions that need to be optimized in support of the business opportunity and understands the costs of the analytics being wrong (the cost of False Positives and False Negatives). So how do we ensure data science success? Cheat.
Cheat by doing the necessary and extensive pre-work before we ever “put science to the data.”
Excuse #2: We Don’t Have Big Data
“Our company lacks the massive amounts of big data out of which we can mine customer, product, and operational insights.”
Counterpoint to #2 – Most companies already have sufficiently-size data sets; they just don’t understand how best to activate those data sets. Retail, manufacturing, transportation, logistics, healthcare, hospitality, entertainment, etc. have vast amounts of untapped data buried under the aggregations that support their reporting and dashboard needs. The problem isn’t having the data; the problem is identifying, validating, valuing, and prioritizing the use cases against which the organization’s data can be applied to create those new sources of customer, product, service, and operational value (Figure 4).
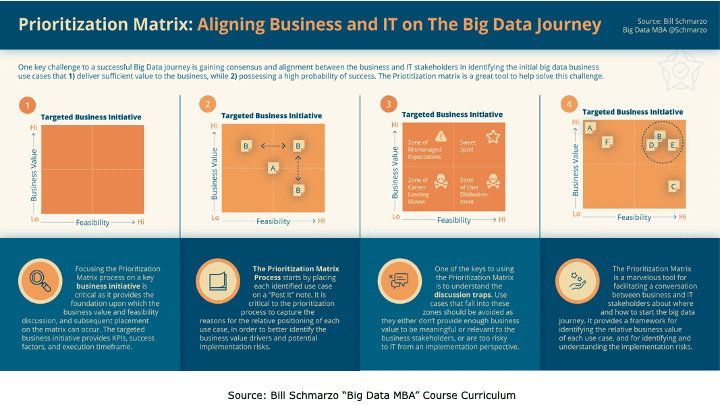
Figure 4: The Power of Use Cases
As I discussed in the blog “Building Value-driven Data Strategy: Use Case Approach – Part 2”, instead of a “Big Bang” analytics development and deployment approach, organizations are better served to employ an incremental, use case-by-use case approach where each use case pays for itself (meaningful Return on Investment) while allowing the organization to iteratively build out its data and analytics capabilities.
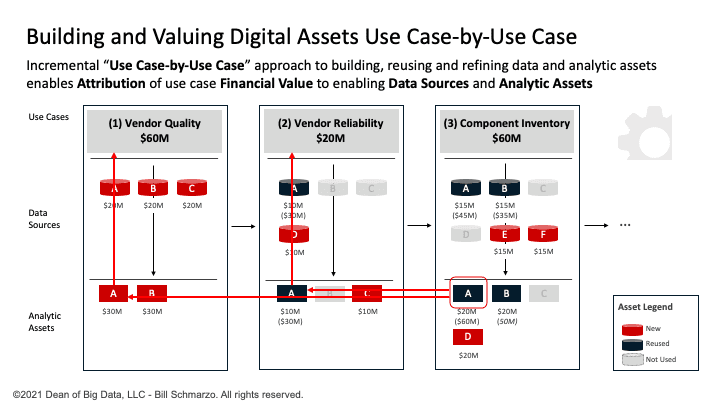
Figure 5: Big Data MBA Educational: Episode 17
This use case-by-use case approach also allows organizations to exploit the economies of learning.
Economies of Learning measures an organization’s economic value creation effectiveness based on a business model of continuous learning and adapting to constant changes in the business environment.
The Economics of Learning concept leverages AI / ML to create products, assets, processes, and policies that continuously learn and adapt to their constantly-changing operational environment…with minimal human intervention.
Excuse #3: Can’t Scale or Operationalize our AI / ML Efforts
“We don’t have the organizational skills and experience to sustain our data and analytic efforts. Instead, we end up with unrelated data projects, data silos, and orphaned analytics.”
Counterpoint to #3 – Ability to sustain is a cultural issue. And culturally, it’s a mindset of employee empowerment and ideation to identify where and how data and analytics can drive business and operational value that any organization of any size can adopt regardless of their technology chops. And the key to driving this cultural transformation is getting everyone one in the organization to Think Like a Data Scientist (Figure 6).
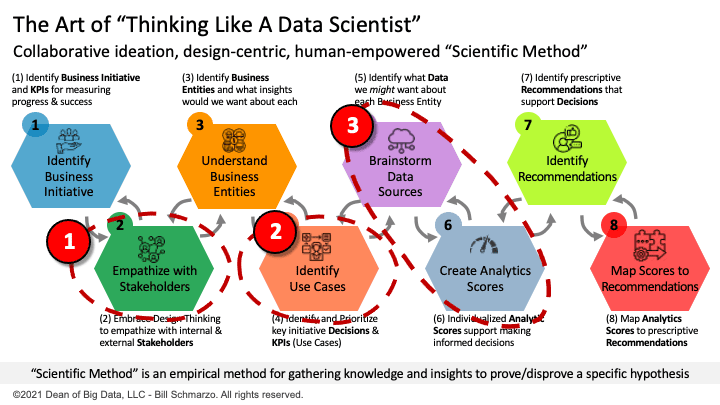
Figure 6: The Art of Thinking Like a Data Scientist
The “Thinking like a Data Scientist” process collaborates across a diverse set of stakeholders to identify, validate, value, and prioritize the decisions that these stakeholders need to make in support of the business initiative, and gathering the KPIs and metrics against which the effectiveness of those decisions are measured.
Subject Matter Experts and the front-line employees play a critical role in scaling and operationalizing AI / ML including (corresponding to Figure 6 Red circles):
- In defining where and how data and analytics can drive value against the organization’s targeted business initiative and identifying and brainstorming the KPIs and metrics against progress and success will be measured.
- Driving cross-organizational alignment and consensus on identifying, validating, valuing, and prioritizing the use cases that support the organization’s targeted business initiative.
- Identifying and brainstorming the variables, metrics, and features that might be better predictors of behavioral and performance propensities.
AI “Blame Game” Point – Counterpoint Summary
It’s just too easy for business executives to blame the complexity of the technology instead of looking in a mirror and holding themselves accountable for understanding (and envisioning) where and how big data and advanced analytics can derive and drive new sources of customer, product, service, and operational value. A big part of the AI / ML value creation challenges include:
1) Value Definition. Clearly defining, aligning, validating, and communicating how the organization is seeking to create value, and identifying and communicating the KPIs and metrics around which the organization will measure that value creation effectiveness
2) Cultural Transformation. Empowering everyone in the organization to be value creators which means disseminating responsibility AND authority the “lower levels” of the organization and accepting that experimentation and failure are critical and powerful learning vehicles)
Finally, I want to thank Jon Cooke, Somil Gupta and Mark Stouse (and many others) for their active contributions on LinkedIn on the topic of helping organizations derive and drive new sources of value leveraging big data and advanced analytics. They challenge me to articulate more clearly in how I’m going to teach these topics into my classes.