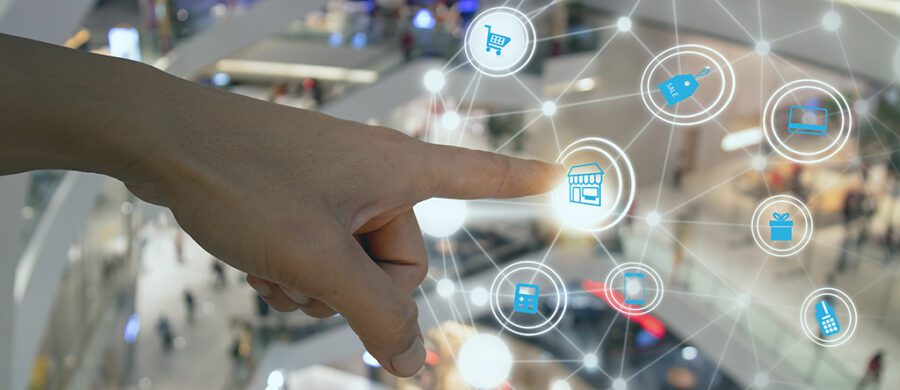
In Part 1 of the blog series on building a value-driven data strategy, I discussed the challenges associated with framing the data strategy process as a deliverable. A Data Strategy, like a Business Strategy, should ebb and flow depending upon what is “valuable” to the organization given the current business environment. Instead of thinking of your data strategy as a one-time deliverable, think of your data strategy as a continuous journey that evolves as the business environment evolves.
This necessitates a different approach to building an agile, continuously learning and adapting data strategy. We must move from data strategy as a “big bang” deliverable toward an agile, continuously-learning, value-driven data strategy journey. We can leverage the “economies of learning” to power our agile, living data strategy journey.
Economies of Learning measures an organization’s economic value creation effectiveness from a business model of continuous learning and adapting to constant changes in the business environment. the Economics of Learning leverage AI / ML to create assets, processes, and policies that continuously learn and adapt to their operating environment…with minimal human intervention.
To exploit the economies of learning, we will embrace a Use Case approach for iteratively developing our agile, continuously learning and adapting data strategy journey.
Power of Use Cases
Use Case is a cluster of Decisions around common KPIs or metrics that seek to deliver a well-defined business or operational outcome in support of an organization’s key business initiative.
Use Cases, and the decisions that comprise a use case, are a powerful vehicle for ensuring data strategy relevance for the following reasons (Figure 1):
- Decisions Are Easily Identifiable: Every business stakeholder knows what decisions they must make because they are already making those decisions today.
- Decisions Are Actionable. A decision infers an action to be taken (including a decision not to act), which is very different from a question which are used to validate or drive ideation.
- Improved Decisions Are a Source of Attributable Value. One can attribute and quantify the value (Return on Investment) of making an improved decision.
- Decisions can be Optimizable with Data Science. While decisions, for the most part, have not changed over the years, by data science, the answers have. The answers have become more granular, lower latency, and more accurate (precision decisions).
- Decisions Drive Organizational Collaborative. Optimizing decisions requires collaboration between Business stakeholders and the Data Science team to identify those variables and metrics (features) that might be better predictors of performance.
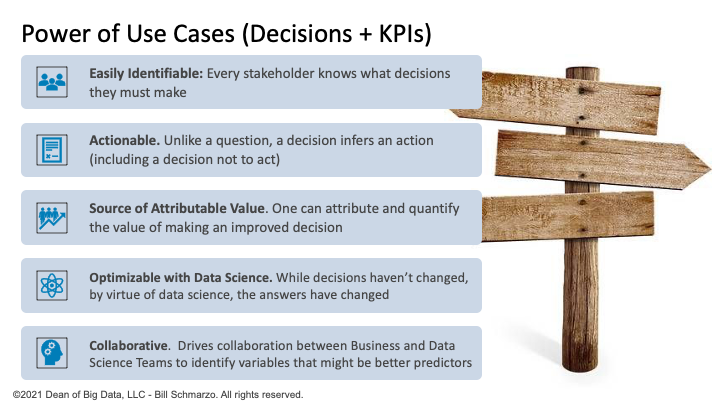
Figure 1: Power of Use Cases
Power of a Use Case-by-Use Case Data and Analytics Strategy Approach
A focus on use cases will be key in creating an agile, continuously-learning data strategy that ebbs and flows with the constant customer and market disruptions. Not only does a focus on use cases ensure business (ROI) relevant but it also allows us to exploit the data economic multiplier effect.
The Data Economic Multiplier Effect – enabled by the ability to share the same data set across an unlimited number of use cases at zero marginal cost – drives the accumulation of attributable and quantifiable value of each data set on a use-case-by-use-base basis.
Let’s introduce some key tools in helping to support our value-driven, continuously learning and adapting data strategy journey.
Tool #1: Prioritization Matrix to Identify and Prioritize Use Cases
Organizations don’t fail because of a lack of use cases; they fail because they have too many.
How do organizations address the overwhelming number of use cases problem? I teach the “Thinking Like a Data Scientist” methodology to drive organizational ideation and alignment around identifying, validating, valuing, and prioritizing the organization’s key business and operational use cases from a business value vis-a-vis implementation feasibility perspective (Figure 2).
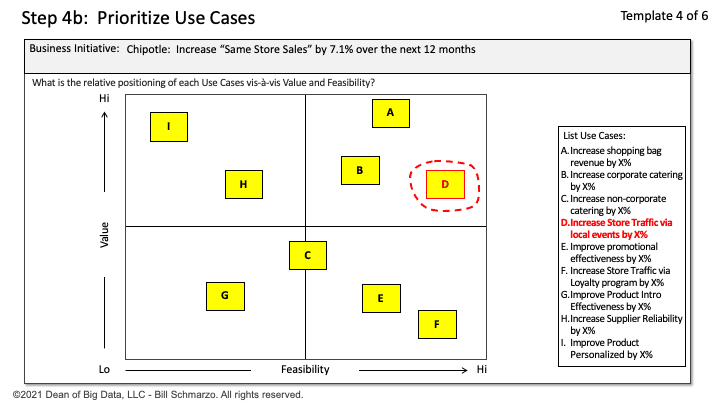
Figure 2: Prioritization Matrix
Check out “Big Data MBA Episode 13: Mastering the Prioritization Matrix” for a short video on how to effectively use the Prioritization Matrix to drive organizational use case alignment.
Tool #2: Use Cases Canvas to Drive Business and Data Science Alignment
A significant effort must be invested upfront to document the organization’s use cases. The Use Case design canvas in Figure 3 drives collaboration with the stakeholders to ensure we have a solid understanding of the use case.
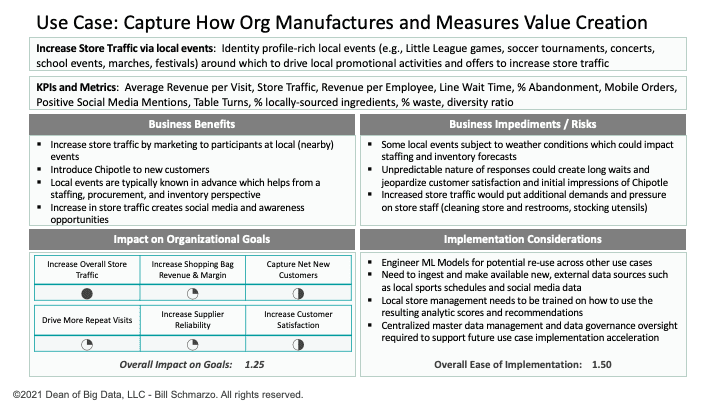
Figure 3: Use Case Canvas
The Use Case canvas captures the following information:
- Explanation of the use case including desired Business Outcomes
- The KPIs and metrics against which use case progress and success will be measured
- The expected business and operational benefits gathered across all the key stakeholders
- Potential impediments and risks
- Implementation considerations to address the potential impediments and risks
- Impact on the organization’s stated goals
Tool #3: Map and Quantify Relative Value of Data Sets
I detailed in my University of San Francisco research paper titled “APPLYING ECONOMIC CONCEPTS TO BIG DATA TO DETERMINE THE FINANCIAL VALUE OF THE ORGANIZATION’S DATA AND ANALYTICS, AND UNDERSTANDING THE RAMIFICATIONS ON THE ORGANIZATIONS’ FINANCIAL STATEMENTS AND IT OPERATIONS AND BUSINESS STRATEGIES” a methodology for attributing the financial value of a use case to the data sets that support the optimization of that use case (you can tell it’s a university white paper by the length of its title!!). The methodology uses a very straight-forward though tedious process to:
- Determine the financial value (financial accounting exercise) and implementation risks (risk management exercise) of each use case and then
- Quantify the predictive capabilities of the data sources in support of each use case (data science exercise)
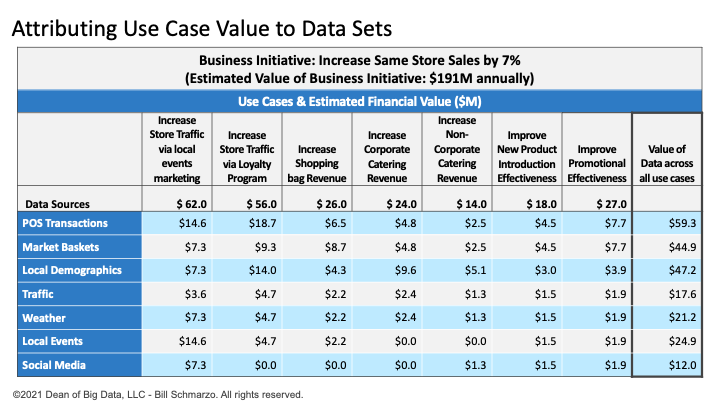
Figure 4: Attributing Use Case Financial Value to Data Sets
The result of the process provides a rough order estimate of the value of your data sets across your prioritized use cases (right hand column in Figure 4) which one can then use to prioritize the development of one’s data (data transformation and enrichment algorithms, data pipelines, master data management, data lake / data mesh architecture) and analytics (data visualizations, ML features, ML models, analytic scores, APIs) capabilities.
Note: You can get more precision in attributing the predictive relevance of each data set to the use case using advanced algorithms such as Random Forest, Principal Component Analysis, and Shapley Additive Explanations.
Tool #4: Iteratively Build out Data Lake / Data Mesh
Finally, one can take a use case approach to build out your data lake architecture based upon the relative value of that use case to the organization. One only needs to load, curate, and govern the data sets into the data lake necessary to support that use cases, thereby avoiding the “big bang” approach of loading all your data into a data lake and hoping that it provides value to your business stakeholders.
If you seek to exploit the unique characteristics of data – assets that never deplete, never wear out and can be used across an infinite number of use cases at zero marginal cost – then transform your data lake into a “collaborative value creation” platform that supports the capture, sharing, reuse, and refinement of your data assets across the organization (Figure 5).
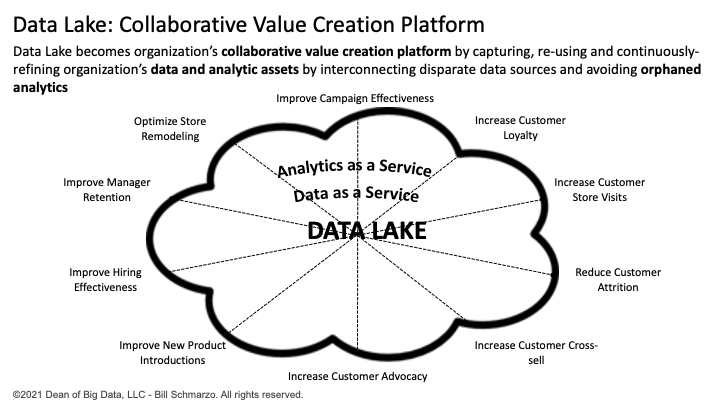
Figure 5: Data Lake as Collaborative Value Creation Platform
For example, if Use Case #1 in Figure 6 is “Improve Vendor Quality” (estimated financial value of $60M), then we only need to load and curate the data necessary to support that use case. And for Use Case #2 “Improve Vendor Reliability”, we only need to load and curate the data necessary to support that use case.
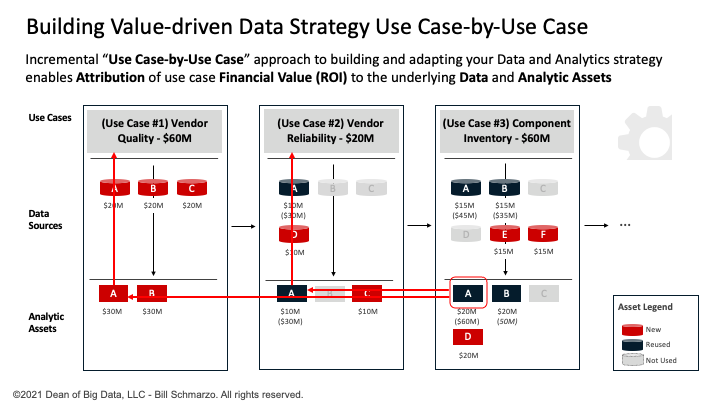
Figure 6: Building Your Data and Analytics Capabilities Use Case-by-Use Case
But wait, for Use Case #2, data set A has already been loaded into the data lake to support Use Case #1. Use Case #2 can use data set A at zero marginal cost. That’s the Data Economic Multiplier Effect in action!
Summary
Treating your data strategy as a static deliverable probably worked fine in a world that never changed. Today, however, your data strategy must be a journey of continuous learning and adapting. Your data strategy journey must be as agile as your business strategy.
Organizations don’t need a big data strategy; they need a business strategy that incorporates big data
Now, that’s easy to remember!
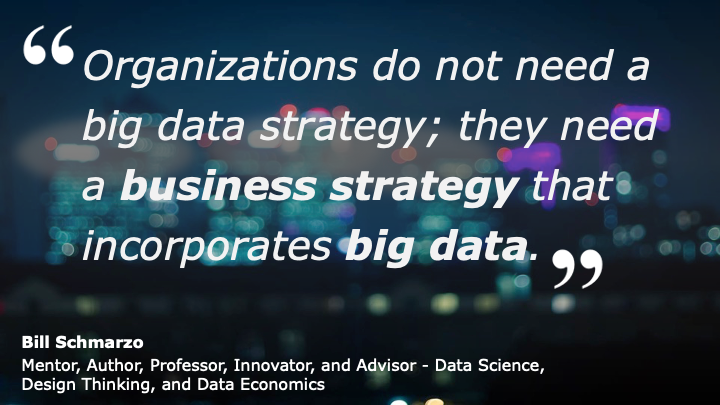