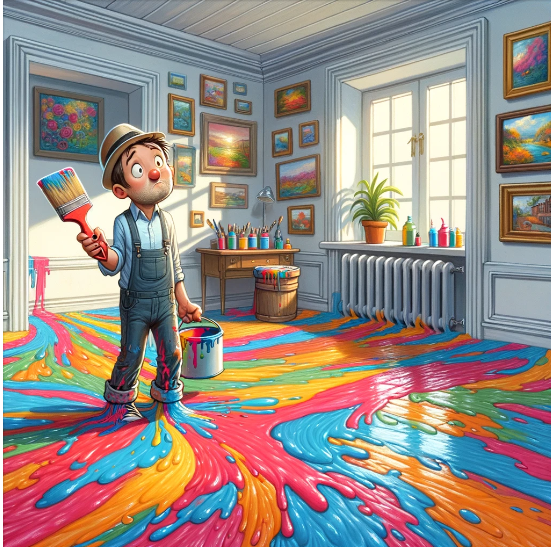
Image source: Dall-e
This week, the tech community has been abuzz with the announcement of the latest model from Mistral being closed source. This revelation confirms a suspicion held by many: the concept of open-source Large Language Models (LLMs) today is more a marketing term than a substantive promise.
Historically, open source has been championed as inherently positive. In doing so, it has increasingly been painting itself into a corner in its quest for seeing to be ‘good’.
At the heart of the open-source debate are the licensing frameworks: copyleft licenses, which mandate the public sharing of derivative works (ex the GPL licence). Over time, we had commercially friendly open source licenses where derivative works could be retained (ex the MIT licence).
The rise of cloud computing has further complicated the open-source landscape. As early as 2008, Richard Stallman warned of cloud computing’s potential to sideline open source by shifting the locus of control. This shift is now mirrored in the realm of LLMs, where the focus is not just on the models’ weights and architecture but also on the exclusive training data, which remains proprietary with all LLMs(open source or closed). This similarity between open and closed source models reveals that the challenges of transparency, bias, fairness, and privacy are universal, diluting the distinction between the two approaches.
Critics like Gary Marcus view open-source LLLMs with skepticism, fearing they pose unique threats.
The debate over open-source LLMs is reminiscent of broader discussions about the moral positioning of tech companies. Google’s recent controversies over Gemini images highlight the pitfalls of claiming a moral high ground in technology development. Such stances, while noble, can become untenable over time, especially when unforeseen challenges emerge.
In conclusion, the discourse surrounding open-source LLMs should be reframed to recognize them as one part of a broader ecosystem rather than a panacea. The development and deployment of LLMs are complex and resource-intensive processes that necessitate a pragmatic approach to innovation, collaboration, and regulation. As the landscape evolves, it will be interesting to observe how open-source initiatives adapt and how the community navigates the delicate balance between openness, innovation, and commercial viability.
The recent developments with Mistral and the ongoing dialogue around the AI act underscore the nuanced and multifaceted nature of these discussions, highlighting the need for a balanced and informed approach to the future of LLMs.
The views expressed here are my own and are not related to any organization I am associated with