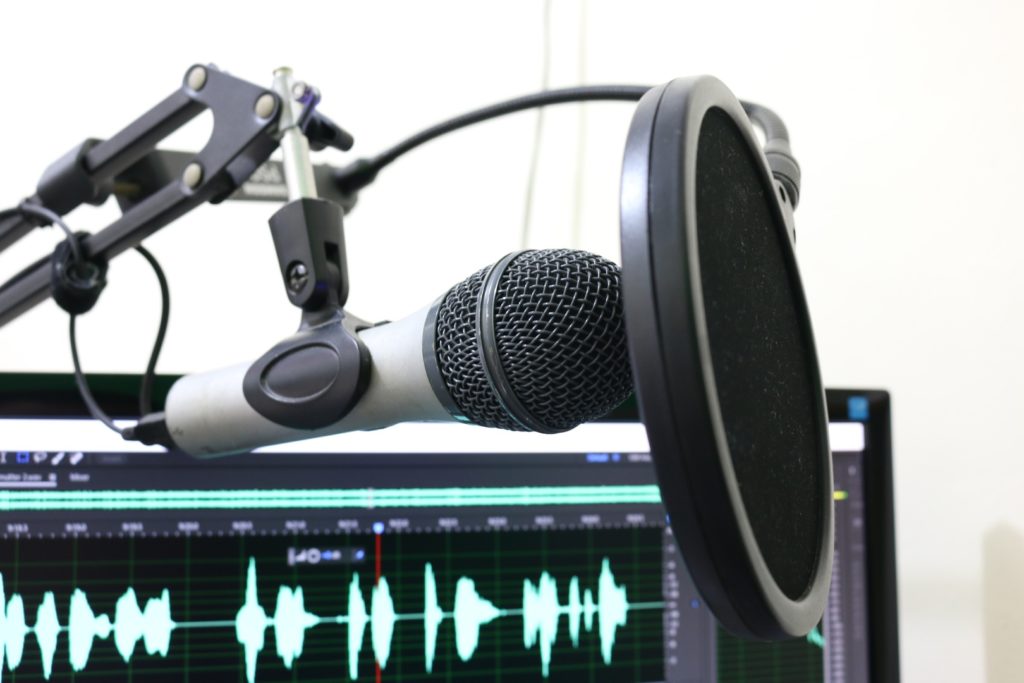
In mid-March 2023, I’ll be launching an interview podcast that will cover the most promising growth areas in FAIR data. TechTarget, parent company of Data Science Central (DSC), has graciously agreed to host the podcast.
You’ll be hearing my interviews with leading thinkers and innovators in next-generation data management, knowledge graphs, data architecture, advanced databases, the decentralized web (dweb), machine learning, natural language processing (NLP), and so many other related areas.
Along the way, you’ll be joining me in my research process as an analyst making sense of emerging technologies. I’ll post these interview recordings when I’m able to. So stay tuned to my DSC and LinkedIn channels for more details.
Why I’m doing a podcast
I love doing interviews with creative people who are focused on big, thorny challenges, and data quality is a huge challenge. As we move more and more toward a sharing economy, we’ll need more and more sharing, and more and more ways to assure the trustworthiness of what we’re sharing. We’ll also need less and less data duplication, to conserve energy and effort, which is why data-centric architecture is so important.
Posting interviews as well as blogs, other content and social media on these topics can have a symbiotic, third order network effect. People like to consume different kinds of content. I love text, but I also love audio. This series will be audio because it’s conversational, and audio is portable, something you can listen to while doing other things.
The podcast format is a friendly one that allows a more expansive exploration of issues together. I do hope you’ll join me to learn more about these topics and hear the stories of those who are hands on or otherwise committed to improving this data journey we’re on.
Framing the FAIR data challenge
The quest for quality data is clear Andrew Ng’s Landing.AI announced the launch of a new computer vision (CV) platform this past February. The company claims the CV platform’s algorithm requires less data to be able to deliver good results for companies without access to big data. The platform also offers AI-assisted collaborative labeling for training set data.
From Landing.AI’s website:
“Landing AI™ is pioneering the Data-Centric AI movement in which companies with limited data sets can realize the business value of AI and move AI projects from proof-of-concept to full-scale production.”
Andrew Ng is an advocate for quality data, and his company is headed in a positive direction. But ultimately, many of us want to do more to boost data quality than just conventional training set labeling.
Why FAIR data?
We have the means of going way beyond conventional training set labeling, a means that will alleviate the problems enterprises are facing with AI. Thing is, data that’s findable, accessible, interoperable and reusable (FAIR) isn’t getting enough attention. If there’s one thing missing from what we’re calling AI, it’s relevant, contextualized data, which is data (and accompanying description logic) enriched by reusable relationships, statements and rules. These relationships, statements and rules can all be contained in a knowledge graph
Smart, FAIR data isn’t just for AI. It’s for all the use cases that benefit from desiloed, logically interoperable data at scale.
Consider this example: In a hospital, each patient has a context, or really, a multiplicity of contexts: The state of their own health, their genomic makeup, the different patient cohorts they should belong to for the different conditions they’re suffering from….
Each patient deserves the available personalized medicine and treatment specific to their own situation. For that reason, placing the patient, the disease, the related patient cohort, and relevant research on diagnoses and treatments all have to be seen from the perspective of the user to be personalized.
Scale this capability, and targeted, data-centric solutions become possible for all sorts of issues.
Smart, FAIR data provides windows of strategic insight that other data can’t, because FAIR structured, document and imagery data develops synergies when woven into a cohesive whole. For example, data that contributes to the patient contexts I mentioned above must be sourced from dozens of different places. It’s best to connect and disambiguate this variety of data within a knowledge graph for FAIR and scalability reasons.
Goals of an organizational FAIR data program
The adoption of FAIR data principles–first published in 2016–is still in its infancy. Most of the FAIR data programs that I’ve seen are Federally funded projects run by principal investigators at universities. The typical use case described in the papers published after these projects is scientific research sharing.
The majority of the papers I’ve seen describe statistical AI model-oriented efforts; that is, the goal is a kind of alchemy where “raw” (but compressed and stripped-down?) data is the input to the model, and FAIR data is the desired output.
The programs the semantics community supports within corporations, by contrast (such as the Pistoia Alliance for life sciences companies), often focus on data preserved in its original, uncompressed, at-least-some-metadata-included state and then contextualized with the help of a semantic knowledge graph approach and humans, especially domain experts, in the loop with machines. Those programs would have goals that include the following:
- Boost effectiveness. Adherence to FAIR principles means the data you need to get more from can be much more useful, time and again.
- Boost efficiency. Using less data to get better results implies less energy and human effort over the long term.
- Avoid reinventing the wheel. FAIR principles open the opportunity to share and reuse each other’s FAIR data. Create once, use everywhere.
- Encourage a data-centric culture. FAIR principles place the emphasis where it belongs: data. Apps and models don’t need all the attention they’re getting.
I’m hoping The Fair Data Forecast series will provide clear, fresh examples of how companies and communities are working toward these goals. Take a listen and find out how others are getting involved and working together on this challenge.