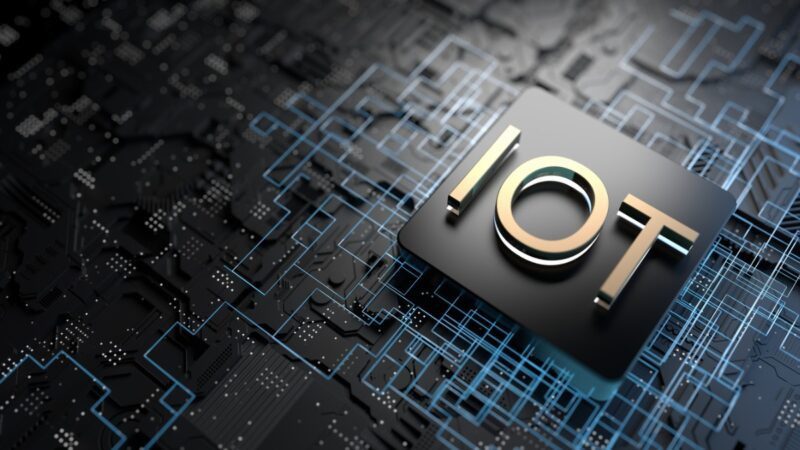
The Internet of Things (IoT) has revolutionized how devices communicate and interact, generating massive amounts of data. As IoT ecosystems continue to expand, the need for efficient data management becomes paramount. In this comprehensive article, we delve into the significance of data mapping in IoT environments. We will Explore its challenges, strategies, and implications for leveraging the full potential of IoT data.
Understanding IoT data complexity
IoT ecosystems comprise various devices, sensors, and platforms, each generating data in multiple formats and protocols. This heterogeneity presents a significant challenge for data integration and analysis. Data mapping is crucial in harmonizing these disparate data streams, enabling seamless interoperability and insights extraction.
Challenges in IoT data mapping
One of the primary challenges in IoT data mapping is the sheer volume and velocity of data generated by interconnected devices. Traditional data mapping tools may struggle to keep pace with real-time data streams, leading to latency and scalability issues. Moreover, the dynamic nature of IoT environments. Where devices join, leave, or change configurations dynamically, adds another layer of complexity to data mapping.
Strategies for effective data mapping in IoT
To address these challenges, IoT practitioners employ several strategies for effective data mapping:
Schema-on-read approach
In traditional data mapping approaches. Organizations can align IoT data streams with standardized ontologies. This ensures consistent interpretation and integration of data across diverse IoT deployments. Redefined schema is often enforced upfront, dictating the structure and format of incoming data. However, a schema-on-read approach is more pragmatic in IoT environments characterized by heterogeneity and variability. With schema-on-read, data is ingested in its raw form, and the schema is applied dynamically during analysis or processing. This flexibility allows IoT systems to accommodate diverse data formats and evolving device configurations without requiring extensive schema modifications. Organizations can achieve greater agility and adaptability in handling IoT data streams by decoupling data ingestion from schema enforcement.
Semantic interoperability
Semantic data mapping techniques promote interoperability across disparate IoT devices and platforms. These mapping involves establishing standard semantics or meaning for data elements, regardless of their physical representation or syntax. Ontology-based mapping is one such technique that relies on formal ontologies to define relationships and constraints between data entities. Organizations can align IoT data streams with standardized ontologies. This ensures consistent interpretation and integration of data across diverse IoT deployments. Semantic interoperability facilitates advanced analytics, context-aware processing, and knowledge discovery, unlocking deeper insights from IoT data.
Edge computing and data preprocessing
Edge computing brings computation and data processing closer to the IoT devices. It reduces latency and bandwidth requirements associated with centralized processing architectures. In the context of data mapping, edge computing allows for lightweight data preprocessing tasks. These tasks can be carried out directly on the edge devices. This includes data normalization, filtering, aggregation, and lightweight mapping operations. By preprocessing data at the edge, organizations can cut down the amount of data sent to centralized servers. This helps ease network congestion and enhances the system’s overall responsiveness. Edge data preprocessing also enhances privacy and security by minimizing data exposure during transit.
Dynamic mapping techniques
In dynamic IoT environments, device configurations and data formats often change frequently. As a result, static data mapping approaches are often inadequate. Dynamic mapping techniques adapt to these changes in real time, automatically adjusting mappings based on contextual information or historical patterns. Machine learning can be used to find and learn patterns in IoT data streams. These algorithms can then infer mappings between data elements, devices, or systems, optimizing data transformation and integration processes. Organizations can maintain agility and responsiveness in evolving IoT landscapes by embracing dynamic mapping techniques.
Data governance and metadata management
Establishing strong governance practices and metadata management frameworks is essential for effective data mapping in IoT ecosystems. Clear policies and standards ensure data consistency, quality, and compliance across IoT deployments. Metadata provides valuable context and documentation for data mapping processes, including schema definitions, data lineage, and semantic annotations. Organizations can track data provenance, lineage, and usage by maintaining comprehensive metadata repositories, facilitating traceability and auditability. Data governance frameworks also enforce data security and privacy policies.
Implications and benefits of data mapping in IoT
Efficient data mapping in IoT ecosystems yields several benefits:
- Interoperability and Integration: Data mapping facilitates seamless integration of heterogeneous IoT data sources, enabling comprehensive insights generation and decision-making.
- Data Quality and Consistency: By standardizing data representations and semantics, data mapping enhances data quality and consistency across IoT deployments, reducing errors and inaccuracies.
- Scalability and Performance: Optimized strategies improve scalability and performance in IoT applications, allowing organizations to handle growing data volumes and meet real-time processing requirements.
Future directions and emerging trends
Looking ahead, several emerging trends and technologies are poised to shape the future of data mapping in IoT ecosystems:
- Graph-based mapping: Graph databases and mapping techniques are powerful in representing complex relationships and dependencies in IoT data, enabling more nuanced analysis and insights generation.
- Federated data mapping: Federated data mapping approaches allow organizations to collaborate and share mapping knowledge and resources across IoT deployments, promoting interoperability and standardization.
- Integration with edge AI: Integration of edge artificial intelligence (AI) capabilities with data mapping processes enables intelligent data preprocessing, anomaly detection, and decision-making at the edge, enhancing real-time responsiveness and autonomy in IoT deployments.
Conclusion
As the IoT landscape continues to evolve, effective data mapping emerges as a critical enabler for unlocking the full potential of IoT data. By addressing the challenges of data complexity, interoperability, and scalabilit. Organizations can harness the transformative power of IoT to drive innovation and create value across industries. Data mapping paves the way for a connected, intelligent, and data-driven future in the IoT ecosystem through dynamic techniques, semantic interoperability, and edge computing capabilities. By embracing these strategies and emerging trends. Organizations can confidently navigate the complexities of the IoT landscape, driving continued growth and innovation in the digital era.