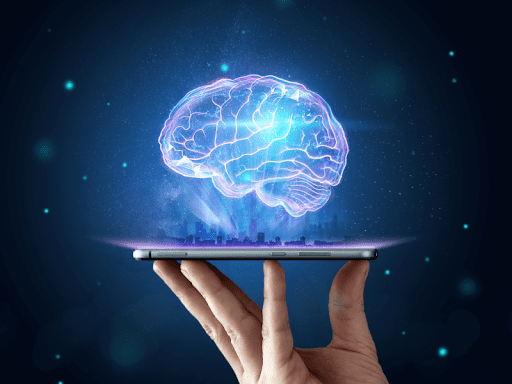
Given how quickly the digital marketing industry changes, keeping up with the most recent trends and technologies can be challenging. Traditional marketing techniques are no longer enough to connect with and engage your target audience.
Machine learning in marketing has got your back.
Check out the top 10 use cases and implementation pointers that offer useful advice for utilizing machine learning to boost ROI, tailor consumer experiences, and optimize marketing strategies.
Benefits of machine learning in marketing
Machine learning has completely changed the marketing industry by enabling companies to develop more successful and individualized marketing strategies through various benefits. Let’s examine the benefits in detail:
- Enhanced personalization: By analyzing large client data using machine learning algorithms, marketing messages, and content can be tailored to individual preferences. Higher customer engagement, higher conversion rates, and greater customer loyalty result from improved personalization. Businesses may give information and offers that resonate with consumers by understanding their unique tastes and behaviors, making their interactions more meaningful and pertinent.
- Improved customer segmentation: Customer segmentation using different criteria is a strength of machine learning, enabling more accurate targeting. By ensuring that marketing efforts are targeted at the appropriate consumers, improved customer segmentation improves the efficiency of resource allocation and campaign execution. Businesses can design highly targeted advertisements that appeal to particular categories by classifying clients based on demographics, actions, and interests.
- Predictive analytics: Based on historical data, machine learning models can predict future trends and consumer behaviors. Businesses can use predictive analytics to plan marketing tactics, spot prospective business possibilities, and reduce risks. Businesses may make data-driven decisions that provide better results by utilizing machine learning to predict customer attrition, demand changes, and new market trends.
- Optimized ad campaigns: Marketers can fine-tune their advertising campaigns using machine learning algorithms to scan large datasets and detect patterns and trends. As a result, campaigns are created that are timed to reach the correct audience with the right message effectively. As a result, companies should anticipate seeing better returns on their advertising investments (ROI) and increased conversion rates.
- Efficient content creation: Natural language processing (NLP) models are one example of a machine learning-powered technology that may produce content with astounding accuracy. These solutions help mass-produce high-quality, pertinent content, such as product descriptions and blog entries. This saves time and guarantees that brand messaging is consistent across all mediums.
Machine learning in marketing use cases
Although machine learning has numerous use cases, the following are the top machine learning in marketing use cases:
- Chatbots
Most people have come into close contact with machine learning through chatbots at work. These software applications, suitably named, use machine learning and natural language processing (NLP) to mimic human speech. They follow predetermined scripts to interact with people and respond to their inquiries by consulting company databases to offer clarifications.
Early chatbot generations operated according to pre-written rules that directed their behavior in response to keywords. To be more attentive to a user’s demands, more accurate in their responses, and ultimately more humanlike in their interaction, ML enables chatbots to be more engaging and productive.
The chatbots that serve as the first point of contact for most consumer call centers nowadays, including Apple’s Siri and Amazon’s Alexa, are common examples of chatbots.
- Data analysis
It takes more than just staring at a computer screen and waiting for connections to appear to be able to analyze data. The data needs to be prepared beforehand. It must be appropriately formatted, accurately accurate, and organized.
When preparing data for analysis, humans are prone to making mistakes. The information is retrieved from the system of record, “cleaned” following a set of standards to assure accuracy, and formatted appropriately by a machine that doesn’t make mistakes.
Machines also analyze more data than people and do it more quickly. One human can only process so much information simultaneously; machines are not limited in this way. With AI in marketing, machines can now analyze unstructured data, including photographs, videos, audio, social media posts, and other types of information.
- Predictive engagement
Machine learning determines a customer’s identity, where they are in the customer journey, and what actions a marketer can take to assist them in moving on to the next stage. Predictive engagement is when machine learning can tell you the next steps. Analytics and personalized marketing are more closely related than analytics and predictive engagement.
Here’s one example of how predictive analytics may be used: you’ve just begun looking into HR software. Predictive engagement wouldn’t send you a testimonial because you’re just beginning your adventure, and that’s not what you’re interested in. A free demo, which a predictive engagement solution is aware of and offers to you, would really help.
- Personalization at scale
Personalization automation may seem like an oxymoron, but AI in marketing can improve personalization’s effectiveness and efficiency. A computer learns the preferences of its users through machine learning and then adjusts its offers accordingly.
For example, Margo frequents the neighborhood Thai eatery religiously. Margo prefers the shrimp pad, Thai, though she may occasionally try something new. She indicates her preference for the restaurant’s AI-enabled marketing program, which then sends her a voucher for the dish.
- Monitoring and quality assurance
According to Nicolas Avila, CTO for North America at IT services provider Globant, machine learning is beneficial for monitoring needs and quality assurance due to its capacity to recognize and discern patterns in data at a size, pace, and level unmatched by humans.
He gave the use of machine learning to monitor supply chain operations as an illustration, pointing out that the technology continuously analyzes patterns to discover anything that deviates from usual parameters and, thus, may suggest a problem to be addressed.
Neural networks, deep learning, and computer vision are some examples of ML technology types that can be used to more effectively and efficiently monitor manufacturing lines and other workplace outputs to guarantee that products match defined quality requirements.
- Predictive analytics
The first step to better knowing your customers is being able to analyze data. But what if you knew what your clients would do next?
Predictive analytics can help with it. The possibility of a client adopting a specific action is displayed through predictive analytics. Consider Matt buying a piece of software for his workplace. The marketing division of the software provider predicts that he will purchase a complete tech assistance package the following year using predictive analytics.
Machine learning in marketing implementation
Marketing strategies must methodically include machine learning to realize its full potential. The following essential actions will help you use machine learning in marketing efforts:
- Define clear objectives
Setting up clear, precise objectives is essential before beginning the implementation phase. Clearly stated objectives direct the implementation process, whether for bettering consumer segmentation, optimizing ad campaign performance, or anticipating customer behavior.
- Data collection and preparation
Any effective machine learning model is built on top-notch data. Collect pertinent information from various sources, ensuring it’s accurate, comprehensive, and reflective of your target market. To get the data ready for analysis, it may be necessary to clean, transform, and normalize it.
- Select appropriate algorithms
Select the appropriate machine-learning methods based on your clearly defined objectives and the characteristics of your data. For example, regression models can be utilized for predictive analytics, while classification algorithms are suitable for customer segmentation. Try out various algorithms to see which one provides the best fit.
- Model training and validation
You should use a piece of the obtained data to train your machine-learning models and save another amount for validation. This enables you to evaluate the model’s effectiveness and make the required corrections. The model’s ability to generalize to fresh data is ensured through cross-validation.
- Integration with marketing tools
Machine Learning models must be integrated with current marketing platforms and tools for smooth functioning. This ensures that the systems used for customer relationship management (CRM), email marketing, ad platforms, and analytics tools are compatible. It allows for scaled-up individualized interactions and automated decision-making.
- Real-time data processing
Real-time data are essential for machine learning to succeed. Implementing systems that allow for quick data ingestion, processing, and analysis is crucial. Setting up data pipelines, using cloud services, and implementing stream processing technologies are required. The ability to make quick, data-driven decisions for campaign optimization and customer interaction is provided by real-time insights for marketers.
- Testing and iteration
Using machine learning in marketing requires a process of iteration. Starting with small-scale experiments and tests is crucial to fine-tune algorithms and models. This makes it possible for marketers to comprehend how ML affects important metrics and how it may be tweaked for better outcomes. Marketers can improve their ML-powered tactics and realize even greater potential by consistently testing and iterating.
- Compliance and privacy considerations
When utilizing machine learning in marketing, it’s critical to put user privacy and data protection compliance first. Marketers need to ensure that the data used in ML models is gathered and handled ethically and openly.
It is crucial to have strong data security safeguards in place and get users’ informed consent. Compliance protects companies from legal ramifications while fostering customer trust, boosting ML-driven marketing initiatives’ success.
Final thoughts
As you can see, machine learning for marketing analytics is essential in helping marketers and enhancing speed and accuracy that was previously unachievable. The requirement to quickly gather, accurately segment, and instantly translate disparate data into meaningful insights makes custom ML systems challenging to develop. Still, they can also help businesses earn more money.
Please go for professional machine learning development services if you want to use them in your business. With end-to-end machine learning marketing automation and, in particular, the creation of unique ML-based solutions, they can give you access to professionals who delve deeply into your company processes and make them more effective than before.