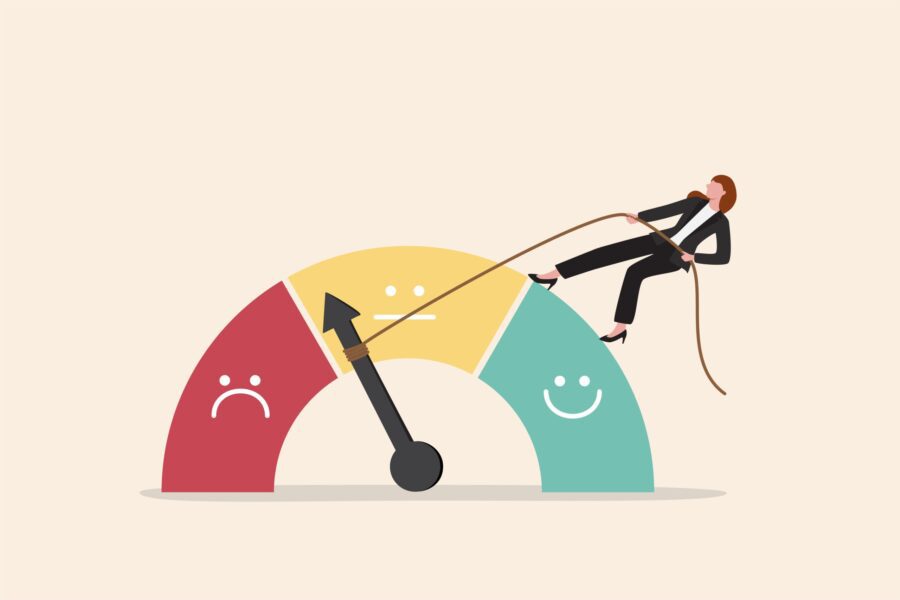
Modern data quality practices leverage advanced technologies, automation, and machine learning to handle diverse data sources, ensure real-time processing, and foster collaboration across stakeholders. They prioritize data governance, continuous monitoring, and proactive management to ensure accurate, reliable, and fit-for-purpose data for informed decision-making and business success.
Modern data quality practices differ from traditional data quality approaches in several ways:
- Data sources and types
Traditional data quality primarily focuses on structured data from internal systems or databases.
Modern data quality practices encompass a wide range of data sources, including unstructured data, external data, social media data, IoT data, and more. The variety of data types and sources has expanded significantly in the modern data landscape. - Scale and volume
With the advent of big data and increased data generation, modern data quality practices address the challenges of processing and managing massive volumes of data. Traditional approaches were not designed to handle such scale, whereas modern practices leverage technologies like distributed processing and cloud computing to manage and analyze large datasets efficiently. - Real-time and near-real-time processing
Traditional data quality processes often operated in batch mode, with periodic data cleansing and validation. Modern data quality emphasizes real-time or near-real-time processing, enabling organizations to detect and address data quality issues as they occur. This is crucial in dynamic environments where data is constantly changing and requires immediate attention. - Automation and machine learning
Modern data quality practices leverage automation and machine learning techniques to enhance data quality processes. Automation enables the efficient execution of repetitive tasks such as data cleansing, validation, and standardization. Machine learning algorithms can learn patterns and anomalies in data, enabling automated detection of data quality issues and predictive data quality management. - Data governance and data stewardship
Modern data quality recognizes the importance of data governance and data stewardship as fundamental components of data quality management. Data governance frameworks establish policies, procedures, and responsibilities for managing data quality throughout the organization. Data stewards are assigned to ensure adherence to these policies and to drive data quality initiatives. - Collaboration and cross-functional involvement
Unlike traditional approaches where data quality was primarily an IT function, modern data quality practices involve collaboration among various stakeholders. This includes business users, data analysts, data scientists, and subject matter experts. Collaboration ensures that data quality requirements are aligned with business needs and that data quality efforts address the specific goals of different departments or projects. - Data quality as a continuous process
Modern data quality practices emphasize the concept of continuous data quality management. Rather than treating data quality as a one-time activity, organizations continuously monitor, measure, and improve data quality. This involves ongoing data profiling, validation, data quality monitoring, and feedback loops to ensure sustained data quality over time.
Overall, modern data quality practices adapt to the changing data landscape, incorporating new data types, handling larger volumes of data, and leveraging automation and advanced analytics. They prioritize real-time processing, collaboration, and continuous improvement to ensure high-quality data that supports informed decision-making and business success. For more information schedule a demo with the DQLabs Expert.