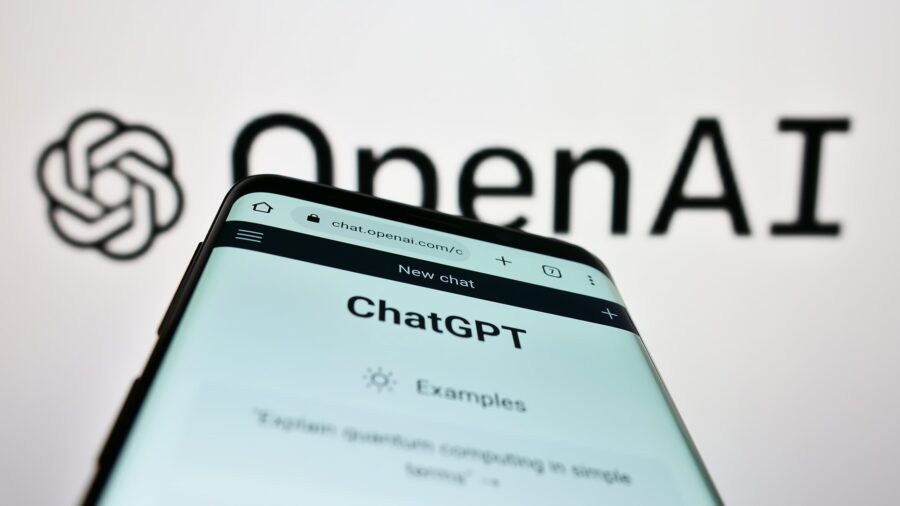
Generative AI has been around for a long time. Some sources say that it appeared as early as the 1950’s. Other sources point to the first rudimentary chatbots that were introduced in the 1960’s. Whatever the true point of origin, we can all agree that those were small pebbles on the historical timeline compared to the huge mountain range of research papers, applications, news articles, blog articles, and conversations occurring in the past year, particularly with generative AI’s appearance both in computer vision models (deep learning for images and videos, including stable diffusion, Midjourney, and DALL·E) and in large language models (deep learning for text and language, including GPT-3, GPT-4, and the preeminent example mentioned in the title of this article).
Generative AI is a field of artificial intelligence (AI) that focuses on the training and deployment of systems capable of generating new and original content, such as creating novel text, images, music, or video from historical training examples of such content types. While this can be applied to structured data (like data tables, time series, and databases), it has proven to be groundbreaking and globally newsworthy when applied to unstructured data (images and text). Unlike traditional AI models that rely on predefined rules and patterns, generative AI models have the ability to produce novel outputs by learning from vast amounts of prior data.
At the core of generative AI are concepts from machine learning (ML) and statistics. (Of course, statistical learning and machine learning are closely related already.)
With regard to the specific aspects of ML that appear in generative AI, a subset of ML called unsupervised learning is used to learn recurring patterns and structures within a given data set. These patterns then become “building blocks with statistical superpowers” (pardon my hyperbole), which can then be combined in logically meaningful and statistically likely groupings to generate new content (often, impressively new content) that closely resembles the training data: text or images. This process is unsupervised learning because it is not aimed at classifying or labeling or reproducing known patterns (supervised learning), but it is aimed at complex pattern discovery in unstructured data (sort of like a general form of independent component analysis ICA, which is similar to but not the same as principal component analysis PCA). ICA is used in signal processing (e.g., in blind source separation or “the cocktail party problem”) – it is a computational method used in identifying and separating a complex signal into a set of additive independent subcomponents.
With regard to the aspects of statistics that appear in generative AI, we encounter many of the key statistical concepts that underlie Markov models and Bayesian learning (hence, the origins of generative AI in the 1950’s). Conditional probabilities, which power those methods, go much further back in history, most prominently of course to the Reverend Thomas Bayes (Bayes Theorem published in 1763). Using conditional probabilities on colossally complex and massively multivariate data, the most likely combination of those building blocks (patterns and structures learned by the unsupervised ML) is calculated by the generative AI in response to the user’s query (i.e., user prompt).
The “secret sauce” in generative AI’s ability to build novel outputs is thus comprised of three basic structures: (1) the storehouse of all possible ingredients (i.e., the ML-learned patterns and structures in the training data); (2) the user’s intent (i.e., personalized request, from a vast menu of choices, provided in the user query, which is the prompt that specifies what the user wants); and (3) the recipe (i.e., the statistical model that computes which combination of ingredients, and in which order, will generate the output that is most statistically likely to satisfy the user query). Please excuse my use of a food metaphor here, but I have my reasons (see below).
To add a bit more color here, the “context” of the query is also fundamentally important, but I intended for that “personalization” component of generative AI to be represented already in the prompt that specifies the user’s intent. Getting the best (most informative, satisfying, and personalized) response is strongly dependent on providing good context within good prompt engineering, which is rising as a new “future of work” workplace skill.
At this point (while writing this), I decided to instantiate my food metaphor with ChatGPT. So, I prompted ChatGPT with this query: “Give me a recipe for a pie that uses regional fruits and spices for a person living in Hawaii.” Here is the response: “Kirk Borne asks ChatGPT for a Hawaiian Pie.” [REF 1] I need to wrap up this blog and go make a pie now.
While all this is exciting, enticing, exhilarating, and explosively transformative, we must also be educational. What I mean is this: before business executives and other leaders get a case of FOMO and say, “give me some of that generative AI right now”, for fear of falling behind their competitors and the rest of the market, there needs to be a foundation for any such deployment to be successful and productive within the enterprise. What are some of the key ingredients in that recipe? [REF 2] Here are three:
- Data Literacy – the people need to understand data and how data provides business insights and value; what are the types of data that exist in the enterprise; where do these data reside; who is using these data; for what business purposes are the data being used; what are the ethical (governance and legal) requirements on the access and use of these data; and ultimately are these data sufficiently ready to be used in training generative AI (large language and/or vision models)?
- Data Quality – do we really need to say it? Okay, I will say it: GIGO “garbage in; garbage out!” Dirty data is far more dangerous in black-box ML models, particularly those models that consume massive quantities of data (e.g., deep learning, AI, and generative AI). Model explainability means nothing if the data is dirty, and model trustworthiness is lost.
- Data/ML Engineering Infrastructure – there is a huge difference between an exploratory ML model running on a data scientist’s laptop versus a deployed, validated, governed, and enterprise-wide model running across the whole business, on which the business is placing a big bet and a ton of dependence. The infrastructure must be AI-ready, and that includes the network, storage, and computational infrastructure. Without this resilient foundation, the ML model running on the CEO’s laptop at a board meeting is probably a better choice than the generative AI “demo demons” showing up at the worst possible time.
So, do you now think that this article was about ChatGPT? I guess it really was. Maybe. [REF 3]
REFERENCES:
- “Kirk Borne asks ChatGPT for a Hawaiian Pie” at https://bit.ly/3pvAzMF
- Source for meme: https://bit.ly/44lgMhI
- The inspiration for the article’s title: https://www.youtube.com/watch?v=mQZmCJUSC6g
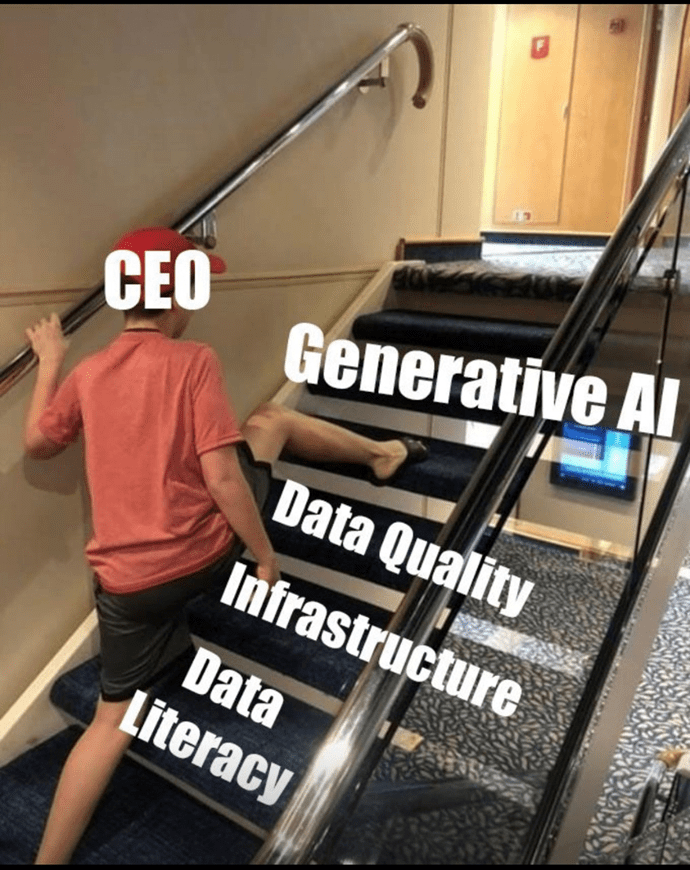