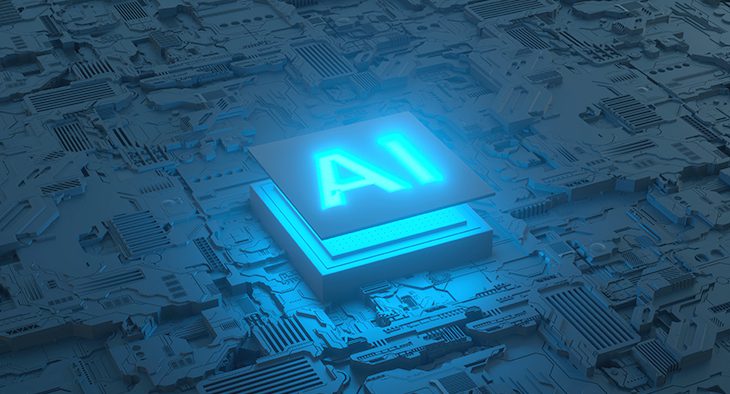
Introduction
In recent years technology has become prominent, both at work and at home. Machine learning (ML) and Artificial Intelligence (AI) are evolving quickly today. Almost everyone will have some interaction with a form of AI daily. Some common examples include Siri, Google Maps, Netflix, and Social media (Facebook/Snapchat).AI and ML have popularly used buzzwords right now, often used interchangeably. Most experimentation has been geared to finding specific solutions to specific problems. Artificial Intelligence (AI) is an application in which a machine can perform human-like tasks. At the same time, Machine Learning (ML) is a system that can automatically learn and improve from experience without being directly programmed.
Data quality refers to how relevant information is for use. If the information isn’t suitable, you won’t be able to make the right decisions. Data quality is determined by several factors, including; accuracy, completeness, reliability, relevance, and timeliness. If there’s a missing factor or is lower than other factors, your data quality won’t be very high. Read more about what is data quality and why is it important.
Increased data volumes have put companies under pressure to manage and control their data assets systematically. Also, standard data management practices lack sufficient scalability and cannot manage ever-increasing data volumes. Companies, therefore, need to rethink their data management. The good news is that substantial progress in artificial intelligence (AI) and machine learning (ML) through entities such as DQLabs.ai – AI/ML augmented data quality management platform, can support you in your data management activities.
How has AI and ML transformed quality management?
Automatic data capture
Besides data predictions, AI helps improve data quality by automating data entry through executing intelligent capture. This ensures all the valuable information is captured, and there are no gaps in the system.
Recognize duplicate records
Twofold entries of data can lead to outdated records that result in bad data quality. AI helps eliminate duplicate records in an organization’s database and keeps precise gold keys in the database. It is hard to identify and remove recurring entries in a big company’s repository without implementing sophisticated mechanisms. An organization can combat this by having intelligent systems that can detect and remove duplicate keys.
Detect anomalies
A small human mistake can drastically affect the utility and the quality of data in a CRM. An AI-enabled system removes defects in a system. Data quality can also be enhanced through the implementation of machine learning-based anomalies.
Third-party data inclusion
Apart from correcting and maintaining data integrity, AI can improve data quality by adding to it. Third-party organizations and governmental units can significantly add value to the quality of a management system and MDM platforms by presenting better and more complete data, contributing to precise decision-making. AI makes suggestions on what to fetch from a particular set of data and the building connections in the data. When a company has detailed and clean data in one place, it has a higher chance of making informed decisions.
Fill data gaps
While many automation systems can cleanse data based on explicit programming rules, it’s almost impossible for them to fill in missing data gaps without manual intervention or plugging in additional data source feeds. However, machine learning can make calculated assessments on missing data based on its reading of the situation.
Assess relevance
On the other end of the scope of missing data, organizations often accumulate a large amount of redundant data over the years that do not have any use in a business context. Using machine learning, the system can self-teach on the data points required and those not needed. Analysis of this kind can help revamp the process and, eventually, make it simpler.
Match and validate data
Coming up with rules to match data collected from various sources can be a time-consuming process. As the number of births increases, this becomes increasingly more challenging. ML models can be trained to learn the rules and predict matches for new data. There is no restriction to the volume of data, and as a matter of fact, more data works favorably in fine-tuning the model.
The cost of bad data
Bad data can prove to be quite expensive for companies. Attempts to quantify the financial impact have resulted in some shocking numbers. It’s also important to remember that decisions based on flawed data can lead to severe consequences in some cases. Machine learning algorithms can flag some of these situations before they get too far. Financial companies use them to identify forged transactions. It’s estimated that ML models can result in a $12 billion in savings for card issuers and banks.
Conclusion
Most businesses look for fast analytics with high-quality insights to deliver real-time benefits based on fast decisions. They consider this a high priority and means of competitive advantage. To enable this, there is an opportunity for organizations to fine-tune and enhance the current data quality approach using ML techniques. Many leading data quality tools and solution providers have tried out ML territory with the expectation of increasing the effectiveness of their solutions. Thus, it has the chance of being a game-changer for businesses in pursuit of improved data quality. Although the current intake level of the use of ML for data quality assessment and enhancement is low, it has promising prospects to churn large data sets and enhance data quality.
If you want to try an AI and ML-based data quality tool to automate all your DQ management, request DQLabs platform demo here.