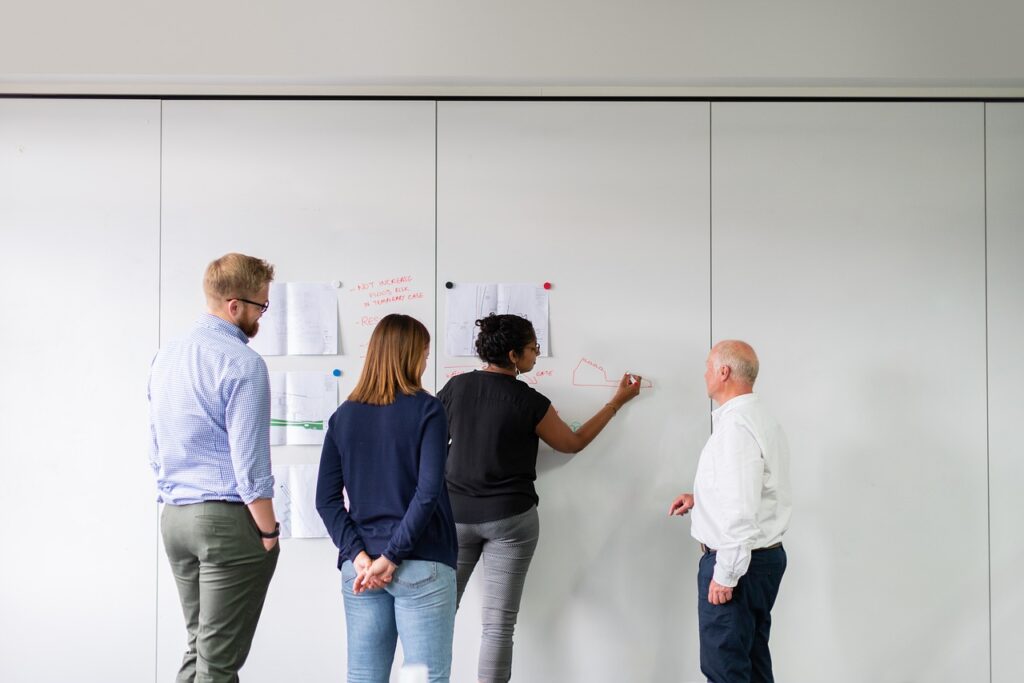
Image by This_is_Engineering from Pixabay
Are you friends with a big-company executive who has clout? Maybe they manage a big AI program, or they head up a line of business or department.
Ideally, they have carte blanche to make substantial changes in how their organization is structured and run.
And they can obtain buy-in from their peers on how to innovate at scale.
And they understand the transformative value of all data–not just the kind that’s in tables, or the kind the company knows how to collect and manage right now.
For the sake of argument, let’s assume you know such a person. Here’s how to help that person build a foundation for an AI-enabling data empire for less than what the company spends on data management today.
Step One: Stop the bleeding
According to dataware company Cinchy, half the average enterprise’s IT budget is spent on integration. That’s clearly a ridiculous and unsustainable situation, one that underscores how misallocated IT resources can become when leadership doesn’t understand the nature of the integration problem or how to solve it, at the data layer, with a graph-managed semantic approach.
How do you change that situation? Commit to a transformed data architecture that harnesses logical graph connectivity. As I’ve pointed out before, thousands of databases, each with its own data model, imply pervasive siloing and a lack of transparency. Thus the continually rising integration cost. Out-of-control SaaS subscriptions only add to the problem, as I described in this post: https://www.datasciencecentral.com/a-12-step-fair-data-fabric-program-for-recovering-application-addicts/
Ideally, your company’s CFO will gravitate to this complexity-is-spiraling-out-of-control argument and back your effort to desilo the data resources you’ll need for your company’s AI phase.
Desiloing via a knowledge graph approach is the only feasible way to stop the technical debt and complexity accumulation of hundreds or thousands of underutilized applications that are trapping your data and logic resources, driving up integration costs in the process. You need that budget to fund your own substantive, system-level data transformation efforts.
To be valid, a data architecture transformation program needs to develop self-describing and self-healing information resources based on a unitary, extensible, standards-based graph data model.
Make sure leadership understands what’s wrong with IT as it’s currently constituted and how to fix it. My previous post pre- and post- data transformation states: https://www.datasciencecentral.com/boosting-innovation-initiatives-with-knowledge-graphs/
Step Two: Envision the how to build a foundation for the future data empire
The future state isn’t having separate data management, knowledge management and content management departments, or business units that are disconnected from the data harmonization effort. That’s the present state of affairs.
Empower all the people in these departments by helping them use the same system to manage information. Help them to work visually, collaborating with the help of whiteboards that can articulate the current and future states of data architecture and the related parts of the organizationo. Help them make that information shareable. Eliminate the redundancy of managing separate or disconnected activities.
All these people could be doing things with data the same way. We have mature semantic graph data (and metadata) standards. The semantics community has thankfully brought the system together to harness the power of these standards. Particularly key is unique global identifiers.
Engage the businesspeople who can make organizational change possible. Help them understand you can have one just department. You don’t need three or four departments. That’s not a modern, graph-based approach to managing shareable meaning at scale. In information technology, the more resources are unified, the greater the efficiency. That’s an argument for meta-organization and organizational boundary-crossing capabilities as well.
Step Three: Work toward a shared network of FAIR digital twins
Digital twins can provide an intentional design focus at a helpful level of abstraction. First it helps to more fully articulate what proper digital twins are. When some people think of digital twins, they’re not thinking about the back end–the self-describing and connecting data and knowledge foundation that’s required to make digital twins interactive and interoperable.
Going to different conferences on different technology topics as an emerging tech research analyst gave me a system of level perspective of what was happening. At a virtual/augmented reality (VR/AR) conferences back in 2019, they had two people on stage talking about the topic. One of them was Kevin Kelly, a co-founder of Wired magazine. He’d written many books over the years. In early 2019, he had written an article for Wired entitled “AR Will Spark the Next Big Tech Platform—Call It Mirrorworld.”
In Mirrorworld terms, there is the physical world and then the represented world.The represented world is the digital mirror of the physical world. In addition, there are virtual elements that you can create as a part of that same “mirror”, to help humans can work together and work with machines via the whole represented ecosystem in certain ways.
Augmented reality and virtual reality put all the attention on the front end. So what Kevin Kelly wasn’t really addressing was all the back end that has to be done before you can have interactive, interoperable digital twins.
Which leads to another useful term–FAIR data. FAIR stands for findable, accessible, interoperable and reusable. In a supply chain scenario, for example, it’s essential to share a lot of information in the right way to the right people at the right time – a classic distribution problem, really. A lot of the reason supply chains have bottlenecks right now is because there’s insufficient sharing happening. The sharing is not timely enough, relevant or accurate enough.
To optimize supply chains, you want every twin to be FAIR so it can be interoperable with other twins. That’s the only way you get to the kind of Mirrorworld Kelly envisions. All the VR goggles, AR glasses and multidimensional presentation don’t matter if you can’t share data sufficiently so that your twins interact interoperably.
Beyond the bright and shiny objects
The big unmet challenge for advanced graph data management advocates is that the bright and shiny objects such as AR contact lenses and generative AI are what capture the attention of most leadership. You’ll need a friendly, influential executive who can tell a budget story about how the self-describing data/knowledge graph backend can pay for itself. So just maybe the CFO and others in the decision loop will see the sense of the argument laid out above.