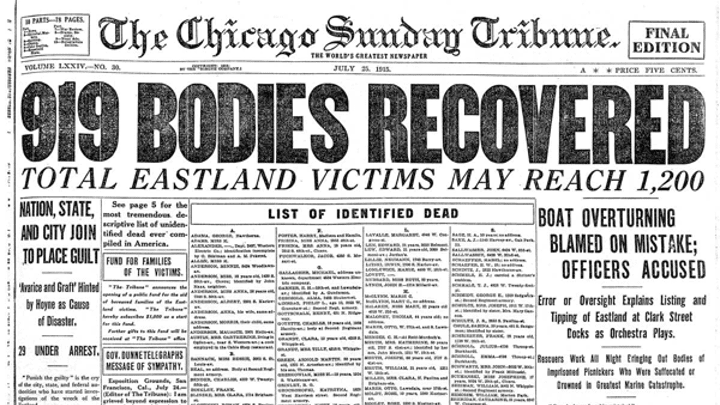
This is part 3 of a three-part series on the Economics of Ethics. In Part I of the Economics of Ethic series, we talked about economics as a framework for the creation and distribution of society value. In Part II, we discussed the difference between financial and economic measures, the role of laws and regulations in an attempt to establish moral or ethical codes, and the potential ramifications of the recently released “AI Bill of Rights” from the White House Office of Science and Technology Policy (OSTP).
In part 3 we are going to focus on brainstorming and minimizing the impact of unintended consequences of policy and operational decisions. I will also introduce the “Economics of Ethics” worksheet at the end of the blog to formalize the process of identifying the variables and metrics that we can use to not only measure ethical behaviors but also help to minimize the consequences of unintended consequences.
Here’s the problem with the data and AI ethics conversation – if we can’t measure it, then we can’t monitor it, judge it, or change it. We must find a way to transparently instrument and measure ethics. And that’ll become even more important as AI becomes more infused into the fabric of societal decisions around employment, credit, financing, housing, healthcare, education, taxes, law enforcement, legal representation, digital marketing and social media, news and content distribution, and more. We must solve this AI codification and measurement challenge today and that is the objective of the “Economics of Ethics” blog series
Contemplating and Minimizing Unintended Consequences
Unintended consequences are unforeseen or unintended results that can occur as a result of an action or decision.
Unintended consequences can occur when not enough careful consideration is given to the wide and diverse range of potential outcomes such Best Case, Most Likely Case, and Worst Case outcomes that we will be considering later in a worksheet exercise.
There are many examples of “good intentions gone wrong” yielding unintended consequences such as:
- The SS Eastland, a badly-designed, ungainly vessel, was intended to be made safer by adding several lifeboats. Unfortunately, the extra weight of the lifeboats caused the ship to capsize, thereby trapping and killing 800 passengers below the decks.
- The Treaty of Versailles dictated surrender terms to Germany to end World War I. Unfortunately, the terms empowered Adolf Hitler and his followers, leading to World War II.
- The Smokey Bear Wildfire Prevention campaign created decades of highly successful fire prevention. Unfortunately, this disrupted normal fire process that are vital to the health of the forest. The result is megafires that destroy everything in their path, even huge pine trees which had stood for several thousand years through normal fire conditions.
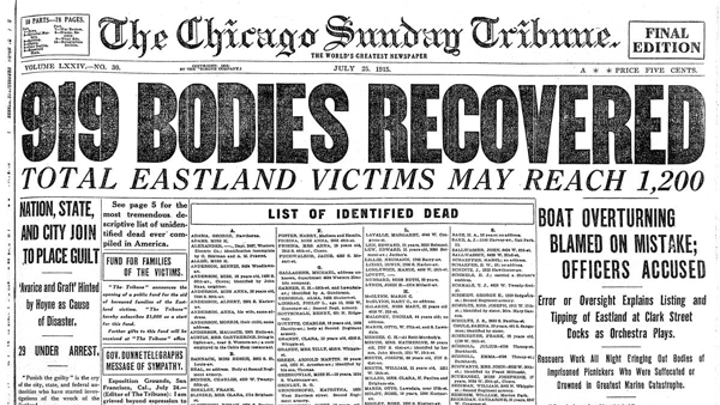
Figure 1: SS Eastland Disaster Kills 884 Passengers and Crew
There are a several actions that organizations can take to scrutinize and minimize the potential impacts of those unintended consequences:
- Train everyone on a formal process that ensures that the organization invests the time and effort to envision the potential consequences of the decision *before* the decision is made. This should be a multi-discipline, collaborative envisioning, and exploration process where all ideas from all stakeholders are worthy of consideration. And yes, this process might take a while (talking days or weeks, not months or years).
- Engage a broad range of stakeholders who bring different perspectives and experiences to brainstorm these potential unintended consequences. Those stakeholders should include internal and external parties that either impact or are impacted by the decision; that is, stakeholders how have a vested interest in doing the right thing.
- Identify the KPIs and metrics against which the effectiveness of the decision will be measured. The KPIs and metrics should cover a diverse range of “value” dimensions including financial, operational, stakeholder, partner, employee, environmental, and societal. Also, identify KPIs and metrics from both a short-term (2 years or less) and long-term (2 years plus) perspectives. Do not shortchange this step as these KPIs and metrics will come into play as you build out your AI / ML models!
- Ensure that the decision-making process is properly instrumented to continuously monitor the process, identify any performance anomalies, trends, and patterns, and make necessary adjustments as quickly as possible.
- Create supporting worksheets, check lists, and design templates to guide the brainstorming, monitoring, and measuring of potential unintended consequences process.
- Establish formal and regular review decision-making checkpoints to capture process learnings and analyze outcomes effectiveness to help avoid similar unintended consequences in future decisions.
One can leverage the processes, design templates, and checklists that are already part of the “Thinking Like a Data Scientist” (TLADS) methodology to help brainstorm and envision potential unintended consequences (Figure 2).
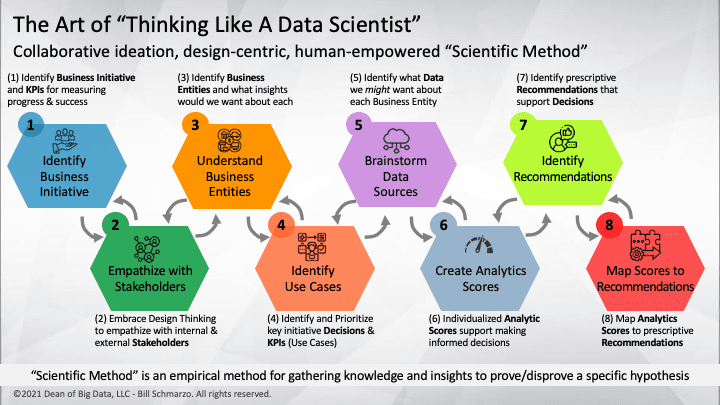
Figure 2: The Art of Thinking Like a Data Scientist
Note: The Thinking Like a Data Scientist methodology seeks to drive business and technology collaboration and synergy around defining how “value” is created and measured, engaging the business and operational stakeholders in identifying, validating, valuing, and prioritizing the decisions that they need to make, ideating the features that might be better predictors of performance, and establishing a feedback loop that supports the continuous learning and adapting business and operational outcomes process
The challenge for trying to codify ethics into laws and regulations is the lag effect that can occur when rapid technology and cultural advancements almost negate the laws before they have a chance to change behaviors. But when actions are taken, they can have huge impact (both negatively and positively). Let’s take a look at a recent effort to codify ethics with respect to how your personal data is used by AI models – the “AI Bill of Rights.”
So, how might an organization go about the process of brainstorming the potential unintended consequences of ethical decisions? Let’s see if we can create a simple worksheet to contemplate and codify the economic ramifications of ethical decisions.
Economics of Ethics Design Canvas
To start gaining feedback and alignment around how we can transparently instrument and measure ethics, I’m proposing a collaborative Ethics Design Canvas that organizations can use to make the codifying of ethics conversation actionable. This canvas is designed to leverage economics concepts around value creation to facilitate the difficult conversation across the organization’s key internal and external stakeholders in identifying how “ethics” will be measured and monitored for a particular initiative or program.
Plus, this ethics design canvas is a nice extension to well-documented and tested “Thinking Like a Data Scientist” methodology including the design canvases already associated with the methodology. And as we learned with the design canvases in the TLADS methodology, it will evolve as we use it and learn what works and what doesn’t work (Figure 3).
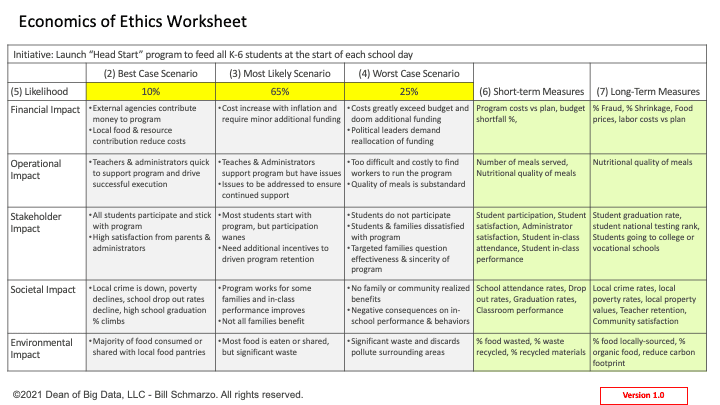
Figure 3: Economics of Ethics Design Canvas
Here are some instructions for the Economics of Ethics Design Canvas:
- Panel 1: State the situation, decision, or program under evaluation
- Panels 2 – 4: Collaboratively brainstorm potential outcomes across the dimensions of financial, operational, stakeholder, societal, and environment across the Best Case, Most Likely Case, and Worst Case scenarios
- Panel 5: Determine the likelihood or probability (%) of the three different scenarios. Note: one can and should have a follow up exercise to brainstorm, capture, and validate the variables that influence Best Case versus Worst Case scenarios.
- Panel 6: From the perspective of the short-term (2 years or less), brainstorm the measures against which one would measure the outcomes impact across the dimensions of financial, operational, stakeholder, societal, and environment. More variables are better than fewer variables.
- Panel 7: From the perspective of the long-term (2 years and more), brainstorm the measures against which one would measure the outcomes impact across the dimensions of financial, operational, stakeholder, societal, and environment.
Since there should be several measures identified during this exercise, it would be useful to weigh the relative importance of each of the measures from either High / Medium / Low or on a scale of 1 to 5.
Note: Many of the measures in the short-term are lagging indicators and many of the measures in the long-term are leading indicators. Leading indicators are useful for predicting outcomes (measures that influence the outcomes), while lagging indicators are useful for measuring outcomes (indicators that measure the outcomes).
“Economics of Ethics” Blog Series Summary
“You are what you measure and you measure what you reward”
What would be the ramifications to society if we actually rewarded (paid) our leaders on the metrics that really mattered the most? How would that change the laws and regulations that got developed, the actions that organizations took, and the decisions that government and social agencies made?
Here’s an interesting point to contemplate: while we can go through these extensive, inclusive, collaborative steps around a value creation framework (economics) to try to create AI models that perform ethically, what are we doing to solve the bigger problem of creating incentive systems to get humans to act ethically?
That one is on all of us…