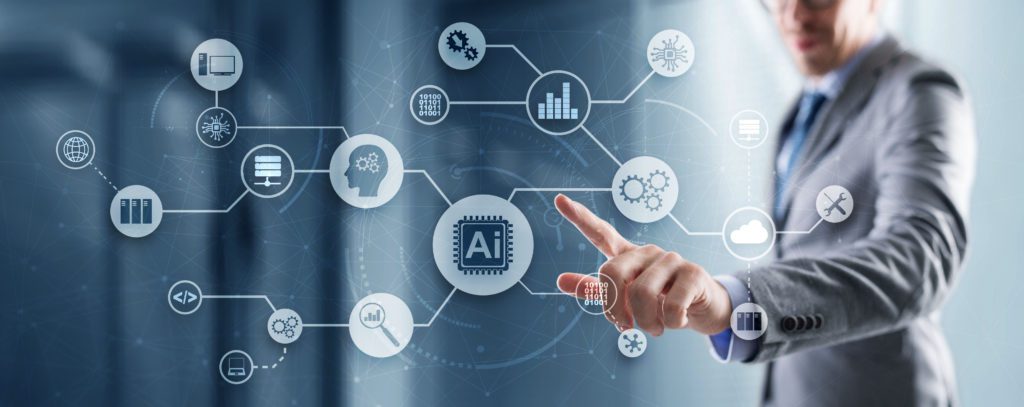
Recent advances in machine learning (ML) and artificial intelligence (AI) technologies are helping enterprises across industries quickly move from their use cases from the pilot stage to production and operationalization. According to a report by McKinsey & Company, by 2030, businesses that fully absorb AI could double their cash flow, while companies that don’t could see a 20% decline*. As market pressures increase, data leaders must move beyond point solutions and assess their entire data science and ML ecosystem when considering new ways to leverage technology and reduce time to market. While the number of available ML frameworks has exploded, developing models remains a complex task involving data acquisition, pre-processing, feature selection, modelling, testing, tuning, deployment, etc. Data science teams need a unified platform that encompasses the complete ML lifecycle, fosters collaboration, and centralizes all data science projects in a secure repository.
Drag-and-drop pipelining to accelerate the ML lifecycle
ML innovation is no longer limited only to specialists with niche expertise. With advanced zero-code data pipeline tools, users can use a visual drag-and-drop UI to easily build and deploy models, irrespective of skillsets. Here’s a closer look at some of the powerful capabilities such tools offer:
• Data acquisition: Ability to connect to a wide variety of batch and streaming data sources for data wrangling in a few clicks. Users can perform data quality checks, identify outliers, and enrich incoming data using self-service operators. This helps build models with accuracy.
• Data preparation and feature engineering: An intuitive, wizard-based UI to handle feature selection and complex pre-processing steps like imputation, binning, one-hot encoding, scaling, etc. Users can scrub missing values, edit columns with proper names, enrich data by adding new sources, mask PII data, and eliminate outliers.
• Training, testing, and tuning: Support for multiple algorithms for classification, clustering, and regression analysis. Users can drag and drop an algorithm and train it using an interactive UI. They can also systematically tune hyper-parameters.
• Scoring: Ability to build streaming pipelines to score models in real-time and choose the best one for deployment from a list of trained models.
• Versioning: A powerful visual versioning and retraining mechanism that allows users to easily roll back to a previous version
• Post-production monitoring: Simplified A/B testing to monitor model performance in the production environment. Users can swap the best-performing models based on real-time performance or accuracy using Champion/Challenger and Hot Swap techniques.
• MLOps: Robust MLOps capabilities to create and deploy models
at scale using automated and reproducible machine learning workflows.
• Model-as-a-service: Standalone data science models can be migrated to an application (exposed as a REST service) for use across enterprise teams and use cases.
Data pipeline tools with in-built notebooks and integrations provide an added advantage, as data professionals can easily sync and manage all datasets, notebooks, models, workflows, tags, and versions of their work with GIT. Additionally, drag-and-drop tools based on open-source technologies provide flexibility by enabling users to import models from platforms like TensorFlow. Many also offer role-based access and other stringent security features to protect data and model confidentiality and integrity.
Democratizing machine learning
Till a few years ago, only tech giants had access to the massive datasets and computing resources needed to train sophisticated AI algorithms and deploy machine learning models at scale. Most small and medium-sized enterprises were not able to get their AI use cases off the ground. The emergence of drag-and-drop ML tools with simple graphical interfaces means that a broader range of data and analytics users can develop and operationalize ML models for a larger number of use cases. Such tools make ML experts more efficient, while empowering anyone working with data to incorporate ML capabilities into their use activities. As a result, enterprises can seamlessly experiment with new packages and languages, reduce duplication of effort, and achieve faster time-to-market.
*Source: https://www.mckinsey.com/featured-insights/artificial-intelligence/notes-from-the-ai-frontier-modeling-the-impact-of-ai-on-the-world-economy