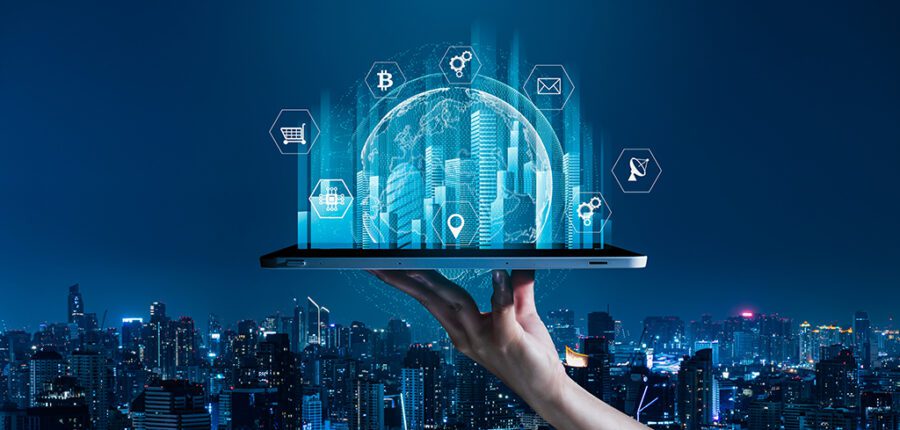
I was conducting a Tech Talk at a client when I mentioned the astronomical growth of data at “the edge”; that data creation at the edge is growing almost as fast as that in the cloud according to IDC.
This sent a noticeable ripple across the executive team audience. The executives immediately began debating “How are we going to manage all of that data at the edge?” Suddenly, one executive said “Wait, the bigger question is how are we going to monetize all that data at the edge?” Ah yes, I had found my sponsor!
The IDC report “The Digitization of the World: From Edge to Core” forecasts that 175 zettabytes of data will be generated by 2025. And of those 175 zettabytes, over 90 zettabytes of that data will be generated at the edge (Figure 1).
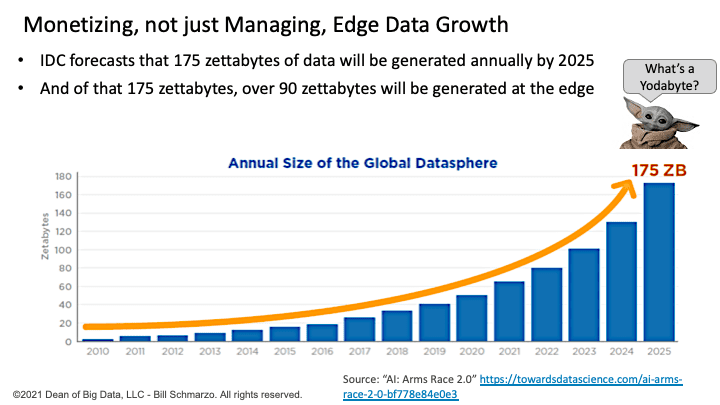
Figure 1: Source: “AI: Arms Race 2.0”
Today, approximately 80% of data is being processed at a centralized location and 20% at Edge. But by 2025, those numbers are projected to reverse! And in the case, like my sponsor stated, organizations must learn how to master monetizing – or driving and deriving new sources of customer, product, service, and operational value – from this growing volume of edge data.
What is “The Edge”?
The “Edge” is defined the point in the infrastructure where sensors and devices communicate real-time data to a network. But the edge is more than just collecting new sources of data. The edge “secret sauce” is its ability to ingest, analyze, and act upon data at the edges in real or near real-time. These new edge capabilities are enabling new business and operational use cases – everything from smart places to predictive maintenance to robotic process automation RPA) to real-time customer promotional activities (Figure 2).
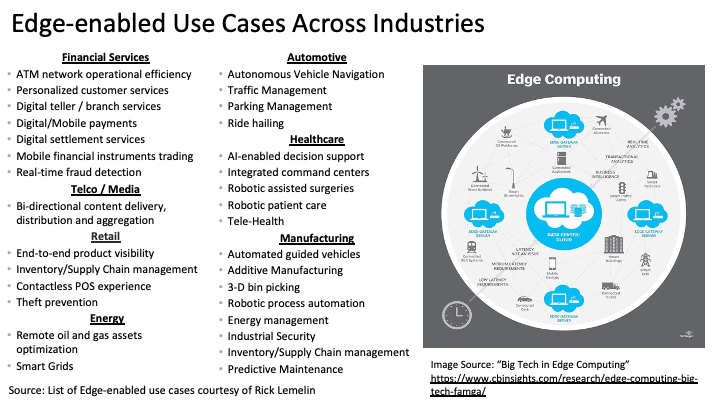
Figure 2: Edge-enabled Use Cases Across Industries
Let’s review a use case showing the power of the edge in action, and how the edge accentuates the organization’s customer, product, service, and operational value creation capabilities.
Smart Airport Case Study
Creating a smart entity starts by understanding the objectives of the smart entity and the KPIs against which the effectiveness of that smart entity will be measured. Those objectives can be broken down into decisions that the key smart entity stakeholders need to make (using the “Thinking Like a Data Scientist” methodology), and then grouping those decisions and KPIs into common business and operational use cases (see Figure 3 for the smart airport use cases).
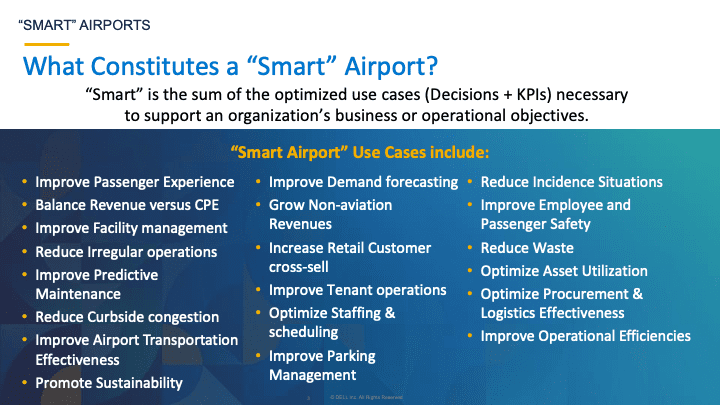
Figure 3: Potential Smart Airport Use Cases
Because the edge enables the capture of data AND the real-time analysis of that data AND actions at the edge based upon that analysis, we must factor the latency or timeliness of the decisions to ensure that we have created the most appropriate data and analytics architecture.
We need to map the decisions across the Edge-Core-Cloud architecture to understand the decision timing considerations – real-time or near real-time versus intra-day or daily versus weekly or monthly – that guides the optimal data and analytics architecture (Figure 4).
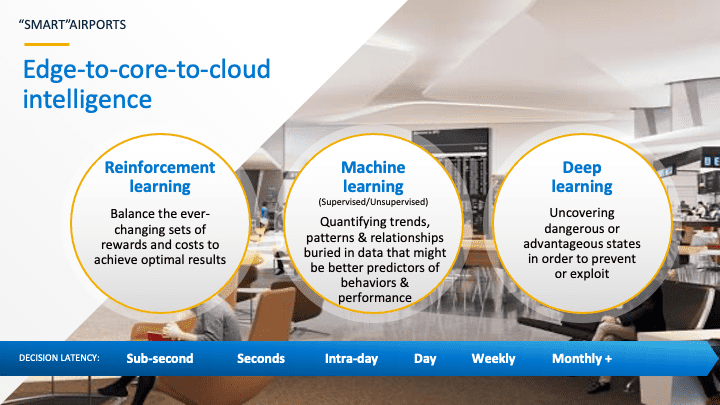
Figure 4: Edge-to-Core-to-Cloud Intelligence
Decision-making at the Edge
The ability to ingest, infer, analyze, and act on data at the edge exploits edge-specific advanced analytic capabilities. There seems to be three prevalent advanced analytic capabilities for making decisions at the edge: Federated learning versus swarm learning (Figure 5):
- Reinforcement learning employs a trial-and-error approach to map situations to actions to maximize rewards. Actions may affect immediate rewards, but actions may also affect subsequent or longer-term rewards, so the full extent of rewards must be considered when evaluating the reinforcement learning effectiveness. The children’s game of “Hotter or Colder” is a good illustration of reinforcement learning; rather than getting a specific “right or wrong” answer with each data action, you’ll get a delayed reaction and only a hint of whether you’re heading in the right direction (hotter or colder).
- Federated Learning requires a central server that trains an algorithm across multiple decentralized edge devices using local data samples. The training data remains on your remote device. Federated Learning works like this: the remote device downloads the current analytic model from a central repository, improves it by learning from data on the remote or edge device, and then summarizes the changes as a small, focused update that is sent to the cloud where it is aggregated with other updates to improve the analytic model.
- Swarm Learning is a decentralized machine learning framework that enables organizations to use distributed data to build ML models by leveraging blockchain technology. Swarm Learning facilitates the sharing of insights captured from the data rather than the raw data itself. Swarm Learning unites edge computing, blockchain-based peer-to-peer networking and coordination while maintaining confidentiality without the need for a central coordinator, which can be seen as a limitation of Federated Learning.
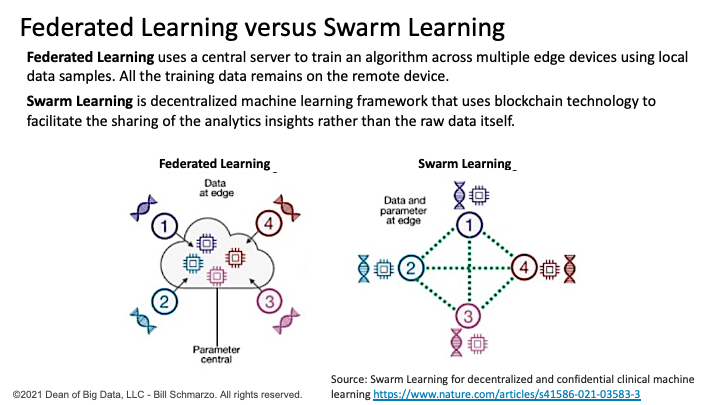
Figure 6: Federated Learning versus Swarm Learning
Summary: Keys to Monetizing the Edge
The edge enables entire new families of business and operational use cases. The bigger business opportunity is the edge’s ability to also infer, analyze, predict, and act upon all that data at the edge in real-time or near real-time (Figure 6).
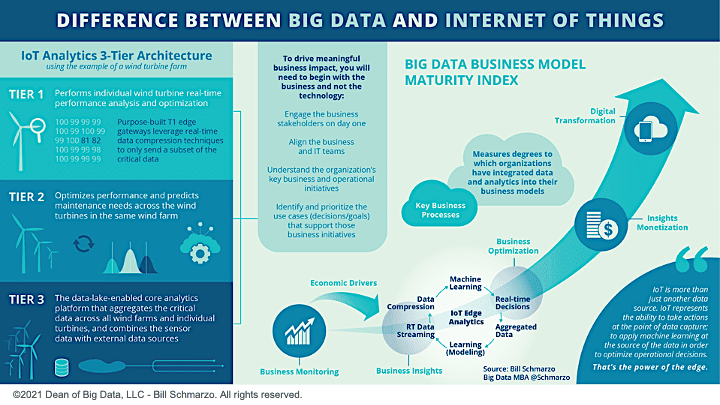
Figure 6: Difference Between Big Data and Internet of Things
The Edge provide new ways for organizations to create new sources of customer, product, service, and operational value. The Edge provides a new value creation dimension for organizations seeking to optimize their key business and operational processes, mitigate compliance and operational risk, uncover new monetization opportunities, and create a more compelling, differentiated customers experience.
But as in all conversations about data and analytics, the conversations must start by understanding where and how the organization creates value (those customer, product, service, and operational use cases) and the KPIs and metrics against which that value creation effectiveness is measured.
If you start your edge journey there, then the edge opportunities are nearly boundless…