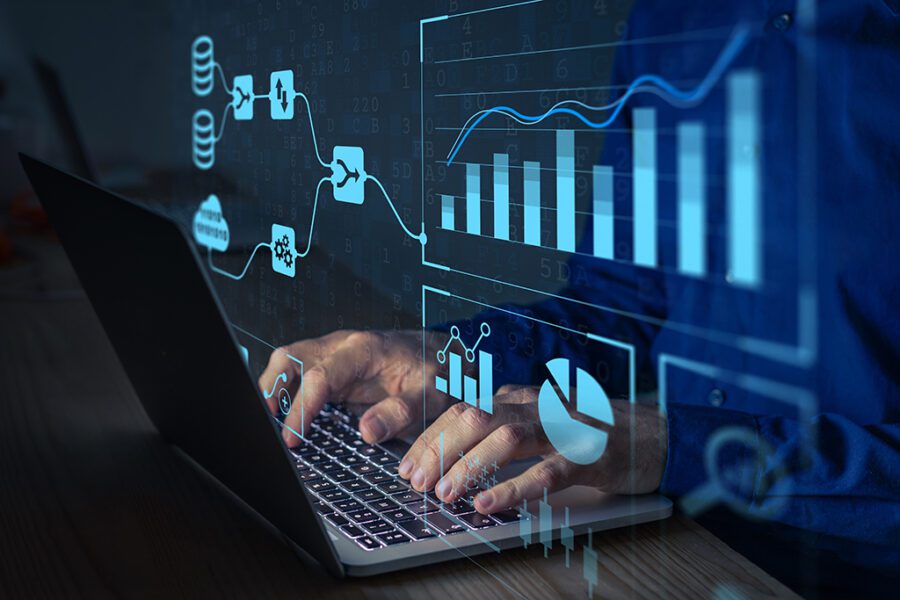
Not surprisingly, digital transformation is a prerequisite for forward-thinking businesses. The catastrophic disruption of the global pandemic did not slow down the need for systems, processes, and people who will help modern organizations move faster. Data, as always, is top of mind.
With so many trends and tools available, it can be hard to see the forest for the trees. What the pandemic has done, for many, is highlight a need to future-proof their data environment from future disruptions.
With more data flowing into businesses and a greater need to automate processes and maximize impact, these are the data trends that will define 2022.
The need for external data will drive increased adoption of data catalogs
There are a few major data catalog providers, and increasingly their platforms are touted as a necessary component of a modern tech stack. Data-driven organizations (or those that aspire to be) are looking for solutions that let them discover, manage metadata, and supervise access from a single control panel. What’s unclear, however, is how organizations are managing the flow of external data into their centralized catalog environment.
External data is a clear differentiator. This became clear during the Covid-19 pandemic, where organizations realized overnight that existing models were rendered obsolete by the rapid shift in markets, so forecasting changes or understanding short-term economic outlook became effectively impossible without the addition of new data that augmented existing or outdated models. Organizations turned to external data sources like Google’s Mobility Reports, the World Health Organization’s Situation Reports, and even Twitter or search traffic to gain insight into the rapidly shifting landscape.
While any organization with a decent data team can spin up a few people to connect to this data, managing its flow over time requires a different set of tools than those available in a traditional data catalog. As Bernard Marr noted recently in Forbes, “there are challenges when it comes to working with external data, even if it’s provided at no cost.” Because organizations connecting to the data aren’t the same organizations like the ones providing it, you may become overly reliant on data providers. You may also need to merge datasets from multiple locations, in multiple formats, that are updating at multiple frequencies. Finally, Marr writes, that by pulling in data from multiple places, you will need to adhere to different use restrictions and run into compliance issues that are different from those you have with internal data.
It’s clear that external data provides a benefit to organizations that can find a way to connect to it. Making sure that your data catalog supports the ingestion, discovery, and management of this data is going to be top-of-mind for organizations in 2022.
Data Monetization finds its footing
The data monetization market is set for rapid growth in 2022. According to a recent business intelligence report released by Data Bridge Market Research, the growth, size, and CAGR of data monetization are set to grow at a rate of 21.95% from 2022-to 2029.
For the past 5 years, data monetization has been a catchall term for any organization that’s trying to find a way to generate ROI from their data over and above using it to enhance analytical capabilities. A good monetization strategy allows a data science division to continue using data to experiment with new models because the bottom line investment in the team is balanced by revenue-generating datasets coming out of the same division.
The problem is that data monetization, like AI, is a goal achieved only when a data infrastructure is set up on rails. The reality is that most companies don’t yet have an environment that’s ready to spin data exhaust into monetizable data products. Even if they did, most businesses don’t have the capacity to build a distribution mechanism to get the data into the hands of consumers.
It’s clear that companies that figure out data monetization quickly are in a position to own a large market share of this exploding market. Before that happens, they’ll need to make sure they have the data infrastructure in place to generate data products and distribute them securely.
Analytic success needs multi-cloud support
More than ever, data is coming from everywhere and is distributed across environments. As Sudhir Hasbe wrote recently in Forbes “over 90% of large organizations already deploy multi-cloud architectures, and their data is distributed across several cloud providers.”
An ideal solution might be to migrate all this data to a central environment (cloud companies, at least, would love that), but undoing years of architecture and lift-and-shifting is at best an expensive headache and at worst a logistical impossibility for most large companies. Instead, it’s more important for these organizations to find ways to perform cross-cloud analytics without moving the data at all.
Without cross-cloud support, data teams will continue to run into challenges. Data silos prevent them from discovering, accessing, and analyzing data from different environments. Additionally, writes Hasbe, “each cloud platform vendor provides a unique set of analytical tools. The lack of uniformity between these tools makes it difficult for data teams to analyze efficiently.” Ideally, data teams can use their preferred tools, no matter what environment the data is coming from.
The goal for any organization that’s trying to become data-driven should be to support the analysis of data where it lives rather than break down the silos themselves.
The rise of solutions that support intelligent data procurement
Being a data procurement officer must be difficult right now. The need for new data has never been more widely acknowledged, but budgets are tight and regulatory compliance is increasingly difficult to navigate. Being a data procurement specialist means working closely with your data team to understand their goals and analyzing what datasets they can use to achieve these goals.
Problematically, there’s often not a lot of insight into what lift, if any, is achieved by any given dataset. Organizations pour resources into a data science division and may see a year-over-year benefit, but the procurement officer may not know what datasets are providing the greatest value. It’s possible that a dataset that costs nothing is being used by dozens of analysts and generating real value and the dataset that costs $500k/year is only exported every couple of months by the same analyst who uses it for benchmarking.
Intelligent data procurement means that an officer can easily monitor and report on data use throughout their organization. What datasets are used most often? Which ones aren’t used at all? Can I extract more value from this dataset? What’s coming up for license renewal? Can I consolidate licenses and save some money? These are questions that any procurement officer should be able to answer quickly, without needing to run a comprehensive data audit first.
In 2022, we’ll start to see a lot more solutions in the market that support this data persona. Data profiling and data reporting tools will start to become a necessity for any organization that’s modernizing its tech stack.
“Data Fabric” matures
Data Fabric is a term that is getting increased exposure, and for good reason. Data Fabric is, according to Gartner, a “design concept that serves as an integrated layer of data and connecting processes.” It runs continuous analytics over metadata assets to support the deployment of integrated data across all environments, making it ready for machine-reading and AI.
The problem with data fabric to date is, like the pre-pandemic AI craze, it’s a holistic design that comprises an entire ecosystem. In reality, there’s no current solution in the market that analyzes all your data assets, inferences connections between them, and discovers unique business-relevant relationships. Data fabric is a goal, not a platform.
That said, there’s a lot of value in using data fabric as a concept that underpins tech procurement. Discovering what solutions you should use for metadata management, intelligent data discovery, creating a data asset inventory or data catalog, and out-of-the-box automation should be top of mind for any organization that’s trying to build a modern tech stack.
___
As the complexity of data environments increases, new tools and technology will emerge to mitigate the difficulty of discovering, governing, and connecting to data. In the next 2-5 years we will see an emergence of tools that support not only the data science and engineering division, but underrepresented data-facing departments like procurement, governance, and business units. Organizations that embrace tools that support actionable, cross-departmental goals will be the ones that benefit the most from the evolving data economy.