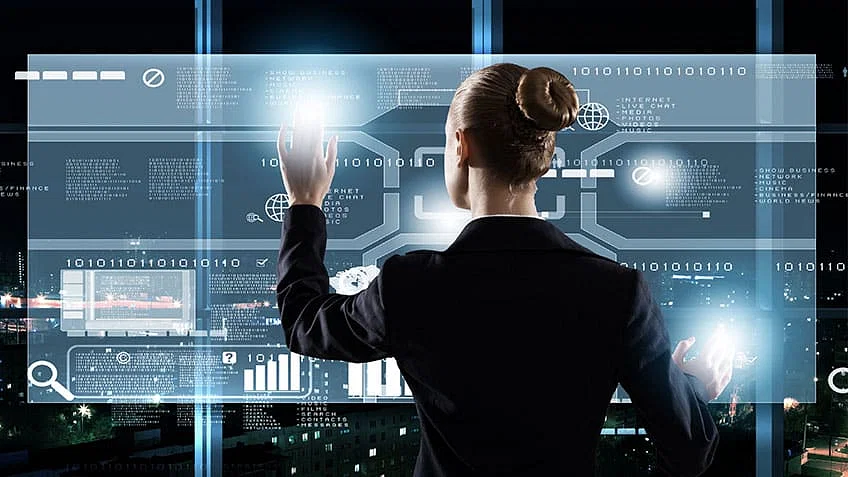
Big Data Management has become a pivotal part of modern business, influencing decisions, shaping strategies, and offering unparalleled insights. With the exponential growth of data from myriad sources, managing it effectively is more critical than ever. However, big data’s sheer volume, variety, and velocity present a unique set of challenges. These challenges range from integration and quality control to security and performance, requiring robust solutions and innovative approaches.
This post explores the complex landscape of extensive data management, delving into the critical issues organizations face and the solutions that can overcome them. By understanding these challenges and embracing the tools and methodologies that can address them, businesses can unlock the true potential of big data, transforming raw information into actionable intelligence.
The explosion of Big Data: A brief overview
In recent years, the world has witnessed an unprecedented amount of data generated. This phenomenon, known as Big Data, encompasses Information from various sources, such as social media platforms, IoT (Internet of Things) devices, e-commerce websites, and more. It is not just the staggering volume but also the variety – structured, unstructured, semi-structured – and the velocity at which it is created and processed.
The role of big data has expanded across various industries, including healthcare, finance, retail, and transportation. In healthcare, it is used for personalized medicine and predictive analytics; finance, fraud detection, and risk management; retail, customer behavior analysis, and inventory optimization. The applications are limitless.
However, the sheer scale of big data brings complexities and challenges in management. The need for efficient storage solutions, integration of data from disparate sources, ensuring quality, and real-time processing demands innovative and robust management strategies.
Challenges in Big Data management
Data integration and quality control
Integrating several types of data from diverse sources is a complex challenge. This includes merging structured data from traditional databases with unstructured or semi-structured data from emails, social media, etc. Ensuring consistency and quality becomes an uphill task, requiring sophisticated algorithms and manual oversight. Inferior quality data can lead to inaccurate analyses and misguided decision-making, making quality control paramount.
Data security and compliance
As data volumes grow, so do the risks related to data security. Protecting sensitive Information, customer details, or intellectual property, is crucial. Compliance with regulatory requirements like GDPR (General Data Protection Regulation) adds another layer of complexity. Not meeting these standards could lead to legal repercussions and harm one’s reputation.
Performance and scalability
Handling large datasets requires robust storage solutions and high-speed processing capabilities. Traditional systems may falter under the sheer load of big data, leading to performance bottlenecks. Scalable solutions that can adapt to fluctuating data volumes are essential to prevent system failures and maintain efficiency.
The complexity of data preparation
Data preparation involves cleaning, transforming, and enriching data to make it suitable for analysis. This can be time-consuming and labor-intensive, often taking up to 80% of the time in a data project. The complexity is compounded when dealing with big data, as inconsistencies, missing values, and errors become more prevalent.
Real-time analysis and processing
Big data is often most valuable when analyzed in real time. Whether tracking stock market trends or monitoring health conditions, the ability to process and analyze data as it is generated is vital. Traditional batch processing methods may not suffice, necessitating the development of real-time analytics capabilities.
Skill gap and resource constraints
Extensive data management requires specialized skills and knowledge. The market has a noticeable skill gap, with a need for more professionals with expertise in big data technologies. Additionally, small, and medium-sized businesses may find the costs associated with extensive data management prohibitive.
Privacy and ethical considerations
With the collection and analysis of personal information, privacy concerns arise. Ethical considerations around consent, transparency, and the potential misuse of data must be addressed, requiring clear policies and adherence to ethical principles.
Solutions to Big Data management challenges
Data integration and quality control
- Solution: Utilizing robust data integration platforms and employing data wrangling tools can automate cleaning and transforming data. Machine learning algorithms can detect inconsistencies while continuous monitoring maintains quality.
Data security and compliance
- Solution: Implementing advanced encryption methods, multi-factor authentication, and strict access controls can enhance data security. Regular audits and alignment with regulatory frameworks ensure compliance with legal standards.
Performance and scalability
- Solution: Leveraging cloud storage solutions and distributed computing frameworks allows organizations to scale according to data volumes. Virtualization and containerization technologies can enhance performance, making the system more responsive and resilient.
The complexity of data preparation
- Solution: Automated data preparation tools can streamline the process, reducing the manual effort required. Devices equipped with AI (Artificial Intelligence) can learn from human inputs and progressively improve the efficiency of data cleaning and transformation.
Real-time analysis and processing
- Solution: Real-time data processing engines and in-memory computing enable instant analysis and response. By integrating these technologies into their existing systems, businesses can take advantage of opportunities or minimize risks as they arise.
Skill gap and resource constraints
- Solution: Investing in training and development can bridge the skill gap while outsourcing specific functions to specialized service providers can alleviate resource constraints. Collaboration with educational institutions and industry partners can foster skill development within the community.
Privacy and ethical considerations
- Solution: Developing clear privacy policies and ethical guidelines and transparent communication with stakeholders can build trust. Regular reviews and ethical oversight committees can ensure ongoing alignment with societal norms and values.
Leveraging analytics and visualization tools
- Solution: Analytics platforms and visualization tools can transform raw data into actionable insights. They allow businesses to identify trends, uncover hidden patterns, and make data-driven decisions. Custom dashboards can be created to cater to different stakeholders, ensuring that the Information is user-friendly.
Implementing distributed systems
- Solution: Distributed systems like Hadoop and Spark allow for parallel processing, handling large data sets efficiently. They provide fault tolerance and can be scaled up or down according to needs, offering flexibility and cost-effectiveness.
Cloud-based solutions
- Solution: Cloud providers offer scalable, flexible, and secure solutions for extensive data management. They provide the infrastructure and services needed, allowing organizations to focus on extracting value from the data rather than managing the underlying technology.
Machine learning and AI integration
- Solution: Machine learning and AI can automate many processes within extensive data management, from predictive analytics to anomaly detection. They can add intelligence to the system, adapting to changing conditions and providing personalized solutions.
Incorporating data governance framework
- Solution: A well-defined data governance framework can guide data management within an organization. It ensures that data is handled consistently, responsibly, and transparently, aligning with both internal policies and external regulations.
Conclusion
In the digital transformation era, extensive data management has emerged as both an opportunity and a challenge for businesses. The complex landscape of data integration, security, scalability, and ethical considerations requires a comprehensive approach to harness its true potential. Organizations can overcome hurdles by employing innovative solutions, including Data Wrangling tools, and maximize the value derived from vast data resources. Embracing automation, cloud-based technologies, AI, and robust governance frameworks alleviates the complexity and propels businesses toward growth and sustainability. The future of extensive data management lies in strategic adaptation and continual innovation, shaping a new paradigm in the data-centric world.