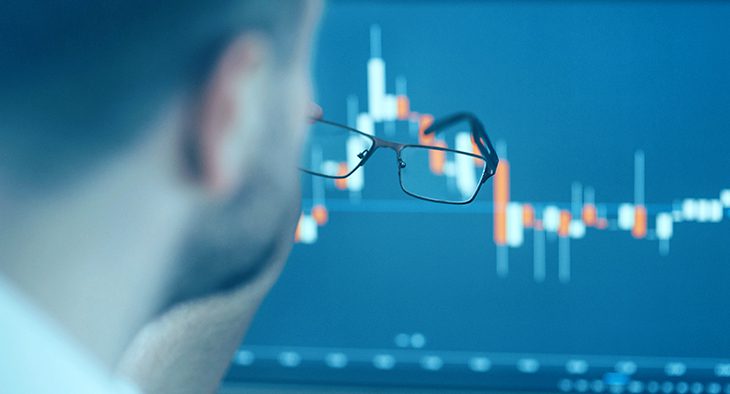
With the world’s data multiplying in leaps and bounds, every organization is trying to make better business decisions in marketing, product development, and finance using insights from the data they hold. The value of businesses today can be measured by the quality of the data they hold. With this in mind, data has become a very critical part of the business world. It is therefore imperative that data must be accurate and of very high quality for it to offer its best uses. That said, how do you maintain data quality? This article seeks to expound on how an organization can maintain high data quality.
In our previous blog series on the DQLabs.ai blogs section, we talked about data quality management, which entails the processes adopted by organizations to ensure data quality. The processes are geared towards deriving useful insights from data to draw accurate business results. The steps below outline how organizations can ensure data quality;
Data monitoring. Data monitoring entails the process that organizations undertake to review and evaluate data to make sure that it fits the purpose it is intended for. The process goes further to verify the data against the set standards to make sure that it meets them.
Data curation. The next step in ensuring data quality involves cleansing data. This is a critical step that entails validating data, checking for inconsistencies, and uniqueness, and uncovering the relationships within the data. This data curation process is used by many organizations as the first step in data analysis. Here is an article to explore more on what is data curation.
Central management of data. In many organizations, multiple people and software gather and clean data every day. These people may be working from different locations or offices. Clear policies are therefore required to manage how all the data is gathered, collated, and managed within the organization. Centralized management of data is the optimal solution that reduces inconsistencies and misinterpretations. It also helps to establish a corporate standard for handling data.
The other step is Documentation. By ensuring that all the data requirements and proper documentation is maintained, data quality is assured. The requirements and documentation in respect to the data processors as well as the sources of the data must be captured. Data documentation captures a data dictionary that provides guidelines for handling data to its users and any new user in the future. It also captures the processes and procedures for support to data users.
To maintain data quality, all data in an organization must be consistent with the set data rules and organizational goals. This consistency must be checked for at regular intervals. During the check, the present status of the organizational data must be captured and relayed to all the stakeholders. This process ensures the quality of data is maintained at all times in the organization.
Enforcing data integrity ensures that the quality of data in an organization is achieved and maintained. A good relational database has the ability to enforce data integrity using various techniques, including foreign keys, triggers, and check constraints. In a typical database, not all datasets can be stored together, especially when the volume of the data grows. A relational database enforces the referential integrity of data by defining the best data governance practices. With the volumes of data being handled by organizations today, the referential enforcement of data has become more complex, leading to inconsistent data, which has integrity issues, leading to data quality problems.
The next step involves the integration of data lineage traceability into data pipelines. In a properly designed data pipeline, the time taken to troubleshoot data issues should not change depending on the volume of the data or the complexity of the system. A data lineage traceability encompassed into the pipeline ensures that data issues are tracked down and resolved faster, enhancing the quality of the data.
The other step involves automated regression testing as part of change management. Usually, data quality issues occur when new datasets are added or when existing datasets are modified. For any effective change management, the test plans should be built with the aim to confirm that the change meets the set requirement and ensuring that the change does not cause an unintended impact on any data in the pipelines that were not supposed to be changed. For organizations handling large volumes of data, automated regression tests that incorporate thorough data comparisons are necessary to make sure that good data quality is maintained.
Summary
In conclusion, ensuring data quality is a continuous process rather than a one-time activity. It requires; data monitoring, data cleansing, central management of data, automated regression testing, and integration of data lineage traceability into data pipelines, among other processes. This article outlines the best practices that will help an organization ensure the good quality of its data.