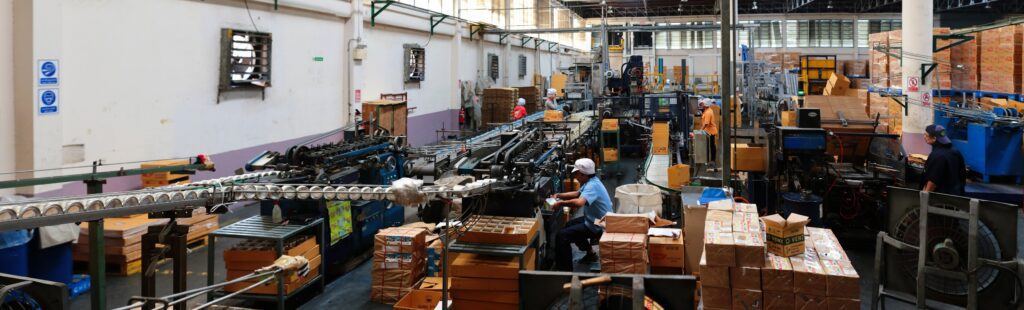
Large language models (LLMs) for generating text and vision models for generating images are notoriously inefficient. The larger they get, the more power hungry they become.
Kisaco Research in September hosted a one-day event in Santa Clara dedicated to the topic of generative artificial intelligence (GAI) efficiency, followed by a three-day Summit on Hardware and Edge AI. Stay tuned for my relevant impressions of that event as well.
During his keynote at the EGAIS, General Partner Mike Shirazi of Pursuit Ventures offered these statistics and observations on the opportunities and efficiency challenges of GAI:
Global revenue forecast: 42 percent CAGR for GAI, reaching $1.3 trillion in 2032 from $14 billion in 2020. (Bloomberg Intelligence)
Compute power and hardware crunch: Greater than 10x computing power needed for new models. Haven’t invented enough innovation at the hardware layer.
Emerging tech in play: Photon level development will lower the power requirements.
Compute-related emissions: GPT-3 training generated 284 tons of GHGs; 4 tons daily to operate ChatGPT every day. (Google and UC Berkeley)
Demand for GAI is clearly substantial and the emissions impact is just as obvious, which means the interest in making GAI more efficient is strong as well. It’s fortunate that new architectures and technologies that can help have been in development for years now.
Will Numenta’s research on the human brain and computing pay off soon?
There are several alternative chip architecture and design approaches that compete with the graphics processing units (GPUs) like Nvidia’s. On the low-power front, for example, neuromorphic chips are available that are inspired by the efficiencies of biological systems.
GAI is a foundational class of technologies with hundreds of potential use cases, many of which have yet to be explored. As such, the workloads for text and image generation can vary substantially.
To date, most GAI processing has been done in the data center, but potential for edge computing applications is high. As a result, different kinds of processor technologies are already becoming part of the GAI mix, including application-specific integrated circuits (ASICs) and systems on a chip (SoCs).
Numenta’s been focused on studying the human brain for nearly two decades now. Co-founder Jeff Hawkins published A Thousand Brains: A New Theory of Intelligence on the topic a couple of years ago, which described the company’s findings on how the brain’s inner workings inform attempts to refine neural networks.
During the week of September 11th, Subutai Ahmad, the company’s CEO, announced the Numenta Platform for Intelligent Computing (NuPIC). Ahmed claimed NuPIC’s architecture will allow CPUs to become more performant and efficient than today’s GPUs in GAI applications. Today’s neural nets in commercial use are rudimentary by comparison with what’s possible, taking advantage of only a fraction of what scientists have discovered about the brain recently.
Ahmad noted that the human brain only uses 20 watts of power. Each neuron acts as an elegant, sophisticated computing device on its own, one that can manage to build context with only sparse data.
Ahmad contrasted GAI with non-generative AI (NGAI) and pointed out that the latter “understands” and can be reliable when it comes to comparing and classifying text, though it’s less able to handle long context at this point.
When it comes to GAI, users have to run the model hundreds of times, one reason that GAI model use demands 10,0000 to 100,000 times more compute power than NGAI.
But Numenta is focused on both GAI and NGAI applications. Ahmad claimed that Numenta’s benchmarked price/performance using the pretrained English BERT-Large language model is ten times that of Nvidia’s GPUs.
Ahmad also mentioned that Numenta’s working with gaming platform luminaries Will Wright (creator of Sims and SPORE) and Lauren Ellioton more interactive, laptop CPU-based gaming. Their venture is called Gallium Studios.
Other alternatives to GPUs
It was evident that Nvidia’s A100 GPU is seeing unprecedented demand and short supply, with demand for the more powerful H100 cards also evident. H100 clusters for AWS users became available in July 2023. Rashmi Gopinath, General Partner at B Capital during foundation model efficiency panel at the Summit said that some users are facing initial budgets that are up to 80 percent allocated to hardware because of the lack of access to GPUs.
Alternatives to GPUs besides Numenta’s mentioned at the Summit included Gaudi2 accelerator from Intel’s Habana unit. Habana Software Product Head Sree Gamnesan observed that some newer LLMs include trillions of tokens, so sufficient scalability is a major requirement.
Gaudi2, she said, offers speed, scaling, ease of use, power and cost efficiency versus an A100-based solution. Habana claims that a single server node with eight Gaudi2s can enable inference of a 178 billion parameter model. Habana’s been busy adding support that developers are demanding for PyTorch, Hugging Face, and pre-built Docker container images, and promises FP8 support and optimizations by the end of Q4 2023.
Overall impressions
GAI is opening up infrastructure innovation opportunities at various levels of the stack, not just in the area of specialized processing units. I shouldn’t have been surprised at how much interest and activity there is in making GAI (mainly a consumer end user and development phenomenon to date) real and feasible for enterprises, but I was. Looking forward to finding out more over the next several days.