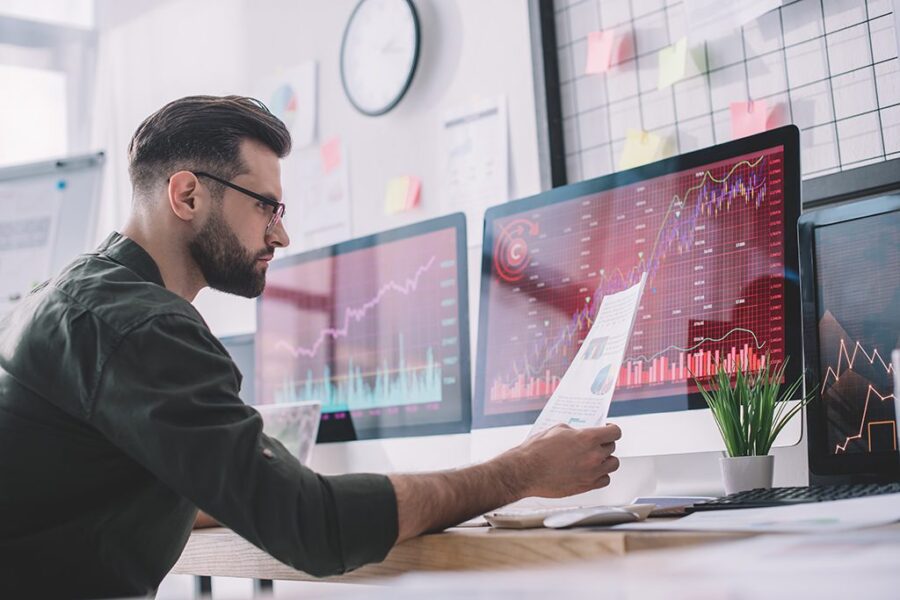
This article continues to the previous Part I – 10 steps to data profiling.
Step 6
Ensure that the data selected for profiling meets the regulatory threshold. It is important to understand the regulations governing the target data to achieve this. In some cases, organizations that own the data may not have the user’s permission to freely use the data generated from their interaction with the organization. In today’s age where massive data is being generated online due to innovation in the eCommerce and social media industries; many governments have passed laws aimed at protecting their citizen’s data from being commercially used to exploit them. Many companies have had hefty fines imposed on them by regulators for violating these rules in their data analysis endeavors.
Remember, different regulations in different jurisdictions govern different data. A business operating in several jurisdictions has to meet the regulations set in each jurisdiction to avoid hefty fines or possible lawsuits. To achieve a smooth data profiling process, the analyst has to harmonize the data to meet the regulations while still remaining useful to the analysis process. Lack of harmonization can lead to incomplete data sets being available for the next step of the analysis plan. An organization should involve the legal department or someone who is familiar with the jurisdiction laws while deciding which data will be made available to the analyst. The analyst and the legal team should agree on how the affected data will be accessed and utilized.
Step 7
Ensure that the target data meet privacy regulations. Consult the organization to get a list of the data they collect and the privacy requirements of that data. For instance, medical records are private in most countries and allowing access can violate the patient’s privacy. The analyst, in this case, should only request medical findings and general patient details such as gender and payment method used. In the recent past, many people have successfully sued companies for violation of their privacy rights while handling the consumers’ data.
A thorough analysis of the privacy data that will be used and how it will be used should be undertaken at this stage. Any potential violations should be addressed before they become a legal problem for the organizations. An analyst must exercise restraint in selecting the depth of the data they wish to use for their analysis. While private data can significantly enrich an analysis report, it can damage the organization’s integrity in the eyes of the partners and clients. Steps to data profiling that an analyst can take to gain important information while still protecting the privacy of personal data:
De Identification: This is the stripping of personal data that could identify an individual from the targeted data sets.
User Access Control: This ensures that only authorized personnel can access with reason data that can be used to identify a person.
Step 8
Ensure that the source data identified will be available on request. Ensure that all the data identified will be available to the analyst during the data profiling stage. Any data that cannot be continuously available should be given priority to maximize the value derived from the data. Alternatively, an analyst can come up with a schedule to be granted access only when they need the data. Remember, the data profiling plan will rely heavily on successful access to the target data.
When the data analyst and the data management team fail to coordinate during the data analysis process continually, missing data or altered data is a common problem. An analyst should communicate with the data management team about the data sources they have chosen, when they’ll need them, and for how long. Failure to do this can lead to useful data archived or deleted after the analyst has identified it as source data.
Step 9
Ensure that the source data is in a usable format. Ensure that the source data files are in formats used during the data profiling stage. Corrupt files or files that are not usable but are necessary should be identified to get repaired or for the analyst to identify another source of the same data. After identifying all the source data needed, an analyst should finally ensure that the data is in a usable format.
In the case of AI-driven platforms such as DQLabs.ai, this is not a major hurdle as it can accommodate data in countless formats. In traditional data analytics, the data format had to be changed to match the format of other data sets. This modern analytics system will also highlight the corrupt files for the analyst and recommend actions that will enrich the quality of the data.
Step 10
Create a data profiling plan. This plan ensures that the data profiling process follows a logical order to gain the most insight into the target data. A data profiling plan borrows heavily from the priorities set in ‘step 5’ and ensures that the analyst keeps regulatory requirements in mind. The data profiling plan should consider how the data being profiled was generated. For Instance, customer data entered manually is more likely to have erroneous entries and thus is likely to consume more time. By understanding how the data was generated, the analyst will know the type of errors and the quality of data they are profiling.
Conclusion
Before embarking on the data profiling exercise, an analyst must prepare by going through the data profiling steps listed above. This will ensure that the data that they know all the data to use, where to get it, the State it is in, and the rules governing the data. With all this in mind, an effective data profiling plan will guide the analyst to maximize the outcome of the data profiling stage. Successful data discovery will rely heavily on all the above data profiling steps taken to prepare for an effective profiling plan. An analyst should also understand how the data selected as source data was corrected to know what to look for during the profiling stage.
DQLabs platform has a data profiling platform that is AI-driven and accepts data from multiple sources in different formats if necessary. The user interface is user-friendly and will allow users to track the data profiling process and make adjustments where they feel necessary. The platform algorithms will detect deep insight into the source data and increase the quality of the profiled data.