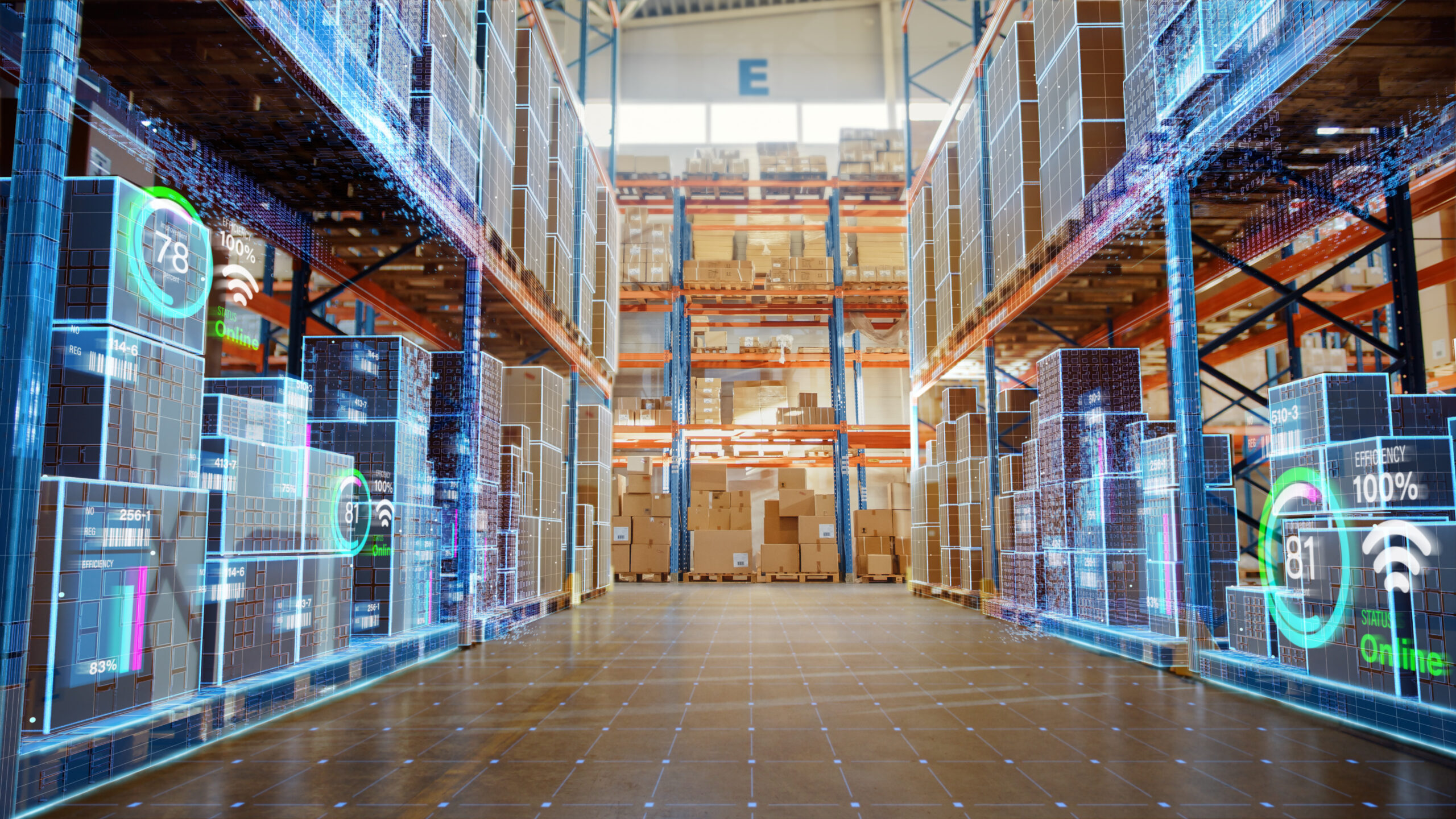
Data warehouses are at the heart of any organization’s technology ecosystem. The emergence of cloud technology has enabled data warehouses to offer capabilities such as cost-effective data storage, scalable computing and storage, utilization-based pricing, and fully managed service delivery. As data consumption increases and more people live and work remotely, companies are adopting modern data warehouse technology to handle higher data volumes. As such, IT spending continues to shift to cloud computing, thereby creating opportunities for companies to attain digital business transformation.
Transitioning to the cloud comes with challenges, but the advantages outweigh the risks for companies that seek cutting-edge data warehouse technology. It is essential for data leaders to strengthen their knowledge about common impediments, create a data warehousing strategy, and shift to the cloud. If they don’t, misconceptions about costs, migration complexity, data security, and flexibility will make data warehouse leaders reluctant to adopt modern solutions. A better understanding of these misconceptions is vital to business success.
Hybrid cloud systems enhance data warehousing capabilities
A hybrid cloud connects private on-premises data centers to a public cloud, enabling data and applications to share information from both on-premise and cloud systems. This hybrid cloud setup helps organizations leverage multi-cloud and on-premises data centers. A key benefit of a hybrid cloud is agility. The ability to change direction quickly is the foundation of any successful digital business. A combination of public, private, and on-premises resources supports agility and improves reporting through integrated artificial intelligence (AI) and machine learning (ML).
Understanding common data warehouse misconceptions
Fallacies about cloud data warehouses may cause IT professionals to question whether they should adopt new data warehouse systems and move to the cloud. Here’s the truth about these misconceptions.
- Data warehousing is analyzing the business using only past data. Data warehousing can be built to handle real-time analytics using modern tools. When considering conventional design for real-time reporting and analytics in enterprise data warehousing, a good option is to use data replication technologies, such as Oracle Goldengate or Shareplex. These integrated data repository (IDR) tools replicate the data from online transaction processing (OLTP) databases to data warehousing databases. They help to implement the extract, transform, and load (ETL) and extract, load, and logical transformation (ELT) online. In the modern data warehousing world, Kafka Spark streaming will archive real-time analytics in the data lakehouse environment. It is essential to plan and manage the lag for the real-time definition as it will vary based on how companies define the real-time solution It is an enterprise’s responsibility to decide and define the definition of lag for real-time data replication and configure the real-time data in the decided lag time. If companies decide to define real-time data with a few hours of lag time, real-time processing can be attained through traditional batch processing.
- There is no common structured query language (SQL) engine to process all types of data in data warehousing. It’s possible to build a common SQL engine in the modern data warehousing environment. Companies depend on their enterprise data warehouse solution if they are processing structured data, or they may use a data lake option to customize and process unstructured or semi-structured data. Some companies use a data lakehouse system with a single SQL engine like Snowflake or Databricks as a unified data processing engine. These solutions, however, should be explored for cost, performance, and data processing. For example, Spark Engine works best for batch processing, but not for simple SQLs with low throughput. A better data lakehouse architecture will analyze the business requirement and build the appropriate SQL engine based on the pattern of data. For instance, a single data lakehouse solution uses a presto engine to process simple SQL with low throughput, and the Spark Engine is utilized for batch processing.
- Modern data warehousing is possible only through cloud providers. Modern data warehousing needs to be built based on enterprise data and its priorities. For example, if the data is sensitive, it needs to be housed in its own data center. At the same time, companies stand to gain the benefits from cloud providers for non-sensitive data warehouse situations as well. In these circumstances, a hybrid data warehousing approach is the appropriate solution. Technology like Cloud Pak for Data process data in real-time from on-premises and cloud data centers simultaneously. Cloud Pak for Data is highly scalable both up and down, providing better performance and creating a hybrid cloud solution for data warehousing.
- It’s difficult to manage data governance in data warehousing. Today, many companies struggle to manage their data lineage. This challenge can be resolved by planning and designing using a data hub solution like Watson Knowledge Catalog or Apache Kyligence Semantic Layer. For example, Watson Knowledge Catalog offers advanced capabilities, like creating virtualized tables from multiple data sources, as well as a single virtualized table with a few columns from multiple different data sources. The solution also provides common engines when users execute SQLs. It internally converts SQL and transports it to the appropriate data sources. These solutions and other emerging technologies can help companies better manage data lineage and governance.
- Data warehousing uses more storage and is expensive to build. Companies use hundreds of different types of databases to manage and process their business requirements. As such, they need to be able to consolidate data from all data sources for reporting or AI and ML requirements. Often, data warehouse leaders choose inexpensive solutions like object storage or they build their own SQL engine to process large volumes of data. Additionally, managing data in traditional block storage is expensive. In these situations, data fabric architecture is a better option for a next-generation solution in data warehousing. The ideal data fabric architecture provides a common SQL engine to process structured, semi-structured, or unstructured data from the relational database management system. Cloud Pak for Data and Watson Query are two examples of data fabric solutions. Because the data fabric architecture processes data directly from the online transaction processing or business database, it reduces costs and eliminates the need for a separate data warehouse solution.
Plan for the future
Adopting a data warehouse solution requires preliminary work, including data management and governance, platform automation, data movement and replication, data modeling and preparation, and infrastructure monitoring. When executed well, these strategies enable organizations to document their current environment, plan their modern platform, migrate their legacy data structures, and govern and automate the new platform. By addressing cloud data warehouse misconceptions and understanding the challenges, benefits, and total cost ownership of data warehouse models, organizations can make better decisions about their cloud data warehousing strategy and unlock the true value of their data.
About the Author:
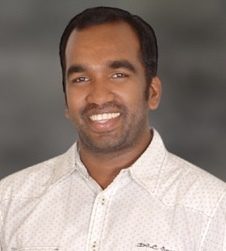
John Thangaraj is a senior brand technical specialist with over 15 years of experience as a lead database architect. He is highly skilled in OLTP database and data warehouse infrastructure architecture, relational and dimensional data modeling, logical and physical database design, data architecture, data integration, ETL, and SQL optimization. For more information, contact him at [email protected] or on LinkedIn.