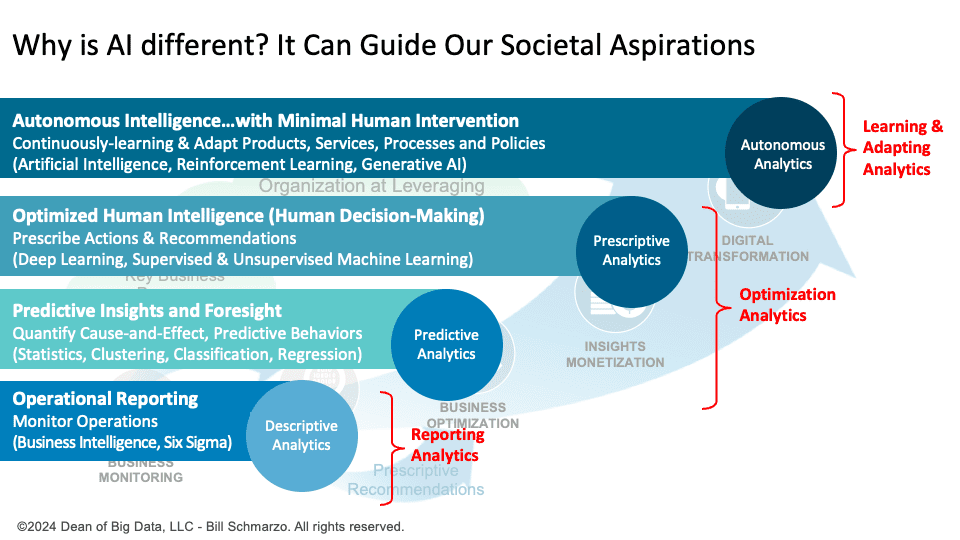
Traditional analytics optimize based on existing data, reflecting past realities, limitations, and biases. In contrast, AI focuses on future aspirations, identifying the learning needed to achieve aspirational outcomes and guiding your evolution toward these outcomes.
When I talk to my students, the question I keep getting is, “Is AI really different from traditional analytics?” My answer is yes. It’s different because it can learn and adapt to operating in incredibly complex, dynamic situations with minimal human intervention.
Traditional analytics are reactive and focus on analyzing past or current data to optimize decisions within pre-existing patterns. On the other hand, AI analytics are predictive and prescriptive, with a learning and adaptive component. They can not only optimize based on past data but can also learn from new data to achieve more aspirational outcomes in the future.
To build AI models that help us realize our full potential, we need a strategic vision that develops analytics capabilities to optimize current operations, drive transformation and innovation, and expand the metrics upon which we measure contributions to society and humankind. This requires us to envision a more aspirational future considering our desired societal outcomes and the metrics we will use to measure society’s progress toward those outcomes (see Figure 1).
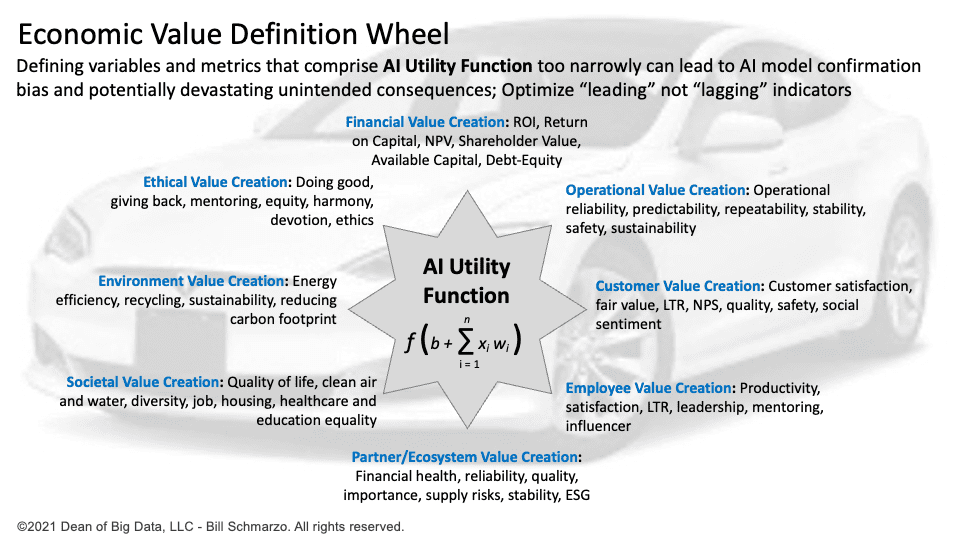
Figure 1: Broaden How Society Will Measure AI Success
For example, integrating considerations like ESG (Environmental, Social, and Governance) into AI analytics reflects a broader trend where businesses align their operations with societal values and long-term sustainability. AI can enhance this by identifying trends, risks, and opportunities in ESG criteria, thus guiding corporations towards sustainable practices and reporting.
This requires a shift in our mindset; shifting from productivity-focused metrics (doing things faster or cheaper) to value creation is critical in this transition. Our goal should be to generate economic value, leverage data as an asset, and focus on outcomes that benefit customers, shareholders, and society. AI analytics can drive this shift by enabling deeper insights and facilitating innovation that traditional methods may overlook.
Yes, AI can help us craft the future we want. But to accomplish that, we must build AI models that reflect what we want to become, not just what we are today.
Importance of AI Utility Function
The AI Utility Function is a distinct feature of AI analytics that sets it apart from traditional analytics. The feedback mechanism is the key to an AI Utility Function that can continuously learn and adapt. The feedback mechanism steers the AI model’s learning and decision-making processes by defining optimal outcomes and their associated economic value and weights. It enhances adaptive learning and decision automation and facilitates the ongoing improvement of model performance (see Figure 2).
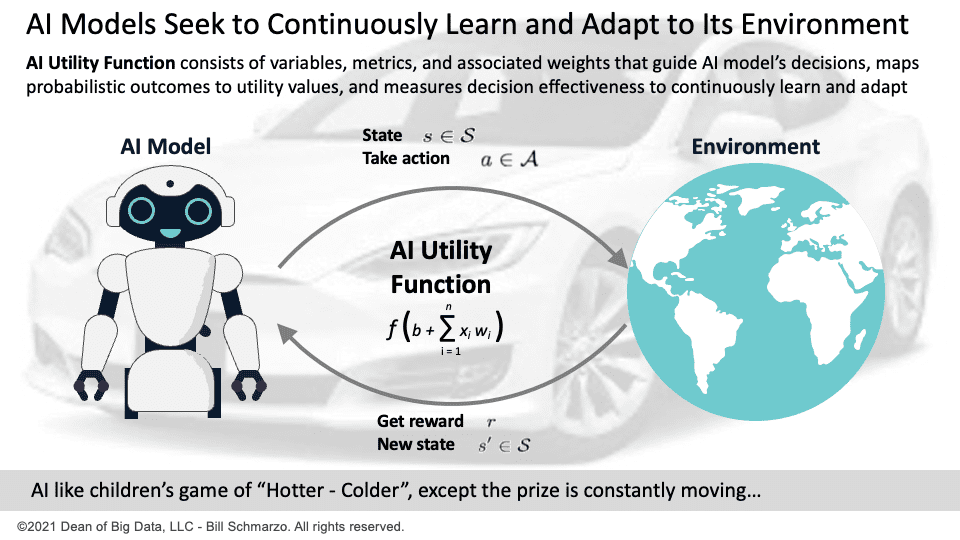
Figure 2: AI Utility Function and Feedback Mechanism
The AI Utility Function feedback mechanism enables AI models to learn and adapt without human intervention, distinguishing AI analytics from traditional analytics (see Table 1).
Traditional Analytics | AI Analytics (AI Utility Function) | |
Adaptive Learning Capabilities | Typically, it lacks a mechanism to autonomously adapt or optimize based on changing data inputs without human intervention. | The AI utility function in AI models (especially in reinforcement learning) guides the system to learn from new data and experiences to maximize its defined utility, inherently supporting ongoing adaptation. |
Complexity of Models | Traditional models are often straightforward. | The AI utility function can engage in complex decision-making that involves balancing multiple objectives, sometimes in conflicting scenarios. This allows AI models to handle complex, real-world problems with multiple variables and potential outcomes. |
Predictive vs. Reactive Analytics | Generally reactive, focusing on insights from historical data without a mechanism to project future states beyond simple forecasts | Uses AI utility functions to not only predict outcomes but also to proactively suggest changes or actions that will optimize future results based on these predictions |
Decision Automation | Provides data-driven insights that require human interpretation for decision implementation. | Techniques are usually limited to structured data in rigid databases and relational structures. They are often constrained by the scope of the data they are designed to analyze. |
Scalability and Efficiency | Scalability can be limited by the computational inefficiencies of older models and the need for human-centric, manual tuning. | Providees a mechanism to autonomously adapt or optimize based on changing data inputs without human intervention. |
Scope of Data Utilization | The AI utility function can engage in complex decision-making that involves balancing multiple objectives, sometimes in conflicting scenarios. AI models can handle complex, real-world problems with multiple variables and potential outcomes. | An AI utility function enables AI systems to derive actionable insights from varied data types and sources by defining what ‘useful’ outcomes look like across these diverse inputs, which can enhance the system’s ability to work with unstructured and semi-structured |
Table 1: Difference Between Traditional and AI Analytics
In the broader strategic context, the AI Utility Function enables systems to align closely with organizational goals. It essentially translates strategic objectives into computational objectives that AI systems can understand and act upon. This ensures that the operational activities driven by AI analytics are in harmony with the organization’s long-term strategic goals, a critical advantage when deploying AI in dynamic markets or environments where strategic agility is essential.
Summary: Importance of Societal Data & AI Literacy
Widespread education on data literacy and ethical AI usage is vital for increasing societal engagement with AI and data. There is a need for a comprehensive pathway to develop basic and advanced AI and data skills, emphasizing the importance of fostering a data-driven culture that extends beyond individual organizations to society. The democratization of data knowledge empowers citizens to utilize AI insights responsibly in their decision-making processes, promoting a more informed and proactive public.
I do have a suggestion… (Figure 3)
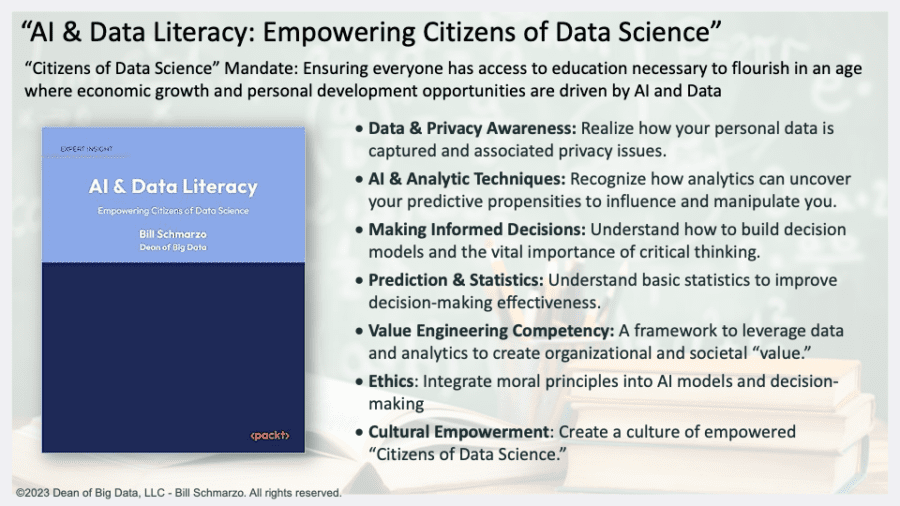
Figure 3: “AI & Data Literacy: Empowering Citizens of Data Science”
“AI & Data Literacy: Empowering Citizens of Data Science” proposes the following foundational framework:
- Societal Impact and Strategic Implementation: Integrate AI and data literacy into the core functions of societal structures, ensuring that these educational efforts are aligned with and actively support civic and social objectives.
- Ethical Frameworks: Guide how societal stakeholders can integrate ethical considerations into AI deployments to establish trust and integrity as AI technologies increasingly incorporate into daily life.
- Practical Applications for Wider Adoption: Illustrate how AI and data literacy can be effectively applied across various sectors of society through a series of case studies and real-life scenarios.
- Promoting Continuous Education: Emphasize the importance of ongoing education and skill development in AI and data-related fields, advocating for continuous learning initiatives that keep pace with technological advancements.
- Leadership in Societal Transformation: Directly engage leaders and policymakers, providing insights on leading societal change initiatives around data literacy to ensure widespread adoption and success.
- Fostering Community and Collaborative Learning: Promote the creation of collaborative communities around AI and data science, encouraging shared learning and collective problem-solving that extends across societal boundaries.
Through these elements, the book “AI & Data Literacy: Empowering Citizens of Data Science” educates and inspires societal change, positioning AI and data literacy as pivotal to informed citizenship and proactive participation in a data-driven future. The book acts as a catalyst and a guide for integrating AI into societal frameworks, driving towards a more informed, ethical, and collaborative future.
Top of Form