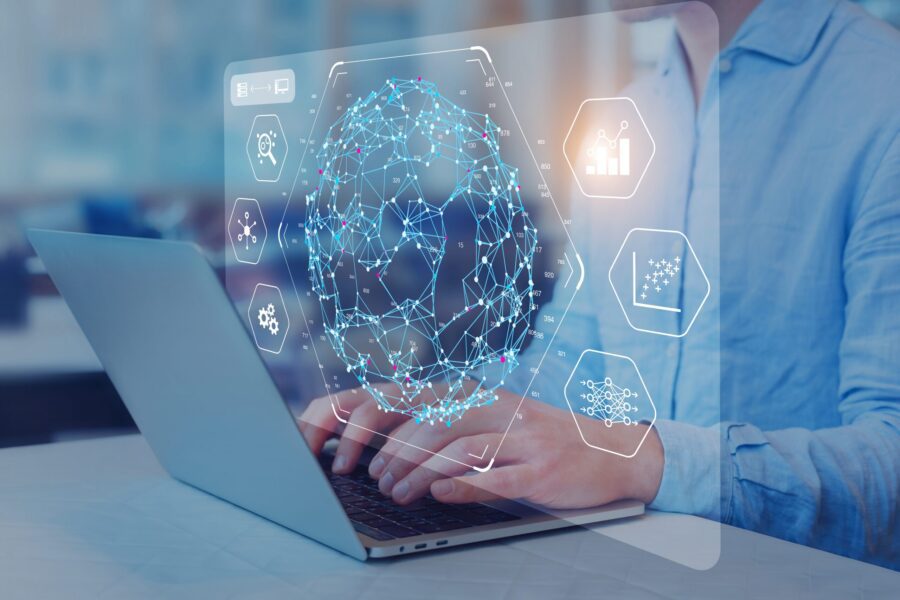
A way to counter AI risks could be to create AI risks. The question is by who, a non-profit, a corporation, a nation, or a treaty? It may take extremes in systems, across tasks, to find out the depths of threats.
If AI is used in weaponry, what are all the possible ways, such that when some deploy it in conflict, it can be detected, and if possible, prepared for how it might be countered, or to at least know that AI is behind?
The same applies to cybersecurity and bioweapons. This exceeds red teaming [or jail breaking], where instead of extricating weaknesses for a product or service, it is for impact in the real world, and divergence to misuse then consequences.
It is possible, in generative AI safety research, to unleash AI systems against stuff across scenarios, to become aware of areas where threats might show up, even if there are no clear answers yet, on how to handle them. This could be a premise of safety, beyond seeking AI alignment, to human values.
AI companies are putting in guardrails, which is fine, but their guardrails may not be what [solely] matters, since others may not, and may apply it for nefarious reasons. So, how can the labs be the solution, when the models of others are used for harmful stuff?
The surprises of deep fakes, voice cloning and so forth, are gaps in AI research, where risks stem from applications in the wild, rather than from explorations in closed, random circles.
There are ways to get a fairly large amount of people — spontaneously — exposed to some outputs of AI, without it being in the public yet, to understand how they would react, especially in situations where they might be emotionally involved. This is separate from beta testing or observations that led to emergent abilities, from internal experimenting. This will be more like phases of AI clinical trials.
For risks with election interference, there are several small-time elections across organizations, associations, and others that are not as consequential as national elections. They are samples to experiment with AI tools, where campaigns would have access to do whatever they want, then data on what they do, how the electorates react, the outcome, the irrationality of the campaigns and so forth. These would be used to understand how it is used and where prevention tactics may come in or help to shape awareness messages, then to use that for cases in national elections.
The purpose is consequence extents for what the application presents, to know what it might mean out there, and to prepare for it, while finding ways to communicate safety, more broadly, against people becoming casualties of it, especially if another team let it loose.
For AI research, the good thing might be that the safety team already had precautions that the world may use against those risks.
There are those who care about AI risks that are here and now. There are others who care about AGI risks and its takeover.
Those risks are possible as experiments in closed circles. For those who are worried about job losses from AI, roles in organizations where LLMs can partially or fully erase may be explored to see what might happen if an employee loses a job, or gets a pay cut, due to reduced performance or a takeover by AI.
There are firms that are already firing employees due to the role of LLMs in tasks. Those people can be tracked, for what they do next or their situations. The goal is to understand scenarios and extrapolate it, to prepare options, or at least know what might result, more than assuming that it will simply be really bad or there will be no meaning to life, if someone is unemployed for — the reason of — replacement by AI.
There are those worried about bias. The same applies, where AI is biased, those affected and the results, both in controlled but random studies and then in real-world cases and consequences, to prepare, find options and communicate effectively, all as aspects of research.
For AGI, what might AI want to do when it has autonomy?
Say, as a major data center, with super models, what might it want? If it is connected to a social media account, what might it want to do? Or, an email, or involved in drug discovery and so forth.
This question can be explored with bots, viruses, and several statistical cases across scenarios. Just to have at it and prepare for its reach, and find weaknesses, or blocks.
Since AGI will initially be digital, what are digital exposures that can drastically affect current life, and where must barriers be, for AI models to not be connected?
It is OK to restrict some outputs of some recent chatbots, with what they can answer, but AI safety exceeds those.
There are different categories of safety, but it will be tough to not play things out to know, similar to a tech gain-of-function. AI safety would be at different levels of efforts to include labs, blocs and so forth.
The ultimate AI safety will be to explore a possible way the human mind works, to state, display and expand the biochemical advantage the brain may retain over silicon wafers.