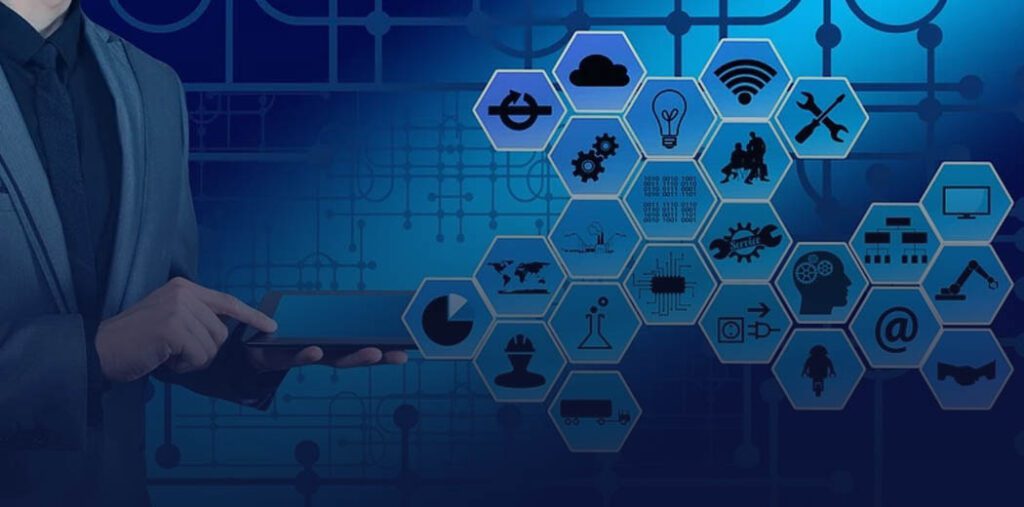
Data is the lifeblood of our digital world. We crave it, analyze it, and base decisions on it. But a hidden truth lurks beneath the glossy surface of charts and graphs: our data is often a muddy mess. Inconsistent, riddled with errors, and prone to manipulation, it can lead to faulty insights, misguided decisions, and even financial losses.
Emerging tools offer innovative ways to combat this problem. This blog covers some of the imminent trends in tech and how they help improve data quality.
Data quality and blockchain
At its core, blockchain is like a shared book of records updated by many computers. Each piece of data becomes a block and is connected to others in a chain. If someone tries to change one block, it messes up the whole chain, showing something’s wrong.
Imagine sending a document across the world. Normally, it passes through many hands, and someone might change it. But with blockchain, it’s locked up digitally and stays safe. Every step is checked, just like a sealed package that arrives unopened.
This transparency builds trust. Everyone involved with the data—from suppliers to consumers—can see where it came from and how it was handled. This transparency stops arguments because everyone knows the facts.
Blockchain doesn’t just keep records; it also stops problems. Since it’s not stored in one place, it’s hard for hackers to mess with it. They can’t break into one computer to change things.
Blockchain can help with data quality in many areas:
- Tracking products: It can show where things like food came from, so you know they’re real and safe.
- Medical info: It keeps sensitive info safe and only lets the right people see it.
- Voting: It stops cheating in elections by recording every vote.
- Digital money: It tracks who owns what in things like cryptocurrencies.
Real-time refinement with edge computing
Data is often stuck in big centers waiting to be checked and used. But now, we want info quickly and right where it comes from. That’s where edge computing comes in—it helps clean up data where it starts, making it better and faster.
Think of a factory with many sensors gathering information on temperature and production. Normally, this info travels far to a central computer, taking time. By then, it might be too late to act on it.
Edge computing changes this. It puts the power to process data closer to where it’s collected, like on devices or servers nearby. This means we can immediately fix mistakes or weird info before it messes up everything else.
For example, if a sensor gives a crazy temperature, edge computing can spot it fast and fix it locally without causing big alarms. This not only makes data better but also helps things run smoother.
Data quality doesn’t have to wait for centralized processing anymore. Edge computing brings the cleaning power closer to the data source, analyzing it in real-time at the network’s edge. This reduces latency, improves responsiveness, and ensures high-quality data feeds even in remote locations.
Edge computing helps ensure data quality in many ways:
- Less data traffic: It sorts data early, so only the important stuff goes to the main computer, saving time and money.
- Better accuracy: Checking data where it’s from makes it more accurate and valuable for local decisions.
- Quick reactions: Quickly fixing problems means less time wasted and things working better.
- More safety: Keeping sensitive data close by makes it safer and follows the rules about data privacy.
Advanced AI and ML
These intelligent tools are already shaking up the data quality game. Machine learning algorithms can automate anomaly detection, pattern recognition, and even predictive maintenance, proactively identifying and eliminating potential issues before they impact downstream processes. Artificial intelligence can further elevate data quality by understanding context, filling in missing values, and enriching data with insights from external sources.
AI and ML can do amazing things to ensure data quality:
- Predictive detection: They can look at old data and tell when there might be problems in the future, helping stop issues before they happen.
- Data enrichment: They can make data richer by adding info from other sources, like social media or demographics, making it more useful.
- Continuous learning: They keep getting better as they see more data. This process means they stay helpful even as things change.
Even with challenges such as ethical issues and biases, AI and ML can make data quality much better. They can save time, make fewer mistakes, and help us understand data better.
Sensor symphony and the IoT edge
The Internet of Things plays a critical role in enhancing data quality by enabling the collection of real-time, accurate, and diverse data from various sources.
- Data Accuracy: IoT devices collect data directly from sensors, machinery, or environments. This data is often more accurate because it’s captured instantly and consistently, reducing human error.
- Real-time Monitoring: IoT allows continuous monitoring of processes or environments. This real-time data can provide immediate insights, enabling faster responses to anomalies or issues, thus promptly maintaining data quality by addressing problems.
- Diverse Data Sources: IoT devices can gather data from various sources and formats. This diversity enriches datasets, providing a comprehensive view that can lead to better-informed decisions and improved data quality.
- Automated Data Collection: IoT devices automate data collection processes, reducing the need for manual entry. This automation minimizes errors and ensures that data is consistently and continuously gathered.
- Predictive Maintenance: IoT sensors can predict potential equipment failures or maintenance needs by analyzing patterns in data. This proactive approach helps prevent data quality issues caused by equipment malfunctions or breakdowns.
- Contextual Insights: IoT devices capture data in context. For instance, in a manufacturing setting, IoT sensors don’t just record temperature; they provide information about the machine, its location, and operational conditions. This contextual data improves the understanding and relevance of the collected information.
- Improved Decision-making: High-quality, real-time data from IoT devices allows for better-informed decision-making. Organizations can rely on accurate and timely insights to make strategic choices that positively impact operations.
Conclusion
As we move towards a more interconnected future, emerging technologies like AI, ML, Edge Computing, and Blockchain are revolutionizing how we ensure data quality. These innovations automate processes, provide real-time insights, and establish trust in data integrity.
While these advancements promise a future where data is accurate, predictive, and secure, they also present challenges like bias, privacy concerns, and the need for human collaboration. Overcoming these challenges is important for using the full potential of these technologies.
Ultimately, the synergy between these cutting-edge tools and data quality drives us toward a future where information is reliable but also rich, timely, and essential for informed decision-making and innovation. It’s about leveraging technology to empower us with invaluable, high-quality data for a better future.