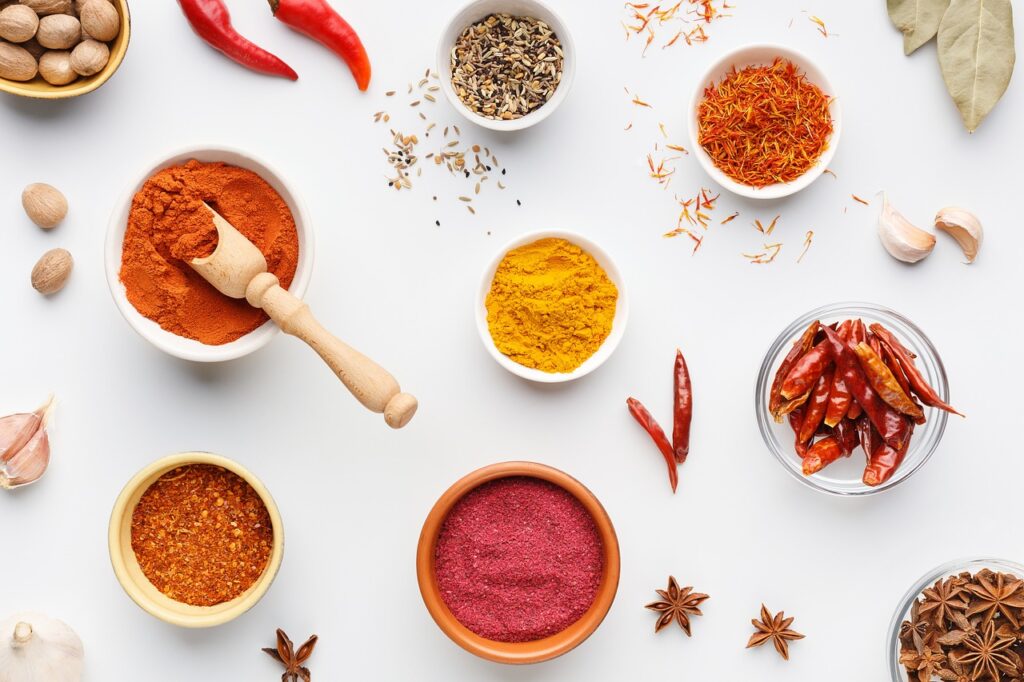
Image by atul prajapati from Pixabay
February’s Enterprise Data Transformation Symposium, hosted by Semantic Arts, featured talks from two prominent members of pharma’s Pistoia Alliance: Martin Romacker of Roche and Ben Gardner of AstraZeneca.
It’s been evident for years now that the Pistoia Alliance, organized originally in 2008 by Pfizer, GlaxoSmithKline and Novartis for industry data sharing purposes, has been seeing benefits from its members’ efforts, particularly when it comes to learning from each others’ new best practices.
As Gardner pointed out, science in general has been nudging pharma in the direction of knowledge graph adoption, for good reason. Scientists need to share their findings and scale up their collaborations.
Pharma, considering that it must bring together comprehensive knowledge of the body, mind, spirit and chemistry, may be the most knowledge-intensive industry around. No wonder that pharma pioneered the notion of findable, accessible, interoperable and reusable (FAIR) data in 2016.
FAIR was a necessary step for the industry to be able to make progress on the scientific data sharing front. Data sharing in the most reusable way requires contextualization, so that compatible contexts can complement and contrast effectively with one another.
In that sense, scientists need a contiguous, logically connected, and ordered landscape to explore together with the help of machines, so they can conduct their research in a fully collaborative, unimpeded, expedited fashion. That landscape – contextualized via knowledge graphs designed to snap, grow and evolve together– is the polar opposite of disconnected, opaque, difficult to work with data in silos.
Use case: Food pairing
The Symposium also highlighted other knowledge graph use cases, some of which wouldn’t immediately jump to mind, at least not for me.
Take the notion of food pairing, for example. Foods that provide a novel multisensory experience are a big deal for consumer products companies facing their own challenges on how to innovate and stay relevant. Those companies need to decide which new products to offer, as well as which products to discontinue when ingredient blends fall out of favor.
What’s not as obvious is how big the gap is that relational databases alone are leaving for graph databases to fill when it comes to food pairing trend intelligence. Or how much opportunity lies in a straightforward, methodical knowledge graph-based approach to a food pairing software as a service. Or how other, not-yet-explored opportunities lie in adjacent spaces such as fragrance pairing.
Stratos Kontopoulos, a knowledge graph engineer at FoodPairing AI, described during his presentation the ways in which the company’s in-house data collecting, sifting, contextualizing and analyzing process helped Unilever Knorr, who had partnered with the World Wildlife Fund to uncover innovative ways to establish a more diverse diet.
For the project, Foodpairing collected and evaluated 3.8 million recipes, selecting ten percent of these that met the project’s vegetable-only criteria. Then they brought this data from the selected recipes together with a unifying knowledge model in a knowledge graph.
With the graph and its in-house sensory/food preparation and experience model of taste, texture and smell, etc., Foodpairing was able to effectively disambiguate and characterize the sensory experience of different ingredient blendings and uncover trends that were difficult or impossible to discover at scale using older methods. As the company expands its reach, the articulation and precision of these consumer taste graphs will surely open up new avenues of product innovation exploration.
Use case: Residential real estate
During his presentation, chief architect and co-founder Tavi Truman of RocketUrBiz said real estate agents deal with a lot of manual or near-manual processes. Much of their activity involves capturing accurately and appropriately responding to what was said by buyers, sellers or intermediaries during all parts of the marketing and sales process.
Ironically, those processes are still manual when it comes to the Multiple Listing Service (MLS), email, real-time conversations and 61 different categories of other software agents might be using. The problem is that the data in those applications is siloed and disconnected, so the user experience is also fragmented and wasteful. Sound familiar? That user experience fragmentation is rooted in neglected legacy data architecture.
That’s why Truman and his co-founder, CEO Debra Schwartz, focused their company’s solution on a transformed, knowledge graph-based architecture to be able to unify the user experience.
Key to unifying the user experience has been linguistically clear and logically consistent modeling of marketing, sales and transactions using common logic and Basic Formal Ontology (BFO), an ISO standard, in conjunction with Yet Another Workflow Language (YAWL). Interoperability at the data layer in this sense unifies the user experience.
The company also supports application development in C#, Java or Python so that customers can harness the power of its TrueSpark interoperability platform for the full unified experience.
The importance of modular architecture design
When wrapping up the Symposium, Semantic Arts President Dave McComb noted that many of the talks alluded to how critical a modular approach to modernization is. Foodpairing AI started small but incrementally added novel ingredient trends, combinations and product insights. RocketUrBiz, similarly, has modeled its interoperability platform and workflows a step at a time over years.
The strategy of adoption, as SA’s Mike Atkin pointed out, is an incremental one too. What’s implied here is that the projects the Symposium highlighted require consistent support of those who are committed to long-term transformation.