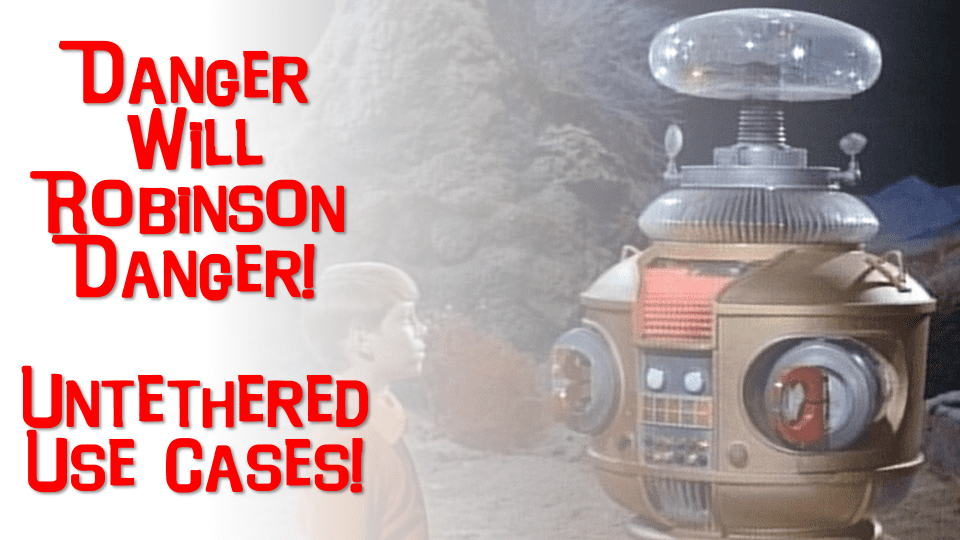
Client: “We’ve identified over 60 use cases!”
Schmarzo: “You might as well have zero…”
I always get very concerned when I see organizations that jump right into the use case inventory process without first considering the organization’s key business initiatives. Every organization has a laundry list of use cases. They tend to be the dumping grounds for organizations that lack a strategic approach for determining where and how to leverage data and analytics to drive business outcomes.
Organizations don’t fail from a lack of use cases but fail because they have too many.
I’ve talked, lectured, and written several times – heck, I even wrote “The Economics of Data, Analytics, and Digital Transformation” – about the economic ramifications of a use case-centric approach to building the organization’s data and analytic assets. A use case-centric approach (Figure 1) unleashes the unique economic characteristics of data and AI by:
- Reusing the same data set – an economic asset that never depletes and never wears out – across an unlimited number of use cases at zero marginal cost and
- Creating data and analytic assets (data products and AI apps, respectively) that appreciate, not depreciate in value, the more they are used.
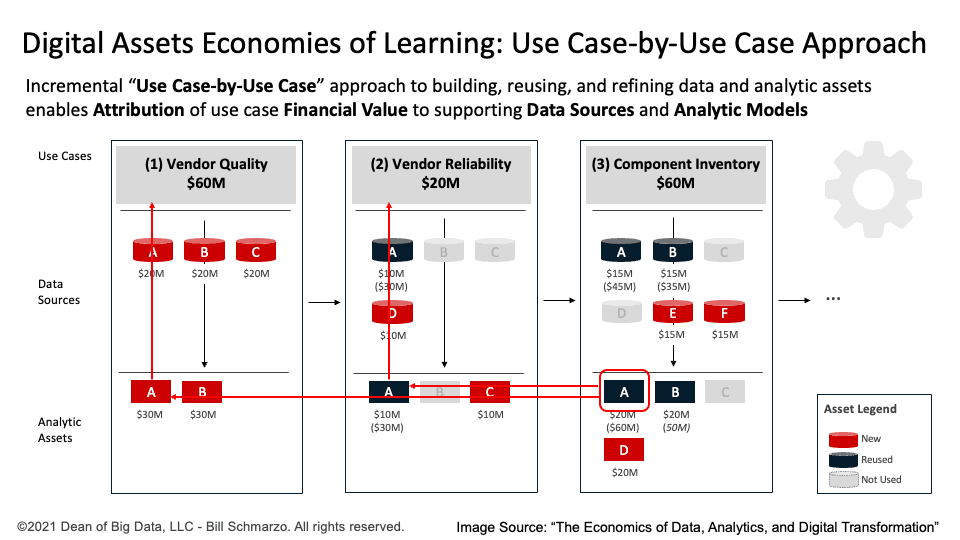
Figure 1: Source: “The Economics of Data, Analytics, and Digital Transformation”
However, a use case-centric approach must start by identifying and understanding the organization’s strategic business initiatives. Once we understand the organization’s key business initiatives, we can decompose them into supporting use cases. This yields the following benefits:
- If it is truly a business initiative that has critical and quantifiable business value, then there is likely someone on the executive leadership team who owns that initiative. You have immediately found a “friendly” on your AI and data journey.
- Use cases tied to that business initiative have a sense of urgency and prioritization, given their ability to enable and optimize that business initiative.
- Use cases tied to the same business initiative likely share the same data, which allows us to engage the Data Economic Multiplier Effect and crank out a stream of related use cases with quantifiable value more quickly and at less risk
Data Economic Multiplier Effect is the accumulation of attributable and quantifiable “value” from applying a data set against multiple use cases.
Trying to sort out a random dump of use cases is futile – they lack senior management interest, likely don’t share the same data sets, and it’s hard to rally support around a bunch of untethered use cases.
Untethered Use Cases are business and operational use cases not linked to or directly supporting an organization’s key business initiatives.
Tethering or linking use cases to an organization’s key business initiative is the best way to ensure that you focus your AI, data science, and data management resources on those use cases that matter to the business. It is also the best way to ensure the collaboration between the business and data science teams on our AI journey to deliver more relevant, meaningful, responsible, and ethical business and operational outcomes.
The AI Journey from Business Initiative to Use Cases
Let’s start this journey with some definitions to ensure everyone is on the same page.
- A Use Case is a cluster of Decisions around common KPIs or metrics that deliver a well-defined business or operational outcome supporting an organization’s key business initiative.
- A Business Initiative is a cross-functional effort typically taking 9-12 months, with well-defined business metrics supporting the organization’s key business and operational objectives.
Put another way, a business initiative is a high-level strategic goal that aligns with the organization’s vision and mission, while a use case is a specific and measurable way to achieve that goal. For example, a targeted business initiative might be to increase customer retention by X%, supported by a series of customer, product, and marketing-specific use cases (Figure 2).
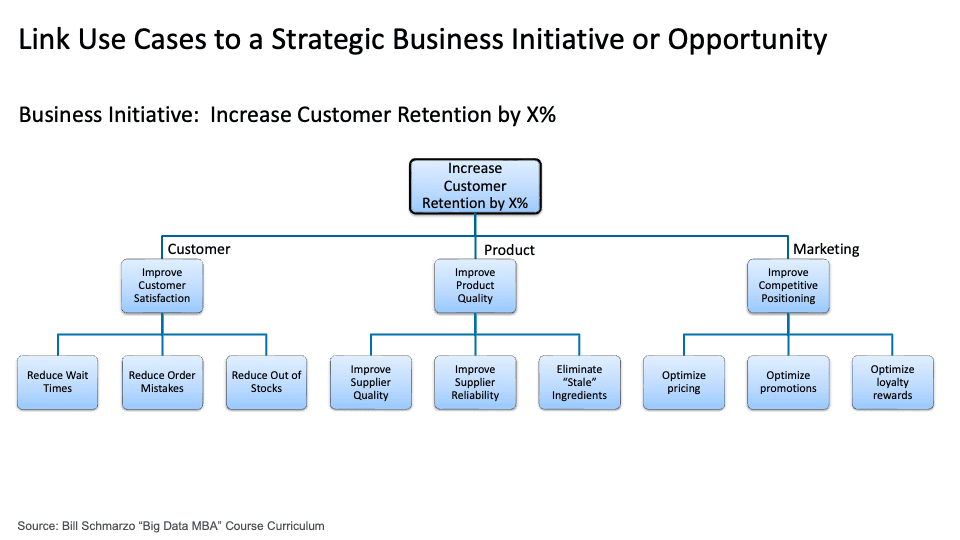
Figure 2: Tethering Use Cases to a Strategic Business Initiative
The process of going from a business initiative to identifying, validating, valuing, and prioritizing the use cases that support that business initiative is relatively easy. It’s not like I’m asking you to design and train a neural network. It just requires a collaborative engagement methodology that leverages the disciplines of data science, design thinking, and economics to unleash and corral the creative juices of the business and data science teams in support of the business initiative. I think I know where you can learn more about such a methodology (Figure 3).
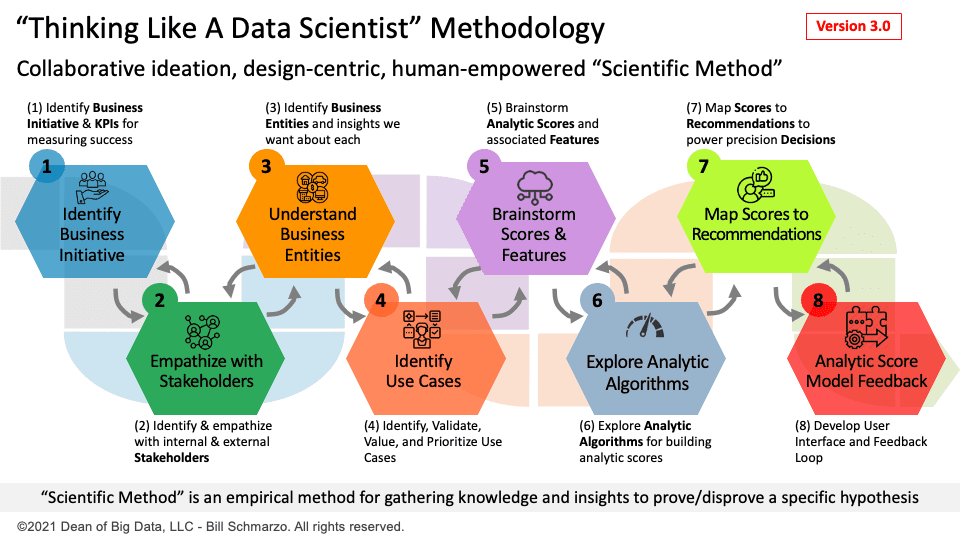
Figure 3: “The Art of Thinking Like a Data Scientist”
Unleashing the Economic Value of Use Cases
When we combine a use case-centric implementation approach with the unique economic characteristics of data and analytics, you get the Schmarzo Digital Asset Economic Valuation Theorem and my effort to win a Nobel Prize in data economics (Figure 4).
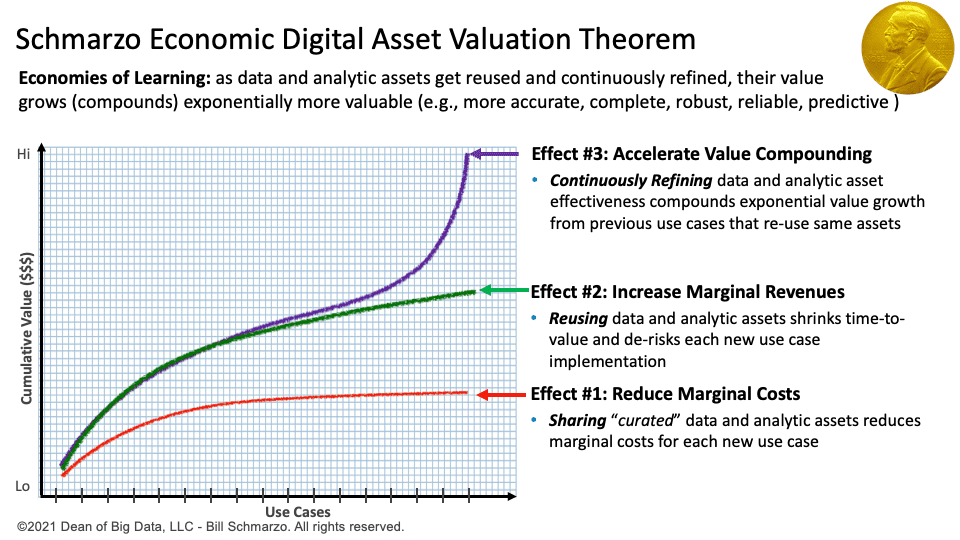
Figure 4: Schmarzo Economic Digital Asset Valuation Theorem
The Schmarzo Digital Asset Economic Valuation Theorem is a framework that explains how companies can increase the value of their data and analytics assets by aligning their development with the organization’s sources of value creation. The theorem is based on the concept that data and analytics are unique types of digital assets that have the following characteristics:
- Data never depletes, never wears out, and can be reused at zero marginal cost.
- Analytics can be continuously improved through learning and feedback and can be shared and reused across different use cases.
- Data and analytics can create network effects, where the value of the assets increases as more users and applications use them.
The theorem states that organizations can realize three effects or benefits from the sharing, reuse, and continuous refinement of their data and analytics assets:
- Marginal Costs Flatten: The marginal costs associated with reusing data and analytic models flatten as the reuse increases, leading to lower costs and higher profits.
- Marginal Value Grows: Sharing and reusing data and analytic models accelerate future use case time-to-value while de-risking implementation, leading to faster and more reliable value creation.
- Economic Value Accelerates: Analytic model refinement (improving analytic model accuracy, precision, and recall) lifts the economic value of all associated use cases that use the same analytic module, leading to exponential value growth.
Summary: Business Initiative-linked Use Cases
Tethering or linking use cases to an organization’s key business initiative is the best way to ensure that you focus your AI, data science, and data management resources on those use cases that matter to the business. It is also the best way to ensure the collaboration between the business and data science teams on our AI journey to deliver more relevant, meaningful, responsible, and ethical business and operational outcomes.
This also unleashes the benefits of the Schmarzo Digital Asset Economic Valuation Theorem by:
- Guiding organizations in prioritizing their data and analytics initiatives based on the potential value creation and reuse opportunities.
- Encouraging organizations to adopt a use case-by-use case deployment approach that exploits the economies of learning through rapid experimentation and feedback loops.
- Motivating organizations to break down data silos and avoid orphaned analytics, which are the great destroyers of the economic value of digital assets.
- Enabling and empowering organizations to measure and optimize their data and analytics assets’ return on investment (ROI) using objective metrics.