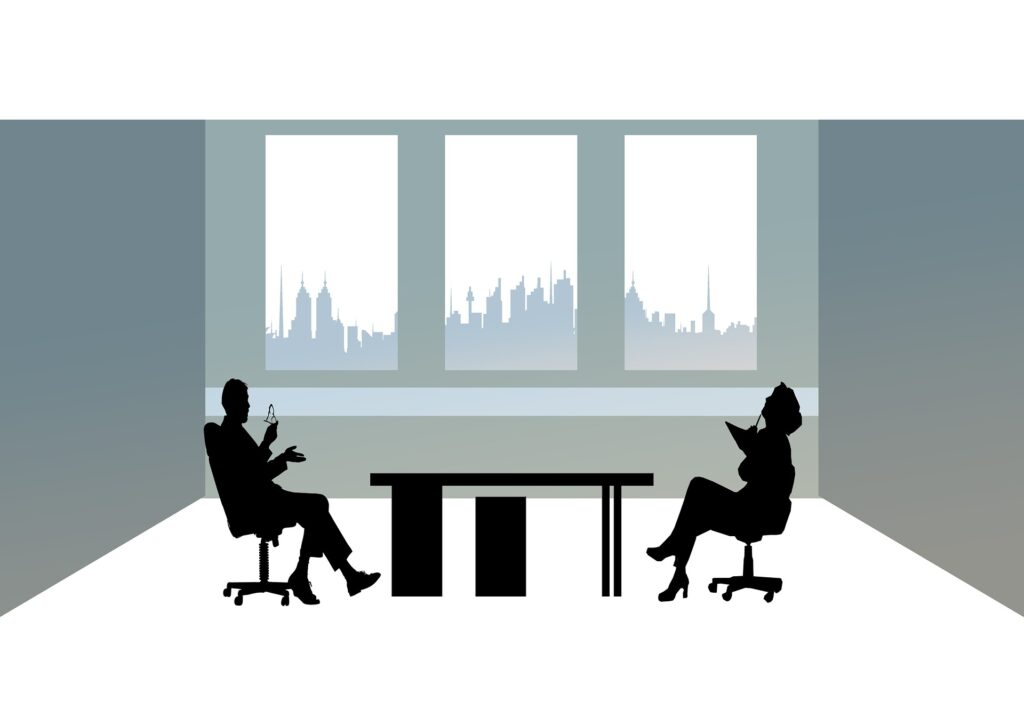
Freelance writer Christopher Beam is a skeptic of sorts. But in a May 2023 piece for Bloomberg on the aftermath of the crypto winter, Beam admitted he finally bought some Bitcoin, in April 2021. A friend had talked him into doing so. The Bitcoin he bought then lost 3/4s of its value. He bailed out a year later and no longer owns any cryptocurrency.
In the article, Beam profiled some of the crypto faithful. Their similarities are more telling than their differences. Near the end of the piece, he noted that his barber David had heard that “AI crypto” (not further specified) would be the next big thing. Beam told David he’d follow up. He still hasn’t.
SaaS–now available with GPT
Crypto needs a lot of help to move forward, as we all know. “AI” will not solve its most troubling problems.
Similarly, software as a service has some deeply rooted issues. But instead of confronting those issues, major SaaS providers have been announcing large language model (LLM) enhancements to their product lines at the Spring user conferences they’ve been staging. Tableau, for example, is now harnessing Salesforce Einstein GPT. Thankfully, Salesforce has put data governance guardrails in Einstein GPT, according to TechTarget’s Eric Avidon. The claim is that the external data Einstein GPT harnesses is trustworthy, and its data privacy is enhanced.
But the messaging surrounding these product enhancements is reminiscent of consumer food product labeling. Instead of “with DHA Omega 3” or somesuch, the SaaS enhancement messaging is along the lines of “Now with GPT.”
Paying software middlemen instead of investing in AI
Those vendors have become the middlemen in-between companies and their own data. That’s a problem because AI works best when it has unfettered access to a semantically connected, siloless flow of relevant internal plus external data sources.
Instead of committing to their own AI-friendly data foundations, enterprises are back on the sidelines. Andrew Ng described the dilemma most enterprises were facing in a June 2022 interview published in the MIT Sloan Management Review:
“I see lots of, let’s call them $1 million to $5 million projects, there are tens of thousands of them sitting around that no one is really able to execute successfully. Someone like me, I can’t hire 10,000 machine learning engineers to go build 10,000 custom machine learning systems.”
In 2023, it seems, many have just shelved their in-house AI initiatives entirely, not to mention the deeper data integration and enrichment efforts. Many enterprises are just sitting back, letting others determine how they’ll be collecting data and using “AI,” keeping the tech at arms length.
As it turns out, SaaS also stands for “silo as a service,” and companies are renting access to more and more of these multi-tenant silos.
Other data issues with development
Application-centric development typically starts with functionality: What’s the app going to do? The mentality behind providing this functionality focuses on coding the app.
When developers use this method, the data is an afterthought, because both designers and those funding the development aren’t thinking much in data terms. The app includes forms with implicit assumptions behind the forms for the data that end users are expected to input. Frequently, the end-user has to intuit how to fill the cryptic forms out to generate helpful data for the app functions. Additionally, the user has to participate to generate behavioral data specific to the app.
If and when the organization using the app decides that the insights coming from the app are insufficient, the knee-jerk response is to seek out another app that may do better. But the root problem is how to collect better data at scale.
There are always apps that vendors claim may do better.
Data-centric development and management commitment—not a new concept
For a couple of decades, API-driven app development has made it somewhat easier to share and reuse data and code. Google Maps was an early example.
What’s been notable about Maps has been serious data management from its inception. You could consider it an early data-as-a-product offering.
Maps required a major up-front investment and a continual level of long-term commitment to a data lifecycle tied to a vertically integrated offering users have grown heavily dependent on. Google decided to do its own data collection from scratch. Its cars still routinely scan the paved public landscape. Maps and Earth go hand in hand. Five million websites use Maps. 154 million users take advantage of it every month.
More data skeptics, including some at Google
The real power in applications derives from taking data seriously, whether AI-enhanced or not. The AI mantra has become tiresome. Some of the Google staff at the company’s annual I/O event, according to Jennifer Elias of CNBC, were rolling their eyes as execs kept mentioning the term.
Keep in mind that the skeptics have been proven correct in the case of AI as well as crypto. In an April 2023 paper, a group of researchers led by Asst. Prof. Sanmi Koyejo at Stanford reported on the abilities of large language models as they grow larger. They concluded that LLMs aren’t more than the sum of their parts. That is, the mystical emergent properties some have claimed for LLMs don’t really exist. LLMs, stable diffusion, and other similar advances aren’t magic. There is no magic bullet.