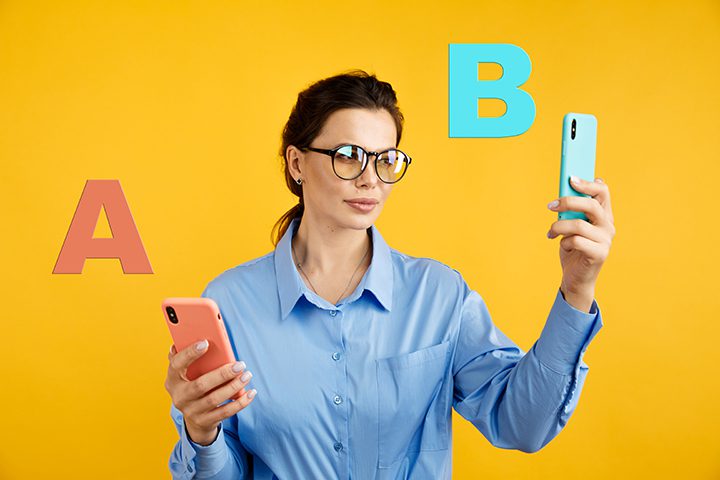
Andrea Polonioli & Ciro Greco
Leading companies have been seizing the unprecedented opportunities offered by the web to test hypotheses quickly by using controlled experiments, typically called A/B tests. A/B tests are now ubiquitous: when you open up your Netflix app, there is a good chance you are part of one or more experiments while you are browsing content. This is also reflected by the increasing number of A/B tests being featured in corporate-affiliated papers published in leading conferences such as SIGIR, KDD, WSDM or RecSys.
The purpose of A/B testing is usually twofold: on the one hand, companies use it to optimize their business using feedback based on real data, on the other, AB testing is a source of knowledge for companies who can use it to understand what works, what doesn’t and to what extent. In other words, A/B testing is an invaluable tool to establish causal relationships between business initiatives and business outcomes.
As we all know, the effectiveness of live A/B testing is grounded in randomized trials: a portion of users are randomly assigned to the control and another portion of users are randomly assigned to the target condition. And of course, randomization needs to be paired with controlling as much as possible with other factors that we know may play a role (e.g. language, geography, seasonality, time of the day, etc).
However, we wish to draw the attention to a remarkable blind spot in company-sponsored research on user and consumer behavior. As it turns out, some really useful econometric techniques are way too often overlooked in modern data science in spite of their value when it comes to drawing causal inferences.
This piece argues that there is plenty of value in adopting quasi-experimental design based on causal inference in industry settings when A/B testing based on random assignment is infeasible.
Our argument here starts by observing that, from an industry perspective, there are non-trivial constraints on A/B testing.
Let’s take an example that we know well: ecommerce. Ecommerce is usually a very fertile ground for A/B testing, since ecommerce websites provide the perfect environment for it.
That’s because a variety of components of the website can be tested extensively against each other with a process that in on many occasions the consists of switching on and off a certain component of the website and assigning a certain portion of the traffic to a version of the website in which such component is on and a portion of the traffic to a version of the website where the component is off.
We can do that with search functionalities. For instance, we can test search personalization by assigning half of the visitors of the websites to a version of the website where search personalization is active, while assigning the other half to a version where no search personalization is available.
However, there are also things that an ecommerce company cannot really A/B test — or at least should be very careful in doing.
For example, let’s say that you want to establish search attribution (how much search causally drives conversions on your website) beyond any reasonable doubt, that is how much search causally drives conversions on your website. Switching off your entire search box for a significant portion of users is likely to harmfully impact business revenue. Similarly, no one has ever tested Black Friday deals to address how much incremental revenue is driven by the Black Friday sale. It isn’t really possible.
So, if we cannot make experiments, how can we determine whether certain capabilities are really responsible for certain business outcomes? Does this mean that ecommerce companies cannot make any causal inferences in cases where A/B testing doesn’t look like a feasible solution?
Not necessarily. Causal inference is actually best seen as an umbrella term encompassing a number of different approaches, whereby random assignment is just one of them.
Regrettably, however, over the past years, little to nil attention has been paid to the availability of alternative approaches to establish causality when A/B tests based on randomized experiments are not an option due to feasibility constraints. Yet, econometrics, arguably data science’s sister, offers techniques and procedures that can prove especially useful to establish causality.
We introduce here the concept of Quasi A/B test to mirror the use of
Quasi-Experiment in the social sciences. In those contexts, the term quasi-experiment refers to an experiment in which units are not assigned to conditions randomly. In our context of company-sponsored research, we refer to a Quasi A/B test as an attempt to draw causal inferences when random assignment and A/B testing are infeasible.
Quasi A/B tests essentially mimic experimental conditions in which some subjects are exposed to treatment and others are not on a random basis. They do so by applying some designs, methodologies and tools for causal inference and from econometrics. These other methods which are available to data scientists and are drawn from the econometrician’s toolbox encompass tools such as regression discontinuity and difference in differences.
We contend that Quasi A/B tests based on causal inference should receive more attention in the context of company-sponsored research on user and consumer behavior. This is also in light of evidence showing that randomized experiments like traditional A/B tests don’t produce overly dissimilar results from other econometric methods based on causal inference.
Make no mistake: we are not arguing that Quasi A/B tests based on causal inference will be a panacea. After all, these tools may not always be available, suitable or the best tool to test relevant hypotheses, but when A/B tests aren’t really feasible, companies should consider resorting to these approaches whenever possible.
But the key message we’d like you to take home is that there will be huge rewards for those choosing to expand their data science toolbox by including methods from econometrics.
To see this, it can be useful to consider a parallel between experimentation based on company-sponsored A/B tests and the use of randomized controlled trials (RCTs) in fields such as education or health.
In those contexts, RCTs would be sometimes unfeasible due a number of factors, including ethical considerations. Imagine for example an experiment aimed at comparing student outcomes in districts that received money with student outcomes in similar districts that did not receive a random allocation of money. A controlled experiment would provide the most rigorous causal estimate of spending on student outcomes of interest. Yet such an experiment would remain a thought experiment at best given the ethical considerations it entails. This helps appreciate the importance of adopting quasi-experimental research designs. When RCTs are unfeasible or unethical, researchers resort to causal inference.
The inability to master Quasi A/B test designs based on causal inference would preclude researchers from the opportunity to explore causality in a number of relevant settings, preventing them from getting valuable, most useful insights.
Researchers in medicine and the social sciences understood the value of quasi experimental design and causal inference a long time ago. It is high time that researchers involved in company-sponsored research follow in their footsteps.
Written by Andrea Polonioli, Product Marketing Manager, Coveo, and Ciro Greco, Vice President of Artificial Intelligence, Coveo.