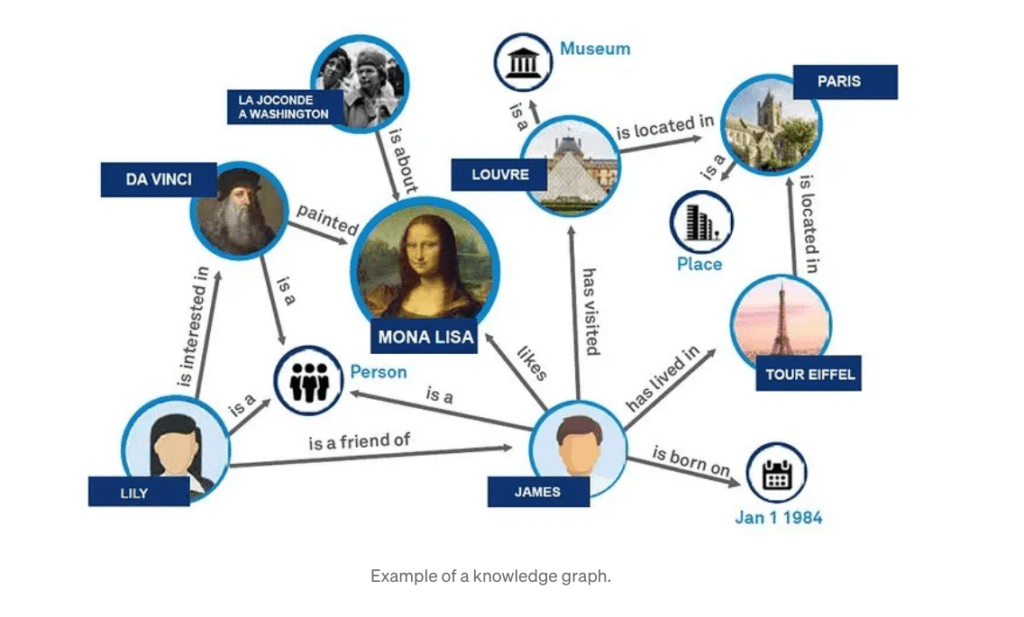
Background
Retrieval Augmented Generation(RAG) is an approach for enhancing existing LLMs with external knowledge sources, to provide more relevant and contextual answers. In a RAG, the retrieval component fetches additional information that grounds the response to specific sources and the information is then fed to the LLM prompt to ground the response from the LLM(the augmentation phase). Relative to other techniques (such as fine tuning), RAG is cheaper. It also has the advantage of reducing hallucinations by providing the LLM with additional context – making RAG a popular approach today for LLM tasks such as recommendations, text extraction, sentiment analysis etc.
If we break this idea down further, based on the user intent, we typically query a vector database. Vector databases use a continuous vector space to capture the relationship between two concepts using a proximity based search. Vector databases find and retrieve data based on the similarity of data points, represented as vectors in a multi-dimensional space.
An overview of vector databases
In a vector database, data—whether it be text, images, audio, or any other type of information—is transformed into vectors. A vector is a numeric representation of data in a high-dimensional space. Each dimension corresponds to a feature of the data, and the value in each dimension reflects the intensity or presence of that feature. Proximity-based searches in vector databases involve querying these databases by using another vector and searching for vectors that are “close” to it in the vector space. The proximity between vectors is often determined by distance metrics such as Euclidean distance, cosine similarity, or Manhattan distance.
When you perform a search in a vector database, you provide a query that the system converts into a vector. The database then calculates the distance or similarity between this query vector and the vectors already stored in the database. Those vectors that are closest to the query vector (according to the chosen metric) are considered the most relevant results.
The use of proximity-based searches in vector databases is particularly powerful for tasks like Recommendation Systems, Information Retrieval and Anomaly detection etc
This approach enables systems to operate more intuitively and respond more effectively to user queries by understanding the context and deeper meanings within the data, rather than relying solely on surface-level matches.
However, vector databases have some limitations based on proximity searches for instance data quality, the ability to handle dynamic knowledge and transparency.
Limitations of RAG
Depending on the size of the document, there are three broad classes of RAG: if the document is small, it can be accessed in context; if the document is large (or there are multiple documents), smaller chunks are generated at the time of the query and these chunks are indexed and accessed to respond to the query.
Despite its success, RAG has some shortcomings.
There are two main metrics to measure the performance of RAGs, Perplexity and Hallucination,
Perplexity represents the number of equally-likely next-word alternatives available during text generation. I.e. the degree to which a language model is “perplexed” by its choices.Hallucination is a statement made by AI that is untrue or imagined.
While RAG helps to reduce hallucination, it does not eliminate it. If you have a small, concise document you can reduce perplexity (because the LLM has few options) and reduce hallucination (if you ask it only about whats in the document). The flipside is of course that a single, small document lends to a trivial application. For more complex applications, you need a way to provide more context.
For example, consider the word ‘bark’ – we could have at least two different ciontexts
Tree context: “The rough bark of the oak tree protected it from the cold.”
Dog context: “The neighbor’s dog will bark loudly whenever someone passes by their house.”
One way to provide more context is to combine the RAG with a knowledge graph (a GRAPHRAG)
In a knowledge graph, these words would be connected to their relevant contexts and meanings. For example, “bark” would have connections to nodes representing both “tree” and “dog”. Additional connections could indicate common actions (e.g., “protect” for tree bark, “make noise” for dog bark) or attributes (e.g., “rough” for tree bark, “loud” for dog bark). This structured information allows a language model to select the appropriate meaning based on other words in the sentence or the overall topic of the conversation.
In next sections, we will see the limitations of RAG and how GRAPHRAG addresses these limitations
Image source: