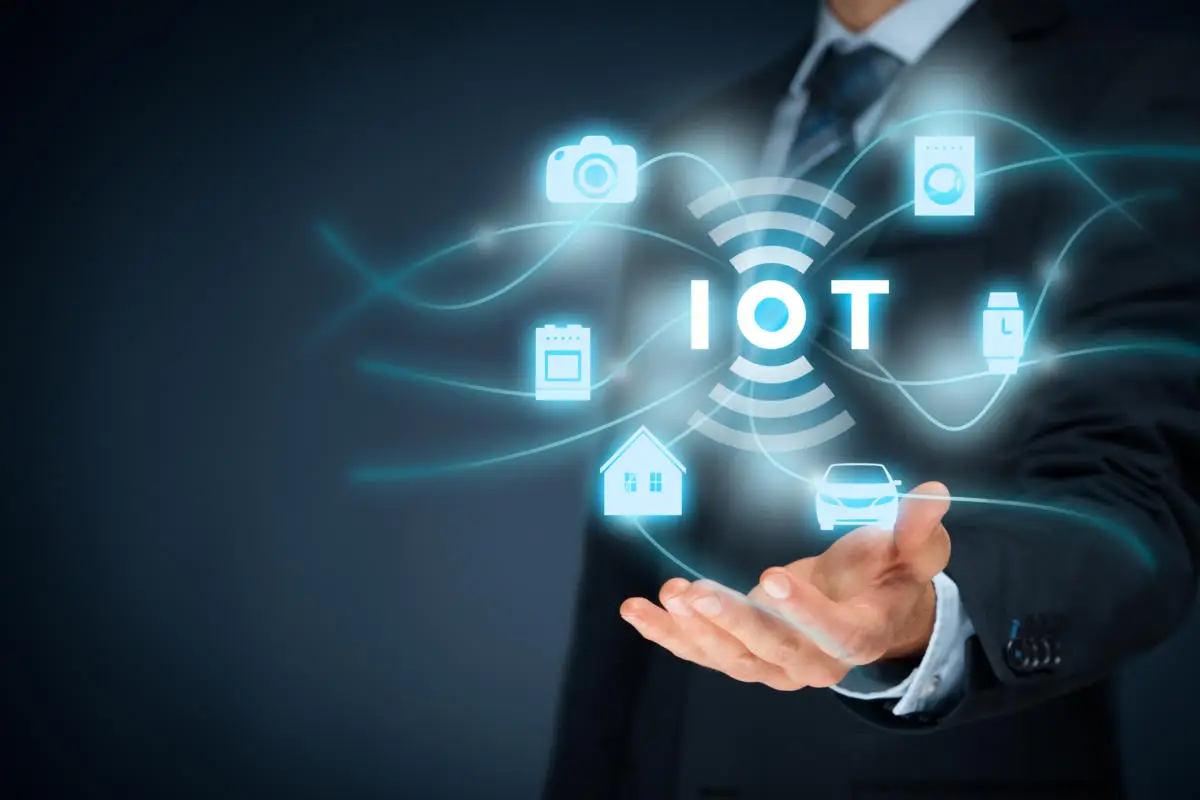
In today’s tech-driven landscape, the proliferation of Internet of Things (IoT) devices has revolutionized how businesses collect and utilize data. The interconnectivity of these devices has created an unprecedented influx of data, requiring efficient management strategies to harness its full potential.
Understanding IoT data
IoT devices span a vast array, from sensors in machinery to smart appliances and wearable gadgets. These devices continuously generate data streams encompassing diverse types, such as sensor readings, location information, user interactions, and more. Harnessing this data presents unparalleled opportunities, but it also poses significant challenges.
The challenge of volume, velocity, and variety
The crux of IoT data management lies in handling the 3Vs: Volume, Velocity, and Variety. The sheer volume of data inundating systems demands robust storage and processing capabilities. The velocity at which data arrives requires real-time or near-real-time processing to derive timely insights. Moreover, the variety of data formats and sources complicates integration and analysis.
Strategies for efficient IoT data management
- Scalable infrastructure using cloud and edge computing:
IoT data often overwhelms traditional infrastructure. Cloud platforms like AWS IoT, Microsoft Azure IoT Hub, or Google Cloud IoT provide scalable storage and computing resources. For instance, AWS IoT Core allows seamless integration, storing, and processing of IoT data at scale, enabling businesses to adapt to fluctuating data volumes.
Additionally, edge computing brings computation closer to the data source, reducing latency and bandwidth usage. Consider a factory employing edge devices to analyze machinery data locally, sending only critical insights to the cloud for further analysis. This minimizes latency for real-time decision-making.
- Data filtering and prioritization:
Filtering data at the source ensures that only relevant information is transmitted. This is crucial in scenarios where bandwidth is limited or costly. For instance, IoT sensors in agriculture collect various data points. Filtering out non-critical data allows farmers to focus on essential factors like soil moisture levels or temperature variations, optimizing crop yields.
Prioritizing data based on urgency or importance ensures that critical insights are addressed promptly. In healthcare, wearable devices monitoring vital signs prioritize sending emergency alerts to healthcare providers, providing immediate attention when necessary.
- Robust security measures:
Securing IoT data is paramount due to its sensitivity. Employing encryption methods like AES (Advanced Encryption Standard) or TLS (Transport Layer Security) safeguards data during transmission and storage. For example, encrypted communication protocols in smart homes secure user data transmitted between smart devices and central hubs, preventing unauthorized access.
Access controls and authentication mechanisms prevent unauthorized access to IoT devices. Multifactor authentication ensures that only authorized personnel can access and manage IoT devices or data, enhancing overall security.
- Streamlined data integration and interoperability:
Integration platforms and standardized protocols facilitate seamless communication and data exchange among diverse IoT devices. For instance, MQTT (Message Queuing Telemetry Transport) protocol enables efficient data transmission between IoT devices and servers in industrial IoT applications, ensuring interoperability.
Implementing APIs and middleware solutions allows different IoT devices to communicate and share data, creating a unified ecosystem. This is evident in intelligent cities where various IoT devices, such as traffic sensors and environmental monitors, seamlessly transfer data to optimize city operations.
- Predictive analytics and AI-driven insights:
Machine learning algorithms and predictive analytics glean actionable insights from IoT data. These tools enable predictive maintenance in industries like transportation, where IoT sensors on vehicles predict potential component failures, allowing proactive maintenance, minimizing downtime, and optimizing operations.
AI-driven anomaly detection in IoT data helps detect irregular patterns, flagging potential security breaches or operational issues. For instance, in the energy sector, AI algorithms analyze IoT sensor data from power grids to identify anomalies indicative of potential failures or cyber-attacks, allowing preemptive measures to be taken.
The future of IoT data management
As IoT adoption proliferates across industries, the future of IoT data management is poised for evolution. Advancements in edge computing, data automation, AI-driven analytics, and 5G technology will further enhance data processing capabilities, enabling more sophisticated real-time applications.
- Advancements in edge computing:
The evolution of edge computing will revolutionize IoT data management. Edge devices with enhanced processing capabilities will perform complex computations locally, reducing latency and dependency on centralized cloud systems. For instance, edge AI chips in autonomous vehicles will process vast amounts of sensor data in real time, enabling faster decision-making without relying extensively on cloud servers.
- 5G technology integration:
The integration of 5G technology will significantly impact IoT data management. Its ultra-fast, low-latency networks will enable seamless transmission of large volumes of IoT data. For instance, remote surgeries utilizing IoT devices and 5G connectivity in healthcare will rely on instant, high-bandwidth data transmission to ensure precision and real-time responsiveness.
- AI-driven analytics and edge AI:
The convergence of AI-driven analytics and edge AI will empower IoT devices to perform more sophisticated data processing and analysis at the device level. For example, smart cameras with edge AI capabilities will analyze video data locally, recognizing objects, anomalies, or potential security threats without continuously relying on cloud-based AI systems, minimizing latency and bandwidth requirements.
- Blockchain for IoT security and data integrity:
Integrating blockchain technology will enhance security and data integrity in IoT ecosystems. Immutable ledgers and decentralized architectures will ensure transparent and tamper-proof data transactions. For instance, in supply chain management, blockchain-enabled IoT devices will track and validate each step of a product’s journey, ensuring authenticity and minimizing fraud.
- Federated learning for privacy-preserving insights:
Federated learning, a privacy-preserving technique, will gain prominence in IoT data management. This approach enables collaborative model training across multiple IoT devices without sharing raw data. For instance, in smart homes, IoT devices will collaboratively train AI models to improve energy efficiency without compromising individual user data privacy.
- Augmented Reality (AR) and IoT integration:
Integration of IoT with AR technologies will create immersive experiences and enhance IoT data visualization. For instance, in manufacturing, IoT sensors integrated with AR glasses worn by technicians will overlay real-time equipment performance data onto machinery, enabling quick diagnostics and maintenance.
- Quantum computing for complex IoT analytics:
The emergence of quantum computing will revolutionize complex IoT data analytics. Quantum computing’s immense processing power will handle intricate data analysis tasks, such as optimizing large-scale IoT networks or performing complex simulations for predictive maintenance in industrial settings.
- Evolving regulatory frameworks:
As IoT adoption grows, regulatory frameworks around data privacy, security, and interoperability will continue to evolve. Standards and regulations will ensure the ethical use, interoperability, and security of IoT devices and the vast amounts of data they generate.
Conclusion
Effectively managing IoT data is a cornerstone for businesses aiming to thrive in the data-driven era. By embracing scalable infrastructure, robust security measures, streamlined integration, and advanced analytics, organizations can leverage insights derived from IoT data to drive innovation, enhance operational efficiency, and stay ahead in an increasingly competitive landscape.