An edited interview with Devin Yaung, Senior Vice President, Group Enterprise IoT Products and Services at NTT Ltd.
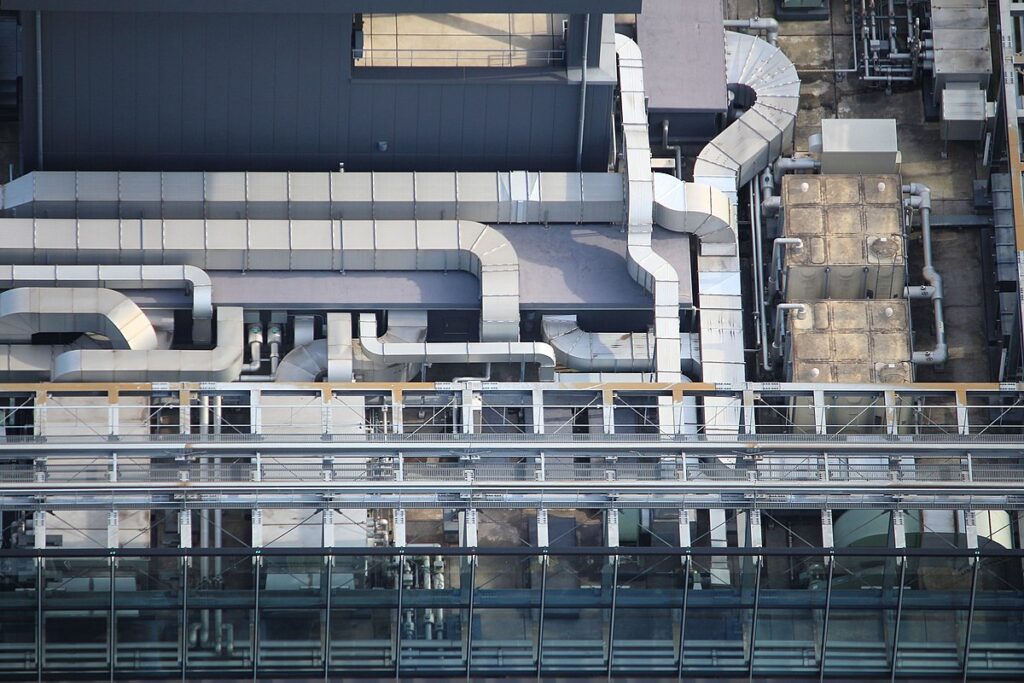
Photo from Wikimedia Commons
Devin Yaung is global lead of IoT for NTT Ltd. Yaung’s consulting background included stints at Accenture and PwC. He’s been at NTT for almost three years now.
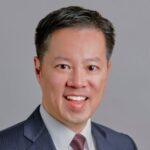
In this interview, Yaung and I discussed the practical approach that NTT takes with clients from a system integrator’s perspective. This interview took place after Upgrade 2024, an annual event that NTT hosted in San Francisco.
Background on NTT. Large conglomerate, Japanese phone company. DoCoMo East, NTT West focus on the domestic market. Everything outside of Japan–the global business of NTT–is now called NTT Data Inc.
NTT Data Inc is the traditional NTT Limited–data centers, submarine cables,network, cloud and edge business–combined with the systems integration and consulting capabilities of NTT Data to give clients the one-stop shop for the full stack.
The big picture on edge computing activities. The battle for the AI and machine learning future is going to be won at the edge.
There’s a lot of buzz around AI, for good reasons, machine learning, all these analytical platforms, but the issue is that it’s garbage in/garbage out as to what you’re using to train as input to these models.
A lot of these AI things are calculators–for now. And your calculators are only as good as your input. The data that is feeding these AI models, your ERP model, your analytics models is going to be the most important. When you’re training your model on imperfect data, you’re not going to have the best results.
The AI timeline and current challenges. AI is still in its infancy. What’s really changing things is the cost to do things. Years ago, the compute power wasn’t there, and the cost to train a model was extremely high.
Then you got more open source, and you got better compute, so that now it’s accessible to most enterprises, to you and I.
But the problem is that we’re running out of data to feed these models. For example, say I’ve invested a lot in enterprise resource planning (ERP) systems. So now I’m going to plan how to schedule my resources such as my work shifts, my raw materials, etc.
If I have no visibility into what my demand and inventory is, where my workers and raw materials are, it’s very hard to plan my resources. I don’t know what my customer demand is. I’m going to be very reactive every time there’s an order. I may need to put in another shift.
But if I knew how inventory was flowing, and I could use AI and all these things to model it out, I could start forecasting demand. I determine the need two shifts, for example, and I can plan months ahead, and I need these raw materials, and I can be much more efficient. Having real time, trusted data can help on the back end for large investments in what we’re doing in AI and ML.
Thoughts on supply chain collaboration. If you think about every aspect of using data and AI to predict where things are going to be, technology is one part of it. There are platforms. There’s the edge that collects the data to feed these platforms, that then makes the recommendations.
But the hardest thing is that once you have that recommendation, you have to take action upon it. There’s all the systems integration and the integration into the backend ERP system to say, “These are the actions that need to be taken and those who are accountable to take that action.
Predictive maintenance example. Let me give you an example. Let’s take your car. I need an oil and filter change, or I need to change a tire. Unless you take action upon it, to say, “I’m going to actually do that,” all these alerts and notifications mean nothing.
So in an enterprise setting, when assigning the work, creating a trouble ticket, ordering the parts and then monitoring that the workflows have been kicked off, and seeing that all the work has been done satisfactorily–that’s when the data is actionable.
Most clients say, “I’m inundated with data. It’s like a nagging person, and I ignore 80 percent of the alerts that I do get because they’re not actionable.” Or, “I don’t trust that data. I’m going to go and verify.”
Not just data, but meaningful process change. As a core network company, you go back to the old days of FCAPS (an acronym for the five working levels of network management: fault, configuration, accounting, performance and security. See https://www.techtarget.com/searchnetworking/definition/FCAPS for more information.) The focus was correlating those alerts and looking for the root cause analysis. What does all this data mean?
What is the cause of the events and the alerts that I’m getting? From a data perspective, (1) collecting the data, to (2) making sense of it, (3) making sure that it’s trusted, and (4) making it actionable are the key four things.
But that’s just the technology viewpoint. What about the people involved? Until you can change the operating model, incentivizing the people to take action on it through a process change, nothing gets done.
Let’s say a water leak has sprung in the basement of a building. For the person dispatching a technician to fix the leak, their metrics are based on reducing overtime. “Wow, it’s Sunday night. I’m going to get in trouble because it’s going to be time and a half to come in on Sunday. I’m going to wait until Monday, when my metrics that I’m measured on–saving money on operations–is what matters.” And the whole time water is filling up the basement.
Are you tying the metrics of the people to the outcome?
Regulatory issues. One of the things that has been challenging is the whole regulatory security and governance environment. And regulation is one of the biggest things because it’s all about moving data. If it’s here in the United States, every state has its own requirements.
For instance, California and Illinois have very strong Health Insurance Portability and Accountability Act (HIPAA) laws. California has strong data privacy requirements, for the most part. We tend to start with GDPR as a baseline, and Europe has recently passed its AI Act. Are provisions like those going to be adopted in the States?
Companies who are thinking about using AI are saying, “I could use it today, but where’s the regulatory environment going to be? A year from now I may find out I’m in violation or another, and this may get me in trouble. That aspect is one of the biggest barriers to adoption.
Companies have to look at these kinds of things in all their dimensions, rather than just in a silo. If all you have is a point solution, that’s not very effective.
What transformation clients really want. Everyone has an edict–for example, digital transformation. Everyone is saying, “Go do something in AI because we don’t want to fall behind.” But because a lot of these things aren’t proven, clients tell us, “Prove this is going to work.” And once they succeed, then they can start adding on. It starts with a small pilot, and then it moves on.
Let’s take the example of predictive maintenance on exhaust fans in a building. There can be hundreds of exhaust fans at places like hospitals. The master mechanic at such a facility has to spend three hours a day walking the roof doing inspections–not only the fans but everything else they’re concerned with. You could put a vibration sensor on each fan to tell you when a bearing is broken, or a fan blade.
You could sensor it up, but then the client’s question is, “Will my master mechanic trust that dashboard to say that all exhaust fans are working fine, or is he going to say, ‘Maybe those sensors are wrong. I do need to walk up and inspect the fans every time.’ But then I’m not saving any money because I’m still hiring a third party to do all this preventive maintenance.”
The client says,”I need those responsible to get addicted to the data. I want them to trust it to the point where they can go do their regular work without having to do the visual inspection too.”
Logistics warehouse example. Another example involves working in a logistics warehouse. The pickers in the warehouse who were picking products were making errors because the products tend to look the same. We trained computer vision to validate that each order is correct so that operations can proceed.
Additionally, we were able to bring in our supply chain team and say, “Let’s redesign the whole pick process to reduce the multiple points of failure, and then use the data to validate that effort.” The client really liked that we were able, not only to bring in the technology, but to make people comfortable with the technology. We gave them reassurance, saying “This is not spying on you. This is an audit trail that makes things more efficient, so that we don’t have as much waste.”
This same company had piloted the use of robotics in the picking process, but no one had thought to coordinate the robotics with the work schedule, so that the robot had a human to hand the picked product off to. Obviously, they fixed that issue.
Sometimes we get so enamored with technology and we think it’s turnkey. And we forget the human aspect of it. Sometimes when watching what’s happening in a factory, I find myself wondering, “Is this the best use of someone’s time?” For example, watching a person take parts off a stamp and then hang them on a hanger for painting. Repeatedly. What’s going through that person’s head? Automation would free them up to do something more value-added.
Or hearing from someone whose job it is to pore over utility bills, when AI could analyze the bills and free the staff up to do something more strategic.
For now, humans will not be taken out of the equation.
Taking a more holistic, practical approach to transformation. We have to take a look at the full, end-to-end picture, what it is that we want to accomplish, and why we’re doing it. There has to be an ROI, or a business case. A tire company approached us and said, “We want to do tire as a service. We would love to be able to predict the tire wear.”
Someone had come up with a system to predict tire wear on trucks. But it cost $100,000, which was just a non-starter for tires on a truck.
We’ve had conversations about IoT-connected flip flops… This sort of conversation underscores that, just because you can do it, doesn’t mean it makes fiscal sense.