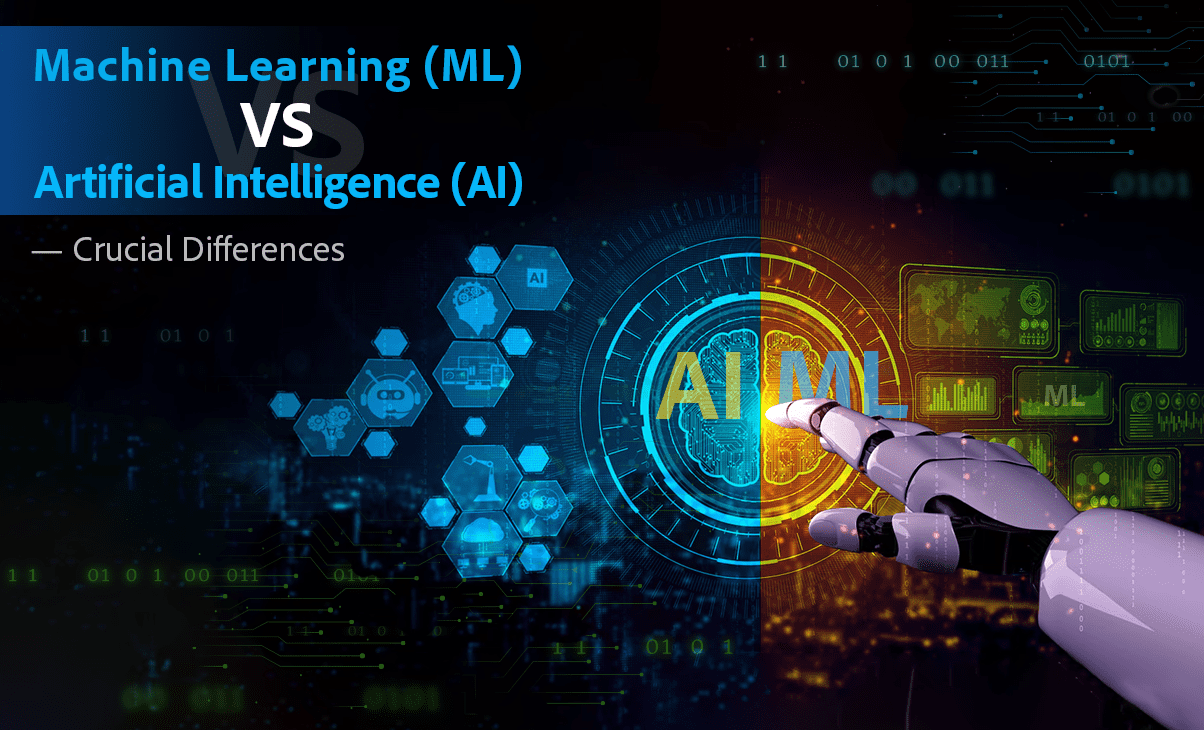
Machine learning (ML) and Artificial Intelligence (AI) have been receiving a lot of public interest in recent years, with both terms being practically common in the IT language. Despite their similarities, there are some important differences between ML and AI that are frequently neglected.
Thus we will cover the key differences between ML and AI in this blog so that you can understand how these two technologies vary and how they may be utilized together.
Let’s begin!
Understanding Machine Learning (ML)
Machine Learning (ML) is a subfield of Artificial Intelligence (AI) that automates data analysis and prediction using algorithms and statistical models. It allows systems to recognize patterns and correlations in vast amounts of data and can be applied to a range of applications like image recognition, natural language processing, and others.
ML is fundamentally about learning from data. It is a continuous method of developing algorithms that can learn from past data and predict future data. In this approach, ML algorithms can continuously improve their performance over time by discovering previously unknown or undetectable patterns.
Types of Machine Learning Algorithms
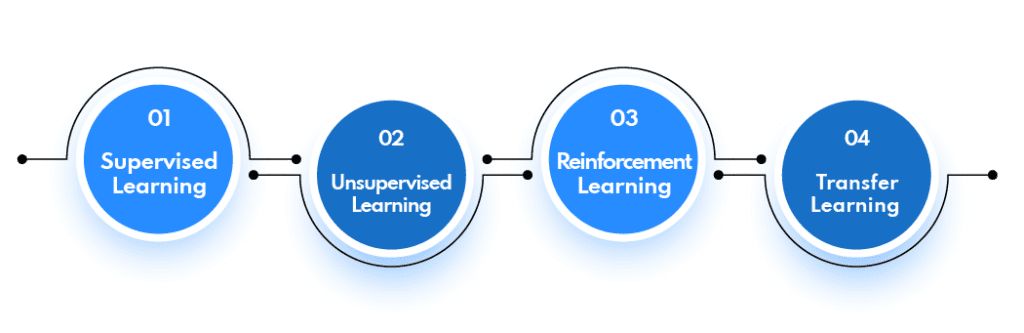
There are commonly 4 types of Machine Learning algorithms. Let’s know about each of them.
1. Supervised Learning
Supervised learning includes providing the ML system with labeled data, which assists it to comprehend how unique variables connect with each other. When presented with new data points, the system applies this knowledge to make predictions and decisions.
2. Unsupervised Learning
Unlike Supervised learning, Unsupervised learning does not need labeled data and rather uses several clustering methods to detect patterns in vast quantities of unlabeled data.
3. Reinforcement Learning
Reinforcement learning implies training an agent to act in a specific context by rewarding or punishing it for its actions.
5. Transfer Learning
Transfer learning includes using knowledge from prior activities to efficiently learn new skills.
Now, to have more understanding, let’s explore some examples of Machine Learning.
Examples of Machine Learning
Let’s understand Machine Learning more clearly through real-life examples.
1. Image Recognition: Machine learning is applied in photographs and videos to recognize objects, people, landmarks, and other visuals. Google Photos uses ML to understand faces, locations and other elements in images so that they can be conveniently searched and categorized.
2. Natural Language Processing (NLP): NLP allows machines to interpret language as humans do. Automated customer service chatbots, for instance, use ML algorithms to reliably answer queries by understanding text and recognizing the purpose behind it.
3. Speech Recognition: ML is used to enable computers to understand speech patterns. This technology is used for voice recognition applications such as Amazon’s Alexa or Apple’s Siri.
4. Recommendation Engines: Machine learning algorithms identify patterns in data and make suggestions based on those patterns. Netflix, for example, applies machine learning algorithms to suggest movies or TV shows to viewers.
5. Self-Driving Cars: Machine learning is at the heart of self-driving cars. It is used for object detection and navigation, allowing cars to identify and navigate around obstacles in their surroundings.
Now, we hope that you get a clear understanding of Machine Learning. Now, it’s the perfect time to explore Artificial Intelligence(AI). So, without further ado, let’s dive into AI.
Understanding Artificial Intelligence (AI)
Artificial intelligence (AI) is a type of technology that attempts to replicate human intelligence’s capabilities such as issue-solving, making choices, and recognizing patterns. In anticipation of evolving circumstances and new knowledge, AI systems are designed to learn, reason, and self-correct.
The algorithms in AI systems use data sets to gain information, resolve issues, and come up with decision-making strategies. This information can come from a wide range of sources, including sensors, cameras, and user feedback.
AI has been around for several decades and has grown in sophistication over time. It is used in various industries, including banking, health care, manufacturing, retail, and even entertainment. AI is rapidly transforming the way businesses function and interact with customers, making it an indispensable tool for many businesses.
In the modern world, AI has become more commonplace than ever before. Businesses are turning to AI-powered technologies such as facial recognition, natural language processing (NLP), virtual assistants, and autonomous vehicles to automate processes and reduce costs.
Ultimately, AI has the potential to revolutionize many aspects of everyday life by providing people with more efficient and effective solutions. As AI continues to evolve, it promises to be an invaluable tool for companies looking to increase their competitive advantage.
We have many examples of AI associated with our daily lives. Let’s explore some of them:
Examples of AI
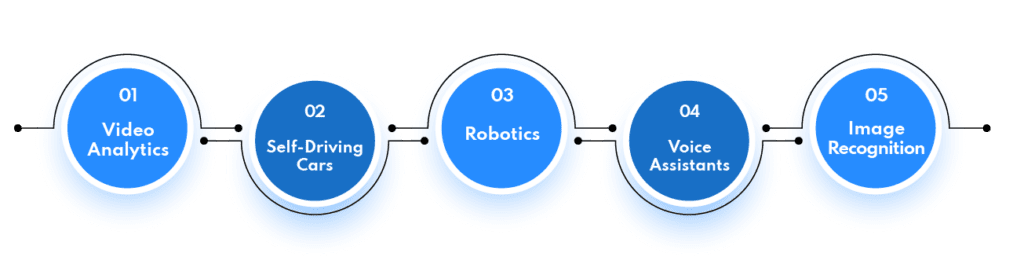
Some of the real-life use cases of Artificial Intelligence are:
1. Video Analytics: Video analytics is an AI application that analyses video footage and extracts valuable data from it using computer vision algorithms. It can be used to detect unusual behavior or recognize faces for security reasons.
This technology is highly used in airports and hotel check-ins to recognize passengers and guests respectively.
2. Self-Driving Cars: Self-driving cars are becoming more prevalent and are regarded as an important example of artificial intelligence. They use sensors, cameras, and machine learning algorithms to detect obstacles, plan routes, and change the speed of the vehicle based on external factors.
3. Robotics: Another important implementation of AI is robotics. Robots can use machine learning algorithms to learn how to perform various tasks such as assembling goods or exploring dangerous environments. They can also be designed to react to voice or physical instructions.
They are used at shopping malls to assist customers and in factories to help in day-to-day operations. Moreover, you can also hire AI developers to develop AI-driven robots for your businesses. Besides these, AI-powered robots are used in other industries too such as the Military, Healthcare, Tourism, and more.
4. Voice assistants: Artificial intelligence is utilized by virtual voice assistants such as Siri, Alexa, and Google Home to comprehend natural language commands and respond appropriately. Natural language processing (NLP) is used by these voice assistants to comprehend user commands and respond with pertinent information.
5. Image recognition: Image recognition is a type of artificial intelligence (AI) application that uses neural networks as a way to recognize objects in an image or video frame. It can be used in real-time to identify objects, emotions, and even gestures.
The examples of both AI and machine learning are quite similar and confusing. They both look similar at the first glance, but in reality, they are different.
In fact, machine learning is a subset of artificial intelligence. To explain this more clearly, we will differentiate between AI and machine learning.
Machine Learning VS Artificial Intelligence – The Key Differences!
Machine Learning (ML) and Artificial Intelligence (AI) are two concepts that are related but different. While both can be used to build powerful computing solutions, they have some important differences.
1. Approach:
One of the main differences between ML and AI is their approach. Machine Learning focuses on developing systems that can learn from data and make predictions about future outcomes. This requires algorithms that can process large amounts of data, identify patterns, and generate insights from them.
AI, on the other hand, involves creating systems that can think, reason, and make decisions on their own. In this sense, AI systems have the ability to “think” beyond the data they’re given and come up with solutions that are more creative and efficient than those derived from ML models.
2. Type Of Problems They Resolve:
Another difference between ML and AI is the types of problems they solve. ML models are typically used to solve predictive problems, such as predicting stock prices or detecting fraud.
AI, however, can be used to solve more complex problems such as natural language processing and computer vision tasks.
3. Computing Power Consumption:
Finally, ML models tend to require less computing power than AI algorithms do. This makes ML models more suitable for applications where power consumption is important, such as in mobile devices or IoT devices.
In easy words, Machine Learning and Artificial Intelligence are related but distinct fields. Both AI & ML can be used to create powerful computing solutions, but they have different approaches, and types of problems they solve, and require different levels of computing power.
ML & AI – Comparison Table
Point of Differentiation | Machine Learning | Artificial Intelligence |
Definition | Machine learning is a subset of artificial intelligence that enables computers to learn from data, identify patterns and make decisions with minimal human intervention | Artificial Intelligence is a term used to describe machines or software that are capable of mimicking or performing tasks usually associated with human cognition or behavior. |
Goal | The goal of Machine Learning is to enable machines to learn from data and improve their accuracy over time | The goal of Artificial Intelligence is to replicate human behavior and reasoning in order to solve problems and complete tasks. |
Process | ML algorithms use mathematical models and statistical analysis to extract meaning from data. | AI algorithms use problem-solving methods like search and planning to solve complex tasks. |
Implementation | ML algorithms are implemented using the programming language. | AI algorithms are implemented using Artificial Neural Networks and Deep Learning architectures. |
Outcome | The outcome of ML algorithms is usually a prediction or recommendation | The outcome of AI algorithms is usually an action or decision. |
Conclusion
Machine Learning and Artificial Intelligence are two distinct concepts that have different strengths and weaknesses. ML focuses on the development of algorithms and models to automate data-driven decisions.
On the other hand, AI emphasizes the development of self-learning machines that can interact with the environment to identify patterns, solve problems and make decisions.
Both are important for businesses, and it is important to understand the differences between the two in order to take advantage of their potential benefits. Therefore, it is the right time to get in touch with an AI application development company, make your business AI and Machine learning equipped, and enjoy the benefits of these technologies.