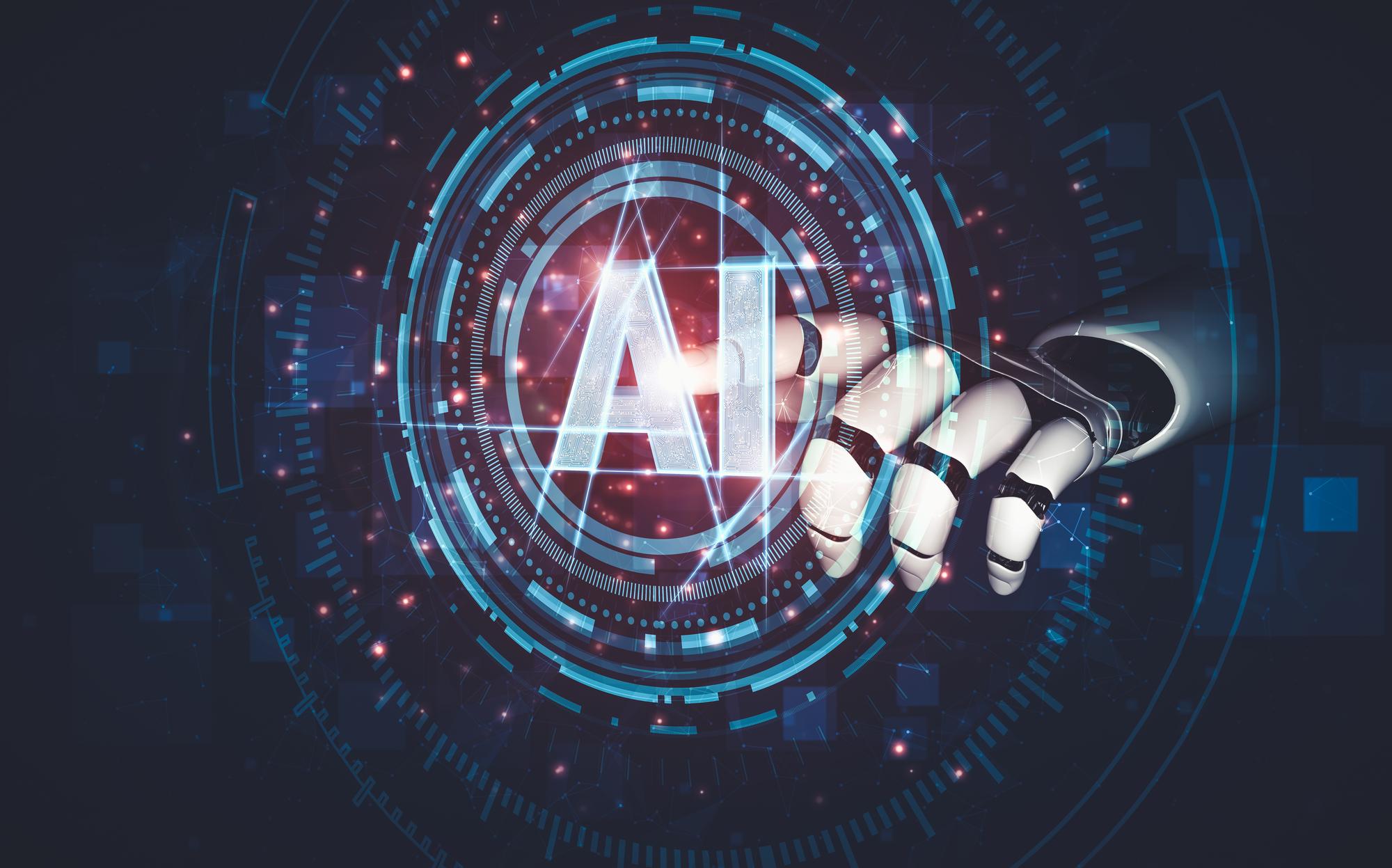
It’s not without good reason. In a recent study, IBM found that the average total cost of a data breach reached $4.35 million in 2022 globally and $9.44 million in the US. This underscores the need for more effective and proactive cybersecurity solutions that provide more advanced detection and response capabilities.
Innovative solutions for an evolving threat landscape
While traditional Security Information and Event Management (SIEM) systems have been a standard part of a business’ cybersecurity arsenal, cybercriminals are now growing in sophistication, developing attack methods that are increasingly capable of compromising systems. Thus, organizations will need to consider SIEM alternatives to stay ahead of the curve. AI and ML are emerging as powerful tools that address the limitations of traditional SIEM systems.
In particular, AI and ML provide innovative SIEM alternatives designed to protect businesses from growing cyber threats. This is particularly essential in providing decision-makers with valuable infosec and cybersecurity insights that will improve security posture. One key difference is how security is managed. Traditional SIEM systems are designed to manage and analyze security event data. This results in challenges keeping pace with how fast attack vectors evolve.
As organizations generate more data from a wider array of sources, however, SIEM systems will often encounter challenges in processing this information in real-time. This results in delayed threat detection and response.
In addition, such traditional systems rely on rule-based methods, which makes it a challenge to identify novel or unknown threats. More advanced alternatives to traditional SIEM systems ensure that cybersecurity defenses can effectively counter these modern threats.
AI and ML revolutionize how organizations approach cybersecurity by leveraging the power of data-driven algorithms and self-learning capabilities. These are able to detect and respond to threats more effectively, as well as learn and adapt to the ever-changing nature of cyberattacks.
For one, AI and ML can analyze vast amounts of data at high speeds. This enables real-time threat detection and response, which is particularly crucial as cybercriminals also start utilizing the same strategies and tools in executing their attacks. Rapid identification and mitigation of such attacks can significantly reduce the potential financial and reputational damages for businesses.
AI and ML can identify patterns and anomalies that may indicate previously unknown threats. Thai provides organizations an advantage in enhancing their security postures, thus staying one step ahead of cybercriminals and attackers.
How SIEM alternatives utilize AI in addressing threats
With the growing demand for alternative and intelligent cybersecurity solutions, such AI and ML-driven SIEM alternatives have now emerged, offering innovative approaches to fighting cyber threats. These go beyond traditional SIEM capabilities, as they incorporate technologies that enhance threat detection, response, and predictive analysis.
Some of these include:
Security Orchestration, Automation, and Response (SOAR): These platforms leverage AI and ML in automating repetitive tasks, streamlining incident response processes, and empowering organizations with more informed decision-making capabilities when faced with a cyberattack. Integration with other tools allows SOAR solutions to build a holistic security ecosystem that can adapt to new threats as they emerge.
User and Entity Behavior Analysis (UEBA): These solutions utilize AI and ML algorithms to monitor the behavior patterns of users and entities across an organization’s digital environment. UEBA identifies deviations from the norm and can therefore detect potential insider threats, compromised accounts, and other security risks. This adds an extra protective layer to a business’ cybersecurity defense.
Endpoint Detection and Response (EDR): EDR solutions focus on monitoring and collecting data from endpoints, which include IoT devices, smartphones, and BYOD devices, in order to identify potential threats. With AI and ML solutions, EDR can provide real-time analysis and thus respond to threats also in real-time. This lets businesses mitigate the risks associated with an expanding attack surface, in line with today’s increasing trend of utilizing BYOD and remote-work arrangements.
Some challenges ahead
While AI and ML-driven SIEM alternatives offer significant benefits, organizations must also consider the potential challenges and risks associated with implementing these technologies, and thus need to consider the following best practices:
Ensure data privacy and compliance: AI and ML solutions rely on large volumes of data to function effectively–thus, organizations must ensure they adhere to data privacy regulations and industry-specific compliance requirements.
Improve human resource capabilities in order to address the AI and ML skills gap: According to a recent study, only 10 percent of the global workforce has in-demand AI-related skills that will be useful in these changing times. As new technologies will also require highly-specialized skills and expertise, industries need to ensure their human resources can keep up.
Balance security, efficiency, and user experience: Maintaining a positive user experience is key to greater acceptance and adoption of any technology. As organizations adopt advanced cybersecurity solutions, they must strike a balance between improving security and maintaining operational efficiency.
The Takeaway
As AI and ML-driven SIEM alternatives continue to gain traction, it is crucial for decision-makers to recognize the potential of these technologies and prioritize their adoption within their organizations, especially those that focus on infosec and cybersecurity responsibilities. Key personnel needs to stay informed about the latest developments in AI and ML and understand the benefits they can bring in terms of enhancing cybersecurity strategies. Decision-makers can thus make more informed choices about the tools and solutions they implement to protect their businesses from cyber threats.
To ensure a successful transition to AI and ML-driven cybersecurity solutions, organizations must also invest in building a skilled workforce that understands these technologies and can effectively utilize them. This may include providing training and development opportunities, as well as collaborating with academic institutions and industry partners to address the AI and ML skills gap.
By carefully considering these challenges and working towards overcoming them, organizations can successfully harness the potential of AI and ML-driven SIEM alternatives to enhance their cybersecurity posture in an increasingly complex digital landscape.