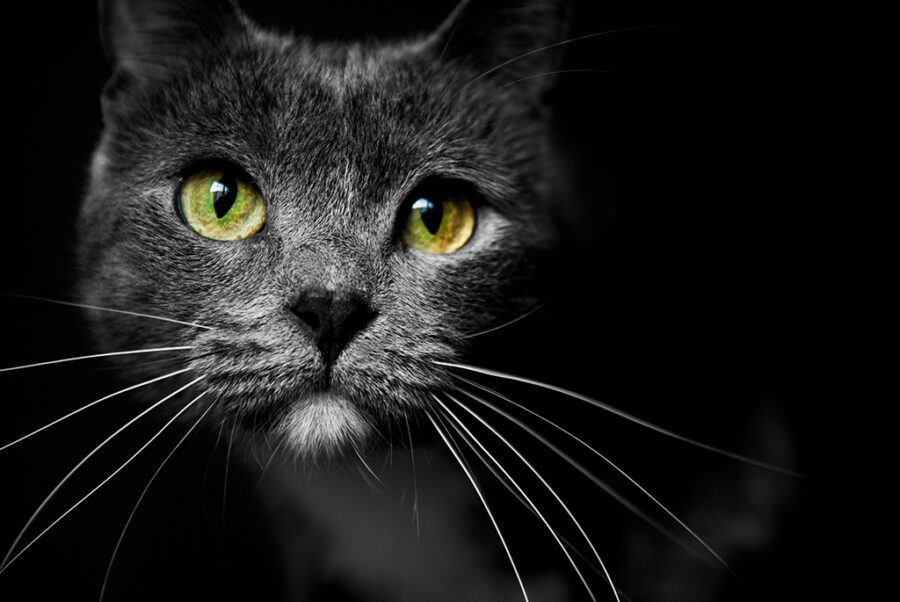
It is hard, looking at the current technological landscape, to believe that artificial intelligence may actually be facing a reckoning that could cause investment in the field to dry up. If you go by the press releases and even the many products that supposedly incorporate artificial-intelligence-oriented products, it would seem that computers capable of thinking rationally, of emulating humans in any way that mattered, was just around the next river bend.
Yet increasingly, the sentiment being expressed by those who work most with AI is that we may be heading for a wall. There are several solid reasons for this concern. Alan Morrison recently noted that what is more recently referred to as Artificial Intelligence can be divided into about five different buckets. The first, from within the realm of Data Science, is the use of stochastic (probability-based) algorithms used in conjunction with large data sets to perform what amounts to predictive analytics. There are a couple of offshoots of this: Bayesian analysis, in which predictions can be made based upon trees of prior predictions, expanded dramatically through the use of fast processors to explore decision trees consisting of millions of such branch points, and clustering analysis, which basically explores models with thousands, millions, or even billions of potential parameters to determine classifications.
Clustering eventually led to the creation of multilayer “neurons” consisting of graphs (a neuron, based upon ideas that have been around since the 1960s but that were only truly feasible recently) that would break apart data into signals and then attempt to find curves along a multi-dimensional surface in order to identify stable minima on that surface. This process involved utilizing a huge amount of data to train the models to recognize patterns that corresponded to known signals: pictures of cats or other objects, fragments of music, video sequences, and so forth. Since the system had to use data to build the model, this process became known as machine learning, though there is no evidence that this is in fact a reflection of how human beings learn things. There are different flavors of such learning, including unsupervised learning (where the datasets essentially attempted to identify and interpret the clustering of machine learning algorithms, a process otherwise known as labeling) and reinforcement learning, in which the model itself revises the initial model with new data over time.
Visual and auditory signal processing takes advantage of these algorithms coupled with others to convert the media into models. Many of these processes make use of non-linear differential equations (via various kernels, or neural networks) to create simulations, which can then be analyzed and pipelined. This has had great success in computerized vision and computerized speech, the former being seen in areas as diverse as Generative Adversarial Networks (GANs) that are able to produce digital portraits composited from existing photographs, image recognition, and even 3D model generation on the one hand, and systems capable of parsing and interpreting written and spoken text and image content on the other (as seen in areas as distinct as GPT-3 and Gato).
However, it is worth noting that all of these cases (even Gato, which is surprisingly versatile) still have to be constrained to work within very narrow domains of knowledge, and once outside of those domains, their accuracy or even meaningfulness drops dramatically. Generalization is proving remarkably elusive.
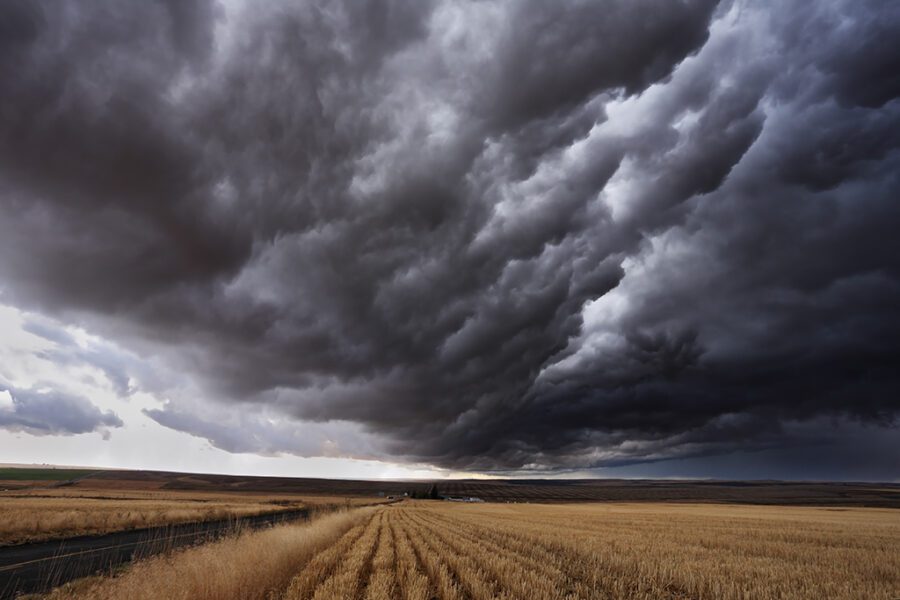
Part of the reason for that is that there is still a dramatic gulf between the algorithmic and inferential camp, though this is beginning to break down. The inferential camp uses graphs, but rather than using them as kernels, they use them to create multiple layers of abstraction through logical methods. One of the big goals of the machine learning camp is to prove that inferential analysis can emerge naturally out of training. My suspicion is that it may, but it is too fragile and subtle to survive analysis. As a very simple example, this is somewhat analogous to calculating an average and standard deviation for a data set, but at the expense of losing information about outliers and similar patterns. There are interesting glimmers where people are exploring graph embeddings and similar analyses, but the distance between the two camps remains daunting.
Ultimately, the ability to deal with multiple levels of abstractions may prove key to making that elusive jump to generalizations — showing that human intelligence is not one process, but several that complement one another. Unification of inferential thinking (and knowledge graphs) with Bayesians seems a likely next step, and already you are seeing machine learning serve as an automated classifier for knowledge graph systems based upon data shapes. Deep learning, which is increasingly dealing with iterated fractal systems (IFS) may also represent a significant next step, as at a certain level knowledge graphs begin to display fractal characteristics that may be crucial to explainability.
The history of computer cognition goes in waves, as sometimes seemingly competing approaches actually mask underlying symmetries that only emerge when you have enough computational power to see them. So, it may very well be that the next real wave of innovation will happen only once we have enough GPU cycles to see them, like some kind of virtual particle accelerator. Until then, we might at least face the cold winds and driving rain of an AI Autumn.
Kurt Cagle
Community Editor,
Data Science Central
To subscribe to the DSC Newsletter, go to Data Science Central and become a member today. It’s free!
Data Science Central Editorial Calendar
DSC is looking for editorial content specifically in these areas for June 2022, with these topics having higher priority than other incoming articles. If you are interested in tackling one or more of these topics, we have budget for dedicated articles. Please contact Kurt Cagle for details.
- GATO and AGI
- NERFs
- Digital Currencies 2022
- Labeled Property Graphs
- Telescopes and Rovers
- Graph as a Service
- DataOps
- Simulations
- RTO vs WFH
DSC Featured Articles
- Top Ways in Which Data Science Improves E-Commerce Sales Ryan Williamson on 24 May 2022
- Why is the Gig Economy a New Future? Rumzz Bajwa on 24 May 2022
- Lessons Learned from Writing My First Python Script Vincent Granville on 24 May 2022
- Russian Troll Detection By Their Tweets myabakhova on 24 May 2022
- Importance of Fearlessness to Exploit the Potential of AI Bill Schmarzo on 24 May 2022
- Why Should the Retail Sector Move to Cloud Computing? Ryan Williamson on 23 May 2022
- Keeping Your Company’s Data Model IP Alan Morrison on 21 May 2022
- 5 Ways to Optimize Database Performance Aileen Scott on 20 May 2022
- The Foundation of Data Fabrics and AI: Semantic Knowledge Graphs Jans Aasman on 19 May 2022
- Part 2: Is Data Mesh Fool’s Gold? Not if You Avoid the Traps Bill Schmarzo on 19 May 2022
- Artificial Intelligence and the Future of Medical Imaging Nikita Godse on 19 May 2022
- How to Use the Resources of MQL5.community to Empower Your Own Business Rumzz Bajwa on 19 May 2022
- Common Sense Machine Learning Vincent Granville on 17 May 2022
- Artificial Intelligence in Social Media Enriches Marketing ROI Nikita Godse on 15 May 2022
- Why Gato from Deepmind is a Game Changer ajitjaokar on 15 May 2022
- Social Media, Cyber Bullying, and Need For Content Moderation Roger Brown on 11 May 2022
- How AI Is reshaping Manufacturing Nikita Godse on 11 May 2022
- Is Data Mesh Fool’s Gold? Creating a Business-centric Data Strategy Bill Schmarzo on 10 May 2022
- Top Technological HR Skills for the HR Professional Aileen Scott on 10 May 2022
- Should the definition of Digital twins include simulation of complex systems? ajitjaokar on 09 May 2022
- Network security guidelines for 2022 Karen Anthony on 08 May 2022
- Understanding EXIF Data and How to View It on Android Phones Karen Anthony on 08 May 2022
- Comparing Cloud Telephony With On-Premise Call Centers: An Upgrade Aileen Scott on 08 May 2022
- The long game: Desiloed systems and feedback loops by design (I of II) jkannoth on 08 May 2022
- DSC Weekly Newsletter 10 May 2022: Data Meshes, Digital Twins, and Knowledge Graphs Kurt Cagle on 11 May 2022
Picture of the Week
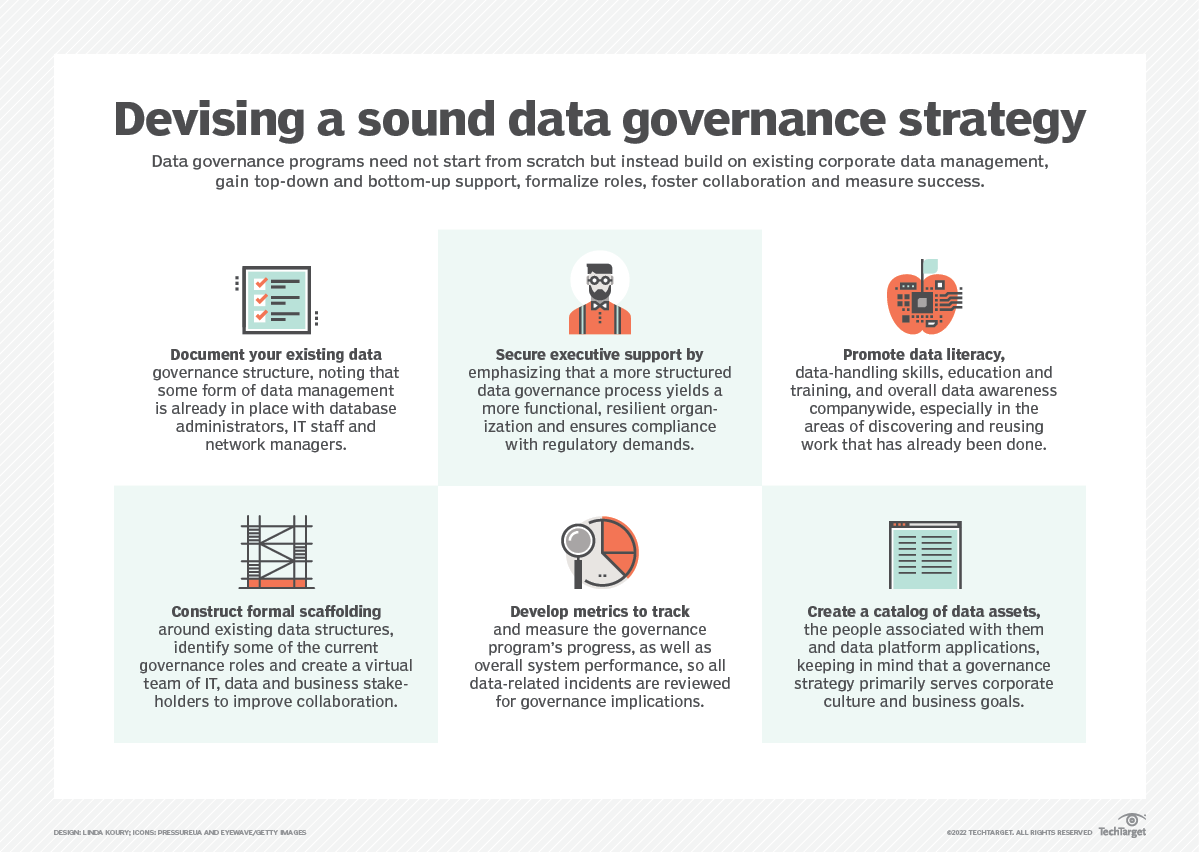