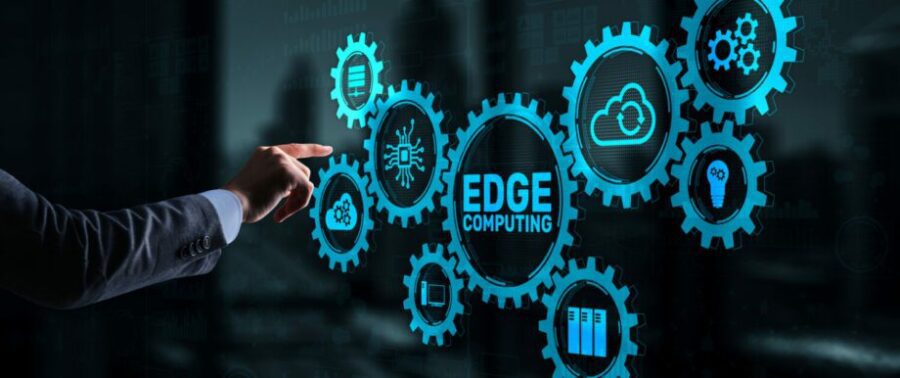
In today’s digital landscape, where data is often hailed as the new oil, the rise of edge computing stands as a transformative force reshaping the way we manage and utilize data. Edge computing marks a significant shift from the traditional centralized data processing model to a decentralized approach, bringing computation and data storage closer to the source of data generation. As this technology gains momentum, exploring how it will impact data management strategies is imperative.
Understanding edge computing
In simple terms, edge computing involves processing data near the network’s edge, where it is generated, instead of relying on a centralized data processing warehouse or cloud. This approach significantly reduces latency, enhances real-time processing capabilities, and alleviates bandwidth constraints. It is ideal for applications requiring rapid response times, such as IoT devices, autonomous vehicles, and smart sensors.
Some key features of edge computing are as follows:
1. Proximity to data generation: Edge computing consists of deploying computing resources, such as servers, storage, and networking equipment, closer to where data is generated.
2. Decentralized architecture: Edge computing follows a decentralized architecture, unlike traditional cloud computing. Edge devices, including routers, gateways, and IoT devices, perform computation and data storage tasks locally without relying heavily on connectivity to a centralized server.
3. Scalability and flexibility: Edge computing offers greater scalability and flexibility than traditional cloud computing models. Organizations can quickly scale their edge infrastructure by adding or removing edge devices as needed.
4. Edge intelligence and analytics: Edge computing empowers edge devices to perform intelligent processing and analytics tasks locally without constant communication with a centralized server.
5. Hybrid edge-cloud architectures: While edge computing offers many benefits, it does not entirely replace the need for centralized cloud infrastructure. Instead, organizations increasingly adopt hybrid edge-cloud architectures, combining edge computing and cloud computing strengths.
6. Security and privacy considerations: Edge computing introduces unique security and privacy challenges that organizations must address. These challenges involve the integrity and confidentiality of their data. Because edge devices are often deployed in physically vulnerable or uncontrolled environments, they are more susceptible to physical tampering, unauthorized access, or cybersecurity threats.
Let’s look at how these features affect data management strategies.
Impact on data management
1. Reduced latency and improved responsiveness
Edge computing brings computing resources closer to end-users and devices, minimizing the distance data needs to travel. As a result, latency is drastically reduced, enabling faster response times for critical applications. Data integration tools must adapt to this shift by prioritizing real-time analytics and decision-making capabilities at the edge, allowing organizations to capitalize on time-sensitive insights without depending solely on centralized data processing.
2. Scalability and flexibility
Edge computing empowers organizations to scale their infrastructure dynamically, distributing computational workloads across edge devices. This scalability and flexibility necessitate agile data management frameworks seamlessly integrating edge data with centralized repositories. Combining edge and cloud resources, hybrid data management approaches will become increasingly prevalent, enabling organizations to effectively balance performance, cost, and data governance requirements.
3. Data governance and compliance
With data being processed and stored across a distributed edge infrastructure, ensuring compliance with regulatory requirements and maintaining data governance becomes more complex. Data management strategies must encompass robust security measures to safeguard sensitive information at the edge. Additionally, organizations must implement comprehensive data lineage tracking mechanisms to maintain data integrity and traceability across the entire edge-to-cloud continuum.
4. Edge-to-cloud data orchestration
Effective data management in an edge computing environment necessitates seamless orchestration of data movement between edge devices and centralized cloud repositories. This entails developing sophisticated data synchronization mechanisms, edge caching strategies, and data replication protocols to ensure consistency and coherence across distributed data stores. Automated data lifecycle management solutions will be crucial in optimizing data placement, retention, and archival processes across the edge-cloud spectrum.
5. Edge-native data processing and analytics
Edge computing unlocks new possibilities for performing data processing and analytics directly at the edge, minimizing reliance on centralized cloud resources for insights generation. Data management frameworks must embrace edge-native processing technologies, including lightweight machine learning models, edge databases, and stream processing engines, to leverage the full potential of edge-generated data. Organizations can achieve greater operational efficiency and innovation agility by empowering edge devices to analyze and act on data autonomously.
Edge computing in action: Smart traffic management system
In a traditional centralized data processing model, an intelligent traffic management system might rely on sending data from numerous sensors deployed across the city to a centralized cloud server for analysis. This process involves transmitting large volumes of data over the network, leading to latency issues and delayed responses to traffic incidents or congestion.
However, edge computing brings processing power closer to the source of data generation, such as traffic cameras, sensors embedded in roads, and traffic lights. In this scenario:
- Real-time data processing: Edge devices installed at intersections analyze streaming video feeds from traffic cameras and data from sensors in real-time. This immediate analysis allows the system to detect anomalies, such as accidents or congestion, as they occur.
- Localized decision-making: Edge devices can autonomously make localized decisions based on the data they collect. For instance, a traffic light with edge computing can adjust its signal timing dynamically. This optimizes traffic flow in response to changing conditions.
- Reduced latency: By processing data locally at the edge. The system significantly reduces the time it takes to detect and respond to traffic events. This low-latency approach improves overall responsiveness, leading to smoother traffic flow and reduced congestion.
- Bandwidth optimization: Edge computing minimizes the need to transmit large volumes of raw data to a centralized server for processing. Instead, only relevant insights or aggregated data are sent to the cloud for further analysis. This optimizes bandwidth usage and reduces network congestion.
Consequently, edge computing revolutionizes intelligent traffic management by allowing real-time data processing and local decision-making at the network edge. By leveraging the proximity of edge devices to the data source, the system achieves lower latency and improved responsiveness. This results in more efficient use of network resources, ultimately enhancing smart city infrastructure effectiveness.
Conclusion
The rise of edge computing heralds a transformative era for data management. It challenges organizations to rethink their data processing, storage, and governance approaches by embracing the decentralized nature of edge computing and adopting agile data management strategies. Organizations can harness the full potential of edge-generated data to improve their data management strategies in the digital economy.